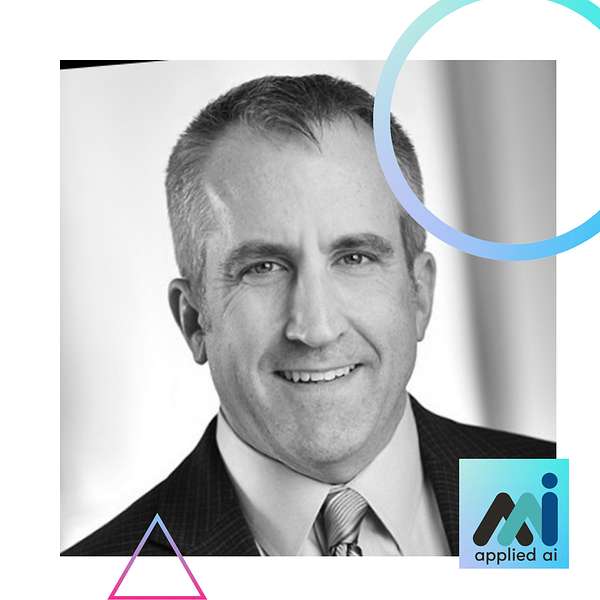
Conversations on Applied AI
Welcome to the Conversations on Applied AI Podcast where Justin Grammens and the team at Emerging Technologies North talk with experts in the fields of Artificial Intelligence and Deep Learning. In each episode, we cut through the hype and dive into how these technologies are being applied to real-world problems today. We hope that you find this episode educational and applicable to your industry and connect with us to learn more about our organization at AppliedAI.MN. Enjoy!
Conversations on Applied AI
Michael Petersen - Artificial Intelligence and Repairing Your Automobile
The conversation this week is with Michael Petersen. With over 30 years of engineering, marketing, and leadership experience, he is the founder and CEO of Raise a Hood Inc., a startup that aims to democratize the automotive maintenance and repair sector through AI, crowdsourcing, and community platforms.
He enjoys building outstanding specialized teams that are committed to a shared vision and a culture of excellence, collaboration, and diversity. As a passionate automotive enthusiast, he spends his free time building engines. Practicing vehicle dynamics and riding adventure bikes. He also serves as a board member and CMO of Sunset Hospice Cottage, a nonprofit organization that supports patients and families in their end-of-life journeys.
If you are interested in learning about how AI is being applied across multiple industries, be sure to join us at a future AppliedAI Monthly meetup and help support us so we can make future Emerging Technologies North non-profit events!
Resources and Topics Mentioned in this Episode
[00:00:00] Michael Petersen: The problem as a whole in terms of diagnosing somebody's vehicle, just based on symptoms and based on the conversation, is incredibly complex because there's so many makes, models, model years, powertrain configurations, options, etc., etc., and then on each vehicle there's still three to four thousand parts that could potentially go wrong.
[00:00:20] That this whole model just blows up to a level that you can't mathematically tackle it. And so this is where AI and these huge databases come into play where you can actually do something with this data. Because if it wasn't for AI, there's just, there's not enough computational horsepower to even begin to look at these elements.
[00:00:40] AI Announcer: Welcome to the Conversations on Applied AI podcast where Justin Grammens and the team at Emerging Technologies North talk with experts in the fields of artificial intelligence and deep learning. In each episode, we cut through the hype and dive into how these technologies are being applied to real world problems today.
[00:00:58] We hope that you find [00:01:00] this episode educational and applicable to your industry and connect with us to learn more about our organization at AppliedAI. mn. Enjoy!
[00:01:11] Justin Grammens: Welcome everyone to the Conversations on Applied AI podcast. Today, we're talking with Michael Peterson. With over 30 years of engineering, marketing, and leadership experience, he is the founder and CEO of Raisa Hood Inc.,
[00:01:21] a startup that aims to democratize the automotive maintenance and repair sector through AI, crowdsourcing, and community platforms. He enjoys building outstanding specialized teams that are committed to a shared vision and a culture of excellence, collaboration, and diversity. As a passionate automotive enthusiast, he spends his free time building engines.
[00:01:40] Practicing vehicle dynamics and riding adventure bikes, which sounds super interesting, Mike, and love to learn more about that through our conversation. He also serves as a board member and CMO of Sunset Hospice Cottage, a nonprofit organization that supports patients and families in their end of life journeys.
[00:01:55] Thank you, Michael, for being on the program today. You
[00:01:57] Michael Petersen: know, Justin, nobody's ever actually read my [00:02:00] intro like that in its entirety and I'm thinking to myself, what person rides adventure bikes and then sits on the board of a hospice cottage? Like, what's the common denominator between the two of those?
[00:02:09] Justin Grammens: Yes, well, hopefully through this conversation we'll get to that answer, right?
[00:02:13] Michael Petersen: Exactly. Exactly. Listen, thanks for having me on your broadcast this morning. I really appreciate it. Yeah.
[00:02:18] Justin Grammens: Anytime. Anytime. Yeah. I mean, I told a little bit about what you're doing today. Maybe you could give a short background of sort of the path of your career and the trajectory of sort of how you got
[00:02:27] Michael Petersen: here.
[00:02:28] Yeah. No, I would love to. And whenever you read somebody's bio, they're always a little bit dry and professional, but there's always a much richer story behind all of that. The fact of the matter is I was born here in the Minnesota area and I grew up in a small town and my father was a mechanic for many years.
[00:02:43] Those seeds that he planted in the automotive space certainly took root and maybe grew a little bit deeper and a little further than he had expected. And so that led me to engineering school and sent me off to Detroit with Ford Motor Company for a long time. After B school though, [00:03:00] I realized that the automotive sector, it was my opinion back then, the automotive sector, Was not moving very rapidly and it was very insular and it just felt a little bit confining.
[00:03:10] And we can talk about all the pros and cons of the automotive sector in detail, but, but the fact of the matter is, I just, I realized there's a, a better chapter out there for me. So I went into high tech, so I went to B School at Michigan and uh, ended up at Dell immediately thereafter and did a number of very interesting things, I think in the high tech sector.
[00:03:28] And so I had this unique blend of tech and automotive and I, but I never really left the automotive space. I still. I raced occasionally and I was teaching track school and all of it was just to kind of fill up this hobby. And so I had this interesting perspective of sort of the world through the eyes of a mechanic, my father, the world of automotive in a very technical, detailed perspective from my years of experience, and then just all the amazing stuff that was going on in high tech and how rapidly [00:04:00] things could change and should change.
[00:04:02] in, in the world around us and, you know, for just, it's amazing to me from the time I started B School, which was many years ago, to today, when I started B School, the internet was just starting to become a thing and, and now, I mean, we just take that stuff for granted like it's, like it's electricity. And so I ended up at this point in my career where I.
[00:04:22] You know, I didn't have to worry about money much anymore and I had kind of accomplished the things professionally that I wanted to, but I realized in the automotive space that it just still was not moving very quickly for the vast number of Americans. That actually relied upon their vehicle. And we get so focused on the stuff around the corner and it's great.
[00:04:45] We have driverless vehicles and, and AI coming into vehicles and battery vehicles and battery electric hybrid vehicles and all sorts of really fascinating, cool stuff, but. Those really interesting [00:05:00] technologies like BEVs, Battery Electric Vehicles, for instance, constitute about a million units per year being sold into the, into this population of 300 million vehicles.
[00:05:11] And so while we all focus on what's around the corner in two years and five years and ten years, the average American, just the idea of going out to buy a Tesla or electric whatever it may be, is so far off their radar that it's not even a consideration set. The average person in North America drives a 12 to 13 year old car.
[00:05:29] The average person. Battery vehicles is not anywhere on their roadmap. They're just trying to figure out how to get to work on Tuesday. And when their car breaks down, this is kind of a traumatic event because the majority of people don't even have the cash reserves to fix a vehicle when it's broken.
[00:05:46] The dynamics within the repair industry, and by the way, we spend 150 billion dollars per year taking care of our cars when they break down and for maintenance. It's a big number. But quite frankly, the wealthy, you know, quite [00:06:00] frankly, you and I probably qualify as that category. If you're driving a new vehicle, you don't even see it because it's all under maintenance and by the time your warranty runs out, you...
[00:06:08] Sell your car and you move on. But the average American isn't like that. And so the question we asked was that, is there a way that we can blend together what we know about vehicles, what we know about engineering, the amazing advancements that are happening in artificial intelligence, and using that to try to address the needs of the average American and try to disrupt.
[00:06:29] this service and maintenance sector for the better. You know, the thing that I think is wonderful about high tech is that you can take an existing ecosystem and disrupt it, but what comes out the other end helps a much wider swath of people. I think a best example of that is Uber. Think about when you and I were young, and I think you and I are of a similar generation.
[00:06:52] When we were young, if you had to take a vehicle to the airport, if you didn't rent a car, you would take a taxi. And you despised taking a [00:07:00] taxi because they were notoriously late, they were overpriced, the cars felt like they were 42 years old, I mean, there was nothing efficient about this system. Yeah.
[00:07:07] Right? And Uber comes along and lift and ride. They were so good that they effectively wiped out the taxi industry to the point now where people say, well, I don't want to drive my own car. I want to take a Lyft because there's all these advantages to taking a ride share service. To me, that's when you can disrupt something and make it fundamentally better for everybody.
[00:07:26] When you look at the world of automotive maintenance and repair. is we have some of those same dynamics. Think about the average mechanic and, and he, and I say he a lot because 98 to 99 percent of the mechanics are he's. We actually have one female mechanic on our team which we're super excited about.
[00:07:45] Her name is Lori Johnson, but regardless, the majority of mechanics are he and, and that average person is making 40 an hour. They're charging out their time at 160 to 180 an hour. You know, there's effectively a [00:08:00] 5X markup and that mechanic doesn't fundamentally even have a chance to talk to customers.
[00:08:05] That mechanic is kind of locked back in the shop doing the heavy lifting, getting the greasy fingers, and they're not leveraging them for their expertise. They have a service manager out there who's trying to do the sale and drive better revenue and drive for their, you know, service on a person's vehicle because they have a P& L to run.
[00:08:23] So the question is, how do you take the people that are the brightest that know about your particular vehicle problem? And get them as close to the customer who really wants that information so that you can reduce the overall cost and start to disintermediate this entire ecosystem. And so while that sounds very aspirational, and it is, is there's absolutely a better way.
[00:08:44] And so our objective is how can we take some of this technology and try to do some of that early disruption.
[00:08:50] Justin Grammens: Maybe walk us through how you're then applying artificial intelligence to this massive problem that you saw on the market and continue to see
[00:08:57] Michael Petersen: in the market. I will say this, that [00:09:00] something interesting happened in 2020.
[00:09:03] First of all, we all know it was kind of the year of COVID, COVID disrupted a lot of things. There were two fundamental changes in the marketplace in around 2020, 2021 that really made this business viable. The first one is when COVID happened. Almost everybody became familiar with Zoom. Now, you and I had probably been using Zoom long before COVID, just that's the way we function in the tech space.
[00:09:28] But the average American did not. And all of a sudden, people became comfortable and aware of having video based conversations, right? So now you can effectively communicate with somebody just about anywhere in the world in a very meaningful way, right? With audio and video. The second though, and this is right in your wheelhouse, Justin, is that AI really started to take hold and it came to the point where you could build AI in a means that was much more affordable and targeted than it [00:10:00] ever had been in the last, you know, since its inception many, many years ago in the seventies, I guess.
[00:10:05] And to the point where now you can start to use AI on a more appropriate capital intensive scale to address some of these issues, right? Because it becomes a little bit of a, you know, a building block exercise. For the business that we're trying to build, first of all, we're trying to get mechanics close to customers.
[00:10:21] You need Zoom for that or Zoom like communications for that. The second, though, is to build an AI engine around this that makes sense. is the problem as a whole in terms of diagnosing somebody's vehicle just based on symptoms and based on the conversation is incredibly complex because there's so many makes, models, model years, powertrain configurations, options, et cetera, et cetera.
[00:10:47] And then on each vehicle, there's still three to 4, 000 parts that could potentially go wrong, that this whole model just blows up to a level that you can't mathematically tackle it. And so this is where AI [00:11:00] and these huge databases come into play, where you can actually do something with this data.
[00:11:04] Because if it wasn't for AI, there's just, there's not enough computational horsepower to even begin to look at these elements. And so it was really the culmination of kind of post COVID that all of a sudden we went, boy, this, this is actually viable now, where if we said, let's go try to stand this business up five years ago, I just don't think it would have been possible.
[00:11:22] Justin Grammens: Yeah. And there's a number of reasons as to why, I mean, was the data not readily available back then? Because that's the other key component. It's not only, you know, the cost of, of compute has gone down and storage has gone down and All that sort of stuff, but do you think the data sets have been easier to get?
[00:11:37] Uh, no.
[00:11:38] Michael Petersen: No, okay.
[00:11:40] Justin Grammens: It
[00:11:41] Michael Petersen: remains to be a problem. Well, and let me rephrase that is, I don't even know what kind of car you drive, Jason. I have no idea. I think you drive an electric, don't you? No.
[00:11:50] Justin Grammens: Interesting going, going back. I love that you went through automotive engineering because that's really what I was going to study in college.
[00:11:55] Actually, I have a lot of family from Detroit. And as I was [00:12:00] growing up, you know, and they all worked for, you know, the big three. And, and so I always sort of thought about going into automotive engineering and I did actually start a program going through mechanical engineering and then working into automotive because I mean, I just would love to design the next engine, but computers pulled me into that.
[00:12:15] Right. So I got into software development and like programming. But, you know, that's a roundabout way of me saying like, I, I love the path that you took because I, part of my heart is like, I actually would have loved to go that direction. I am one of the people that you said drives an old car. So I have a 2005 Mazda 6.
[00:12:34] It's a hand me down. I always buy a new car and I just drive it until the end of its life. And I even get the even hand me down from my wife, right? So she always has the newer car, but I'm absolutely going to be getting an EV next. But right now my car continues to function and I'm lucky enough to only have to drive a couple miles a day.
[00:12:52] My office is pretty close to my home and I don't really have to go, you know, a lot. But I sympathize with whenever there's a problem that happens, [00:13:00] how do I get it fixed? And who do I talk to? Again, I'm mechanically inclined. I don't have time, and in a lot of ways my time would be better spent, I think, in front of a keyboard and, uh, thinking through how we apply artificial intelligence than it would be to changing spark plugs, for example.
[00:13:16] So that's the landscape of me personally. I think I fit in that category of like this person, I'm out front in the tech space, but when it comes to vehicles, I guess I'm pretty practical and I just kind of continue to ride what I got. So
[00:13:27] Michael Petersen: you, you have a, a better appreciation of what we're trying to accomplish.
[00:13:32] When you have your, your Mazda 6 repaired, Justin, what do you do with the data?
[00:13:37] Justin Grammens: Data, like what was replaced and who replaced it and what the costs were and all that sort of stuff. It is literally sitting on a receipt in a bunch of receipts that are probably sitting there in my car right now.
[00:13:46] Michael Petersen: Correct. I mean, that's what every American does.
[00:13:49] If you're really maniacal about it, you might have a folder in your desk. That says Mazda 6 and you might stuff it in there. Yep. And then after you sell the car, you probably throw that [00:14:00] all away anyway because people are always concerned about sharing personal information. So the point I was making is, there's nothing to do with the data.
[00:14:07] There's no place to report it. Yeah. If you go to a dealership, that information is absolutely recorded. Yeah. And all of it goes, if it, from Mazda, Mazda consumes all that information. They primarily use it for warranty control costs. And then after the fact, if they have an excursion, they'll look at things and say, do we have enough parts to go cover, you know, this part that's failing at 120, 000 miles, but there's no incentive for them to share that information.
[00:14:33] In fact, I would argue it's on the other spectrum, which is there is so much litigious risk for them sharing that information because imagine them sharing all this data and you could go back and say, hey, in almost 1 percent of the cases, this fuel pump fails. At 100, 000 miles, you could leave somebody stranded on the side of the road in the middle of a snowstorm.
[00:14:54] You know, you, this is your responsibility, Mr. Manufacturer. And so there's [00:15:00] huge litigious risk across all of this. And so they, they need to protect that data for obvious reasons. So part of ours is how do we acquire that data? And we can talk about the data acquisition strategy, which is a, you know, a whole separate stream.
[00:15:12] But the fact of the matter is. We pull it from different modes, including directly from the consumer, but that's where these kind of these knowledge graphs and other elements start to become important so that we can take elements of data that's a little bit disparate and start to stitch them together in a way that's meaningful and applicable to an AI environment.
[00:15:32] Sure. So we're changing behavior. We have to change some behavior out there to get the necessary data. It also means with the partner shops that we have, we do extract data from partner shops. So even if it's an independent mom and pop shop, when you get your car repaired and they print the receipt out, which they give you, it says, here's my VIN, here's my mileage, here's what the customer complaint was, and here's what was done.
[00:15:55] We can silently consume all of that through our partner shop. We strip [00:16:00] out any personal information. We don't care about that, but we know make, model, year. And now we can get this silent feed coming in from the hundreds and thousands and hundreds of thousands of different shops around the country to do exactly that, which is to actually collect that data.
[00:16:15] Yeah, phenomenal.
[00:16:16] Justin Grammens: You guys are then trying to build, you know, in some ways, I guess, like an expert system, but based on all this data, create the best mechanic experience, I guess, for the end consumer. Like, but
[00:16:26] Michael Petersen: what's the... Well, what's the end goal on this? Yeah. You'll appreciate this being a little bit of a car guy.
[00:16:31] Is if you go online on your Mazda 6 and you say, I'm having trouble with whatever it may be. You will find a mountain of opinions out there online and it's certainly no shortage of conversation and it is typically amateurs talking to amateurs. And once they have it fixed, rarely does somebody come back and actually report what their problem, what result.
[00:16:54] They're just, everybody's looking for the answer but they don't say, they don't pay it forward. And so if you [00:17:00] went in with your car and your Mazda 6 and maybe you had some tick in the engine. is, you may spend a half of a day doing research online, you bring it to the shop and you'll say, Hey, Mr. Mechanic, I've been Googling this and YouTubing this, I think I have this figured out.
[00:17:15] I'm almost positive the problem is such and such. What percentage, because people do this all the time, what percentage of the time will mechanics tell you that you got it right? Now, they'll never tell you to your face because they want to keep you as a customer, but behind your back, what percentage of time does a customer get it right?
[00:17:32] It's
[00:17:33] Justin Grammens: pretty low and you know, it's funny when you were saying that, I actually was thinking it seems similar to a going into a doctor for a diagnosis as well, right? Very
[00:17:41] Michael Petersen: similar. Very similar. The good news is there is certainly a lot of litigious risk about making predictions about somebody's ailment versus making somebody's prediction about their water pump.
[00:17:53] But when somebody comes to the mechanic and they say, Hey, I've been Googling this. I got it figured out, man. Can you change my such and such? The mechanic will [00:18:00] typically say, Hey, you're probably right, Justin. We're going to check that out just to make sure, but we'll get you straight taken care of. The customer gets it right about 15 percent of the time.
[00:18:09] So you have Google with, you know, terabytes and terabytes of data all over the world, and people get it right one time out of, you know, 10. If you reverse that though, and you go to an actual Mazda mechanic who has a lot of expertise in your Mazda six. And you say, Hey, David, my car is doing such and such.
[00:18:29] The mechanic will start asking you questions. Well, when did this start? How many miles do you have on the car? Does this happen at startup or what makes seems to make it worse or, but they start asking you very specific questions about when you actually started experiencing a problem, when you experienced that problem, what you can do to exacerbate the problem because they're trying to diagnose it.
[00:18:51] When the mechanic quizzes the individual, and even if they've never had a chance to lay eyes on the vehicle, that mechanic [00:19:00] will get it right not 15 percent of the time, they'll get it right 85%. Interesting. And so, the, the idea with our AI engine, and by the way, our AI engine is branded GUS, which stands for generative uniform syntactics, but it just happens to also sound like a mechanic and it's easy to spell and easy to remember, so.
[00:19:19] So anyway, GUS, our AI engine. Is the idea is we start with an expert system, just like you said. And so we interview all these mechanics and we effectively take these expert systems and consume that into the AI engine. And then what is unique, we actually have patent pending in this area about how we effectively put the AI engine on appropriate guardrails so that we can blend together the best of the human intelligence and the best of the big data.
[00:19:46] So that the intention is Gus is as at least as smart as the best mechanic. And then it only gets better from there because the downside from a mechanic, if you were a mechanic and you had spent [00:20:00] the last 30 years working on Mazdas and they were working on Mazda 3s and Mazda 6s and Mazda CX 9s and Mazda Miatas and Mada, you know, RX 7s and RX 8s and all sorts of cool Mazda stuff, right?
[00:20:13] How many Mazda 6s? Is, have they seen with your particular issue, 10, 15, you know, really not a very large number. Sure. Yeah. And so that's where this gets exciting, is now you're blending together a mechanic, often can't give you good, accurate diagnostics of. Probabilities, because they just don't see enough cars to make a statistically accurate recommendation.
[00:20:39] Justin Grammens: One human can only see so
[00:20:40] Michael Petersen: much. That's exactly right. So they have been trained, so most mechanics have been trained, that if you bring your car in for service, if there's a risk something is going to fail, they don't want you coming back because they see that as a negative. So they tend to be very conservative and replacing many parts even long before it's necessary just because they go, well, [00:21:00] I know this is a.
[00:21:01] Typical failure point or it could, it could represent risk, so let's just mitigate all risk. It's a little bit like a lawyer. If you ask a lawyer, should I do this and there's risk associated with it, he or she will say, no, don't, don't do that.
[00:21:14] Justin Grammens: That's their job is to mitigate your risk. That's their job.
[00:21:17] Michael Petersen: That's the idea around Gus is we're trying to blend together the best of human intelligence plus the best of more traditional ML or AI intelligence. into something that's truly unique and differentiated. Nice.
[00:21:30] Justin Grammens: That's fascinating. And you guys have been kind of on a little uptick here, right? You guys are hiring, I think.
[00:21:35] And in fact, you guys were just up for a Techni Award, weren't
[00:21:38] Michael Petersen: you? We've been pushing hard on this and we're, we're really excited about the business and others share some of that enthusiasm. We won the, the Minnesota cup this year, the tech division for the Minnesota cup this year. And so we were really excited about that.
[00:21:50] We were up for the Techni Awards in the category of advancements in artificial intelligence. The fact of the matter, we were up against Target and Medtronic. [00:22:00] I'm pretty sure that their budgets are slightly larger than ours and their teams are slightly larger than ours. That's good company to be in. It's great company to be in.
[00:22:08] Yeah. Although Medtronic won for, ironically, Medtronic won for improved diagnostics of heart palpitations, right? And the last I checked, the heart is just a pump. And so Medtronic won for really servicing one pump. And we serve fuel pumps, and we serve water pumps, and we have air pumps, and I mean, we have multiple pumps.
[00:22:30] I'm quite surprised that we didn't actually win that award. We'll get over it. Yeah,
[00:22:36] Justin Grammens: much harder. Yeah, you guys solved a much harder problem. Well, that's, that's, that's fabulous. That's fabulous for you guys. I guess this one question I do like to ask people who are on it, who are kind of like running businesses around AI, what's, what's a day in the life for, for, for you as the CEO of this business and focusing on AI?
[00:22:52] Michael Petersen: Somebody said this wisely once, they said, you're going to work 80 to a hundred hours a week building a business. And then the [00:23:00] other half of that you're going to spend towards fundraising. You know, there's just, there's just not enough hours in the, in the day. And so we're going through what is, you would refer to as a seed round.
[00:23:10] On a, you know, a fairly typical valuation for SaaS businesses at this stage. And so that certainly consumes a lot of time. I think what we have found is the business has proved out to work, the story works, people like the idea, it's differentiated, but it's easy, well, let me rephrase that, building it out is still a heavy lift because what we're trying to alter is not just an AI engine, we're trying to alter the way people interact with their vehicles and how they solve problems and so there's a number of fundamental issues in there that we're trying to um, to rectify.
[00:23:43] So we spend a lot of time in the fundraising bit right now. But more importantly is preparing ourselves for the next stage of the business, which is business to business interactions, where the business that we have today, albeit still early and relatively small, we focus on consumers [00:24:00] and consumers only.
[00:24:02] My job is to help Justin when there's an issue with his Mazda 6. We know long term, though. That you still, and you said at the top, I'm not gonna do self repair, I'm gonna bring it somewhere. You're with the other 85 percent of Americans. So the question is, where should you bring it? Which shop is best prepared?
[00:24:23] to take care of that actual problem, where can you go, who has availability, and ultimately handing you off to the right shop, who's best prepared to go do this. And so that's where we become an aggregate connector between the two, but the AI becomes an important element of that, because if you're a shop, you may say, Hey, I'm a shop that specializes in Mazdas, but I don't do a lot of engine work.
[00:24:48] I do more suspension and body and electrical. You might be a, a shop that specializes in BMWs for instance, right? And you're trying to pull business away. But if you're an [00:25:00] independent shop and you're only targeting this sub segment of the market, how do you go do that? I mean, how do you go find those customers that really align to your skill sets?
[00:25:10] It's not that easy to do. No, it's not. Agreed. Agreed. Agreed. And for you as a customer, we had a customer call us the other day and I thought that he was actually out of Michigan, out of Ann Arbor, you know, your kind of your stomping grounds. They had bought their kid a BMW 5 series, kind of a used one from a friend of a family and it's a decade old and it ended up with some engine issue and he brought it to one local mechanic who said, yeah, we deal with BMWs, we're certified.
[00:25:37] And they said, boy, I don't know what this is. You're going to have to take it to the dealership. So they bring it to the dealership and the dealership said, well. We're not sure what it is, but for about three, two to 3, 000 of diagnostics, we can, we can figure out what's wrong and then the repair will likely be.
[00:25:53] 8, 000 to 9, 000 on top of that. So here's this family sitting with a 15, 000 car and a 12, 000 [00:26:00] repair bill at the dealership. Meanwhile, even the independent who specializes in BMW says, I don't want to touch this because I'm not really sure. And do you think the customer has time to go shop this around from independent shop to, I mean, you could spend three days just trying to get this vehicle around.
[00:26:20] And if it's not running, now it's on the back of a truck and. It gets expensive and I mean it becomes a very difficult question to align this problem to this particular shop. And I know there's a shop in the Detroit area that specializes in that engine and that particular problem, but how do you find them?
[00:26:38] Yeah, sure,
[00:26:39] Justin Grammens: sure. So you guys want to create this network or this, this platform so people can easily get connected to the right, the right expertise they need.
[00:26:46] Michael Petersen: I think it's very analogous to something that happens here in the state of Minnesota, and that's the Mayo. And if you think about what's great about the Mayo, and we're, we're so fortunate as a state to have the Mayo with us.
[00:26:58] But if you think about what's [00:27:00] great about the Mayo, they have great doctors, right? Great generalists. But typically what they do is they bring somebody in and they say, Hey, Justin, I think this thing that's going on with your elbow is some specialized, you know, mole, whatever it may be. Yeah, sure. I'm going to get you in front of this particular doctor who specializes in that particular area.
[00:27:20] And so very quickly, what they do is they redirect you to a specialist. Who's best equipped to deal with your specific problem because your problem might be one out of a million, but that doctor has dealt with that same issue 50 times in his or her life. Sure. And that's what makes them special because as a generalist doctor, you just can't, you can't be everything to everybody, obviously.
[00:27:43] And so to a certain degree, we're trying to do the same thing, Justin, which is how do I get this problem to the right specialist, and it may not even be a specialist, but the right person equipped to go deal with that particular problem. Sure. Sure.
[00:27:56] Justin Grammens: And to bring it all the way back. Yeah. You guys are using artificial intelligence to make [00:28:00] this happen.
[00:28:00] Make it easy behind the scenes. And if
[00:28:02] Michael Petersen: it wasn't for AI, I honestly don't know how you'd build this thing. Mm hmm. Any thoughts
[00:28:06] Justin Grammens: around conversational large language models and stuff like that? You guys are probably looking at that, how you could actually converse with this at some point, be a little more self serve from the customer standpoint or even from the mechanic standpoint?
[00:28:17] I don't know. Just, just kind of spitballing ideas here.
[00:28:20] Michael Petersen: No, no, I think you're, you're spot on. And I think there's two things. One is the LLMs are wonderful and we use them already in GUS. As a portion of the model, not the, not the core for both the intake side, as well as the, the output side, the challenge that we bump into is that if you use exclusively an LLM model in this, in this area is the LLM models are not great.
[00:28:45] As you know, of coming up with very specific recommendations for very specific problems, right? It's a language model. It's not an, it's not a machine learning model. And so I think. The LLM models are really important if they're appropriately used. The other [00:29:00] thing though that I think that we're doing is for the next generation is, is taking information in via language, taking information in through the existing computer system, which is called OBD II interfaces on most cars, so we're reading directly off the car, using actual audible inputs as well so you can actually listen to a tick or a groan or a moan.
[00:29:22] And you can actually do that through your, you know, through your phone so you can have the phone listen to it and start to match it up. And so that's where some of these things start to get pretty interesting on how do you dovetail all this together and LLM models is just, is just one aspect of that.
[00:29:37] Justin Grammens: Yeah. The more data you can throw at the mechanic or the AI model, the better prediction obviously you'll get regards to what the true source of the problem is. When you mentioned language too, I just wanted to clarify, you're talking about like, like English to Spanish translations and stuff, right? You can start communicating with other.
[00:29:53] Mechanics that maybe don't speak the native language? We
[00:29:55] Michael Petersen: can do that. I think even right now though, I would call it mechanic to English. [00:30:00] And what I mean by that is that, I mean that. Is some of the work that we're doing is that translating what the actual problem is to the actual something that's more easily understood by the layman.
[00:30:14] Our number one objective with Gus is to understand what the root problem is, like underlying what's actually broken, what do we need to fix? But when people describe an issue, they describe the issues in very soft terms, right? The car just feels squishy when I'm driving down the road. It kind of goes clunk, clunk, clunk.
[00:30:33] Or it feels like, you know, the wheels are going to fall off. Just sometimes it's, it's very squishy stuff. Yeah. Yeah. So what we use is the LM LM model, which has actually been trained by the mechanics because the mechanics know. How customers typically talk about particular issues. And then we use that as a feeder into the actual core.
[00:30:53] So we try to convert their natural language into something that can actually send Gus off in the right direction. And so we try to [00:31:00] use that as the conversion in that way. But, but then to convert it from English to Espanol or. I'm sure you know, pick your language. That part is relatively easy, I think.
[00:31:09] Yeah. But we're not there yet. We have not incorporated that in yet, Justin, just to be clear. Sure,
[00:31:13] Justin Grammens: sure. I'll focus on the US market first to begin with for sure. Well, good. This is awesome, Michael. Um, how do people get a hold of you?
[00:31:20] Michael Petersen: They can always find me directly at michael at raiseahood. com. But more importantly, if you want to check out the website, it's raiseahood.
[00:31:30] com. One long word, raiseahood. com. You can register for free and that part is easy and you can automatically, it will pull your vehicles directly into the garage and you can see a lot of interesting information about your particular vehicle and all we need is your license plate and we can kind of take care of the rest.
[00:31:47] So once we know your license plate and state, we know way more information about your car. Now it's just about, it's not about you as an individual, it's just about your particular VIN because all of that stuff is in public databases. And so, but we want to [00:32:00] know the specificity about your particular vehicle because that allows us to do better diagnostics on it.
[00:32:05] So we ask questions like what's your zip code, how many miles are on it, and whatnot. And uh, every time somebody uses it and it gets a little more, a little more intelligent every time it's exercised. That's
[00:32:15] Justin Grammens: fabulous. Well, cool. Well, thanks so much. I appreciate you being on the show today. We talked about a lot of stuff around automotive and, you know, we've got such a wide range of people that are listeners to this.
[00:32:25] And so, you know, it's always new topics, always new topics that we have people come on and, but I love the application of what you're doing. And like I say, it's all about applying artificial intelligence. So you guys sort of. Hit the mark on all those things, so I'll be curious to see how you
[00:32:38] Michael Petersen: guys continue.
[00:32:38] Well, I have to laugh because I attend your conferences, the Applied AI conferences, which are brilliant, by the way. But I listen to some just such bright people trying to solve really heady, heady problems. And then we're out there trying to determine if you have a water pump issue or a cracked radiator.
[00:32:54] And we feel kind of lowbrow in that respect. But what's unique about our business [00:33:00] is virtually everybody has had issues in this area. I mean, it's a very real issue. Like you said, it's applied AI. We're not solving world peace, but we're making everybody's lives a little bit, a little bit better when they actually have challenges.
[00:33:13] That's for
[00:33:13] Justin Grammens: sure. Well, great. I appreciate the time, Michael. You guys take care. Good luck on your, all your adventures and look forward to keeping in touch.
[00:33:22] AI Announcer: You've listened to another episode of the Conversations on Applied AI podcast. We hope you are eager to learn more about applying artificial intelligence and deep learning within your organization.
[00:33:33] You can visit us at AppliedAI. mn to keep up to date on our events and connect with our amazing community. Please don't hesitate to reach out to Justin at AppliedAI. mn if you are interested in participating in a future episode. Thank you for listening.