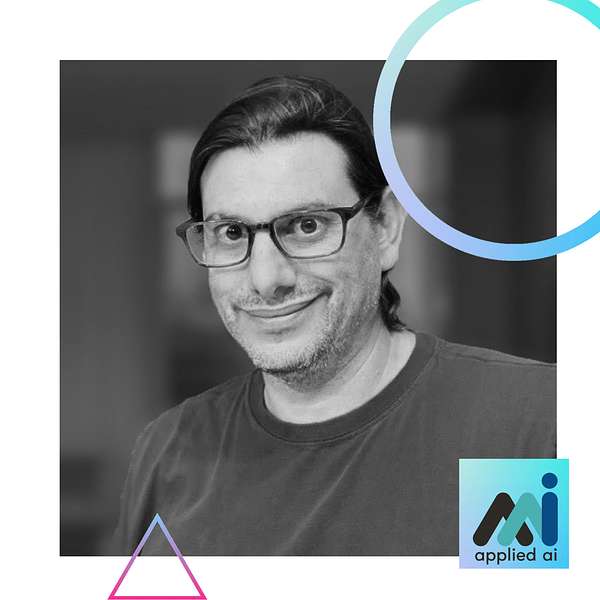
Conversations on Applied AI
Welcome to the Conversations on Applied AI Podcast where Justin Grammens and the team at Emerging Technologies North talk with experts in the fields of Artificial Intelligence and Deep Learning. In each episode, we cut through the hype and dive into how these technologies are being applied to real-world problems today. We hope that you find this episode educational and applicable to your industry and connect with us to learn more about our organization at AppliedAI.MN. Enjoy!
Conversations on Applied AI
Alex Muller - Deploying AI Easier and Faster Using Turn-Key Solutions
The conversation this week is with Alex Muller. Alex is the founder of Savvy AI, where he and his team run a mission to make AI work for all of us.
Previously, he co-founded GP Shopper, which was later acquired by Synchrony in 2017. During his time there, he served on their investment committee, where he helped the venture team evaluate prospective venture investments. His current interests are innovative AI ML-based products, tools, and companies.
And supporting fellow diverse founders to refine their operations, technology, vision, and strategy as they go through their entrepreneurial journey. He was named by Forbes as a top 10 CEO disrupting the retail industry through technology.
If you are interested in learning about how AI is being applied across multiple industries, be sure to join us at a future AppliedAI Monthly meetup and help support us so we can make future Emerging Technologies North non-profit events!
Resources and Topics Mentioned in this Episode
- How AI is Changing Every Industry
- The Top 10 Industries Using AI Today
- How AI is Changing the Retail Industry
- What is the Difference Between Artificial Intelligence and Machine Learning?
- A Beginner's Guide to Artificial Intelligence and Machine Learning
- GPShopper Acquired
- Savvy AI
- GP Shopper
- Synchrony Financial
- AI (Artificial Intelligence)
- ML (Machine Learning)
- Data Science
- MLS (Machine Learning as a Service
- IoT (Internet of Things)
- Reinforcement Learning
- Star Trek
Enjoy!
Your host,
Justin Grammens
[00:00:00] Alex Muller: You know, you'll never find a company who's deployed an AI solution at scale going, Oh, now I'm going to go back to not AI, right? Netflix will never go back to not using AI, uh, Amazon will never go back to not using AI, Google will never go back. People build, deploying, uh, applications that tell you who should get sneakers will also never go back to not using AI.
[00:00:22] AI Voice: Welcome to the Conversations on Applied AI podcast where Justin Grammons and the team at Emerging Technologies North talk with experts in the fields of artificial intelligence and deep learning. In each episode, we cut through the hype and dive into how these technologies are being applied to real world problems today.
[00:00:41] We hope that you find this episode educational and applicable to your industry and connect with us to learn more about our organization at AppliedAI. mn. Enjoy.
[00:00:53] Justin Grammens: Welcome everyone to the podcast. Today we're talking with Alex Mueller. Alex is the founder at [00:01:00] Savvy AI, where him and his team run a mission to make AI work for all of us.
[00:01:04] Previously, he co founded GP Shopper, which was later acquired by Synchrony in 2017. During his time there, he served on their investment committee, where he helped the ventures team evaluate prospective venture investments. His current interests are innovative AI ML based products, tools, and companies.
[00:01:19] And supporting fellow diverse founders refine their operations, technology, vision, and strategy as they go through their entrepreneurial journey. He was named by Forbes as a top 10 CEO disrupting the retail industry through technology. Thank you, Alex, for being on the
[00:01:33] Alex Muller: program today. Oh, thank you. Awesome.
[00:01:36] Justin Grammens: Well, cool. Maybe you could give us, just as a first question, sort of an opening question, I have said a little bit about maybe your background and what people know publicly, but maybe you could tell us a little bit about the trajectory of your career, kind of how you got to Savvy and, you know, what was the path to get you there?
[00:01:49] Alex Muller: Sure. So probably if we really look about how we ended up at Savvy, we have to start doing my first startup, GP Shopper, where In 2007, we started this company called Cheapy Shopper, focused [00:02:00] on being a mobile platform for retailers, brands, and financial institutions. Fast forward, one of the brands we started working with is Adidas, and they were doing these things called Adidas Confirm, which is an application.
[00:02:13] That let people sign up and participate in a lottery like program to get a reservation for super high demand shoes for what were, you know, probably now not so much in demand, but at the time, really high demand Yeezy sneakers. Basically, 500, 000 people would ask to get a reservation for one of the shoes, but only 1, 000 people would get it.
[00:02:32] So, one in 500 actually got the shoe. Crazy. It was kind of crazy. You know, when we deployed that application for Adidas, for 25 minutes, a 50 percent company was serving Facebook traffic. So it was kind of wild. The interesting thing about that is the first few times we did it, we did it purely on random.
[00:02:51] And we realized that there were some percent of the people who would reserve the sneakers. And not actually go buy them. They were just trying to get the reservation. So we thought, [00:03:00] how can we improve that? Well, and then we thought, well, let's use a machine learning algorithm to determine who is most probable to actually reserve the shoe and actually buy it.
[00:03:09] Because the goal was ultimately to sell the shoe. Sure. And so we turned to machine learning algorithms. And they worked, and I mean, they work well, and it's funny because now it's like, in hindsight, you're like, well, of course, AI works, you know, you'll never find a company who's deployed an AI solution at scale, it's going, oh, now I'm going to go back to not AI, right?
[00:03:30] Netflix will never go back to not using AI. Amazon will never go back to not using AI. Google will never go back. And, you know, people build deploying applications to determine who should get sneakers will also never go back to not using AI. A few years later, the company was actually bought by Synchrony Financial because we started enabling some mobile fintech solutions.
[00:03:50] And after the company was bought, the CEO of Synchrony Financial, Margaret Keane, comes to Maya and I, who are the co founders of GP Shopper. She says, what's next? So we think about it. We'd already [00:04:00] fallen in love with AI. We basically created a vision for a completely AI enabled direct to consumer credit program.
[00:04:06] That will no longer just look at FICO, but it'll look at all these different things, have natural language processing, be machine learning algorithms for determining who should get which credit offer, algorithms for optimizing how that's collected. All sorts of AI, even rewards were driven by AI. And, you know, everybody loved it.
[00:04:24] We spent from 2017 to 2020 building it. We spent over 20 million between people, hardware, and software. And then three years later, we're ready to go live, but COVID hit. And so the world pivoted and this whole experience was about travel and entertainment. So those were the two things that weren't going to happen in 2020.
[00:04:45] And, you know, at that point, you know, as founders often do after three years of being the acquired company, you're like, okay, maybe it's time for something different. Yeah. And we left. And so this is how we got into Savvy. We actually left, we moved out to California, we [00:05:00] were living in Chicago at the time, and we started hiking.
[00:05:03] And one day we took a hike, and we walked the trail backwards. And I told Tamaya, I think everybody's doing AI wrong. And she goes, no, they're not. And I said, I think they are. And the reason she was joking and kept saying no is because she knows exactly what that means. You're onto something else. Exactly.
[00:05:22] We just spent 13 years between starting a company, finally selling it, and finally having a little bit of fruits of our labor. And we were in that hype was the moment where we knew we were going to be jumping back into a new startup. I told her the idea, we had done AI like everybody else, focusing on the data first, building the algorithms, and then seeing what use cases we could apply.
[00:05:44] And I feel like if we focus on the use case first, define the goal, then request the data, you actually have a far better, far cheaper way of deploying AI. And that's how Savvy was born. And Savvy is a decisioning first AI that's really about [00:06:00] helping you decide between options A, B, and C for things like recommendations.
[00:06:04] decisions, classifications, and can also serve in predictions as well. So that's about how we got there. That's fabulous. And then for the last three years, we've been helping companies then deploy machine learning at radically cheaper rates than, say, the legacy process. The companies are used to even today.
[00:06:23] Yeah, that's awesome.
[00:06:24] Justin Grammens: And so there's, there's people that listen to the podcast and maybe, you know, they don't understand maybe what the difference between machine learning and artificial intelligence is. I'd like to ask people on the show, you know, how would they define AI as sort of like one potential question, but also, yeah, how, how do you view machine learning and AI sort of co mingling together?
[00:06:41] Where does one work versus the other? Are they a subset of each other? Curious to know
[00:06:44] Alex Muller: your, your thoughts. So, okay. So let me tell you my definition of AI first. 79 AI is an umbrella term for basically any computer that can learn. Think about it for a second. If any of you out listening to this podcast have ever developed software before the AI period, [00:07:00] everything was command and control.
[00:07:01] If x, then y. If a user comes to this page, show them these options. AI is very, very different. In AI, you do not want to define the x and the y. You define the goal or the strategy. Okay, I want to increase conversions. And you let the AI decide what options to show. That's it, you know? Mm-Hmm. . When you're thinking about it, if you think about everything from self-driving cars to generative ai, they are all different software applications that have learned now.
[00:07:32] It's kind of like machine learning and AI are a difference without a distinction, and it's just different people like using different terms. If you're talking to actual data scientists, they often prefer the term machine learning. because they feel like AI has taken on like some other meaning. But for the vast majority of the world, everything that is a machine learning application is an AI application.
[00:07:54] And there's really no difference. Agreed.
[00:07:57] Justin Grammens: All right. Yeah. No, that's, that makes a lot of sense. [00:08:00] I, there's actually an interesting graphic that I've seen put up by Google. I use it in my class that I teach. I teach a class on machine learning, IOT and AI where it's, yeah, it's like, you know, back in the early days, back when I was programming and probably when you, when you were programming as well, you know, basically there were inputs that were put in and it was some logic there that was in the middle that was all hand coded and then there were outputs and it was that thing that you needed to hand code.
[00:08:22] And I think what we realized is over time is, is if you can feed in enough data on the way in. And an update on the way out, it can start creating the code for you. For lack of a better term, right? Creating a model, that's really what it's doing. But it's making those sort of decisions.
[00:08:34] Alex Muller: Sometimes I like to explain it is, because a lot of people understand the kind of the if then, a lot of non developers understand like the if then scenario.
[00:08:42] Yeah. It's like where someone usually creates code that says maybe 20 if thens in a particular situation, machine learning will be producing 10, 000. And then deep learning would be producing a billion, right? And it's just, you know, very, very much more [00:09:00] fidelity in your scenarios. Yeah.
[00:09:02] Justin Grammens: So it seems like what you've done is obviously you got deep into this, into this machine learning AI technology.
[00:09:08] You went through a couple of different, one prior product, and then you, you sort of have built now this platform, right? Is that how I could explain Savvy as I, and you're focusing on people that maybe don't need to understand how. ML Ops works or I don't need to have a data scientist on, on staff. Maybe, maybe talk to some of the scenarios, I guess, that you're seeing your software being used for.
[00:09:27] And if I even have it, right.
[00:09:29] Alex Muller: You have it correct. I mean, it's funny, like there's, I feel like maybe sometimes investors like the word platform. I kind of like the word tool. Because, one, I, I feel more akin to our clients in that our clients tend to have this need. And their need isn't AI or ML. Their need is they have a decision tree, but the decision tree isn't getting any smarter because it's static.
[00:09:50] And they want it to make better decisions over time. That's the actual need. And AI is a great tool to solve that need. And the whole [00:10:00] purpose of our product is to give people those tools without needing. to understand the deeper machine learning statistics involved. And the tool will provide its own transparency, tells people why it's made a specific decision.
[00:10:15] It's completely auditable. It's very useful in financial institutions. And that has been the driver. The driver is solving the problems that we had at Synchrony. Without having to build everything in, by the way, this isn't a new thing in software. This is like rinse and repeat. Every time a new software technology comes out, the original uses of that technology requires people to build it from scratch almost every time.
[00:10:38] Think about e commerce, right? Who would build an e commerce engine now? You know, you would just use Shopify or eCommerce cloud on Salesforce eCommerce cloud, or you would use some other big commerce tool. You would never go out and custom build your database and custom build your web pages and your checkout flow.
[00:10:56] That's nonsensical. So the same could be said [00:11:00] for cloud management. I mean, where have all the database admins gone, right? Nobody hires DBAs anymore. Data scientists will go the way of DBAs. In other words, there will be data scientists. They're working on the engines. I'll still hire them because I will build out an engine, but the people using the engines won't need to understand those kind of deeper layers.
[00:11:21] There'll be people who can understand the engine, but they don't need to know how to build the engines. Like when you drive your car, right, unless something is going real wrong, you use a mechanic. If not, you're just driving from point A to point B. Right. Right. Yeah.
[00:11:35] Justin Grammens: And I think what's, what's interesting, you know, as I've been looking through your, your website and I, and I even, you even gave me a sort of a rough demo and been playing around with it.
[00:11:42] 'cause you guys have like an introductory, like, hey, kick the tires for free.
[00:11:45] Alex Muller: Right. Only in special cases. Special cases, yeah. Only for you. Yeah. Well, thank you. I, I appreciate that. I, I also do wanna talk
[00:11:52] Justin Grammens: about your, I have no data angle that you guys are coming at it with, but it's cool because you guys are focused across a lot of different industries.
[00:11:58] But maybe what you're also hitting on [00:12:00] is a bunch of different roles within an organization. Right. If you're a business leader or a product person or even data team, it feels like anybody in the organization could probably glean some value from using your, your tool. Yeah,
[00:12:10] Alex Muller: absolutely. We tend to want to lead with the product manager or the data analyst.
[00:12:17] Now notice I'm not using the word data scientist or even the CTO. Why do I say that? Because. Let's start with a product manager. Oftentimes a product manager is a person who has a vision for the next generation of their product or the next version of their product. And they're saying, okay, I use e commerce sometimes as an example because everybody shops, right?
[00:12:34] I have a checkout page and it's listing 20 different payment options. But you know, showing 20 different payment options on a mobile phone is really kind of not great UX. So I want to pick the best three payment options, whether we're using a firm or Buy now, pay later, or a credit card, or cash app, or Amazon Pay, or Google Pay, right?
[00:12:56] I want to pick the best three for this user, and that's a great machine [00:13:00] learning decisioning algorithm, right? That's like, Justin gets one, Alex gets a different, Maya gets a different set of three options based on the ones that are most likely to actually convert. The person who's going to make that decision to put that functionality into their mobile shopping site or into their mobile app.
[00:13:17] is the product manager. So that's why we're saying, like, let's start with you. Can you define that decision? And they almost always can, or somebody on their team can, because it tends to be like, here are my decision options. Here's my goal. You know, I track conversion rates. And here are the data points that could influence that goal.
[00:13:33] Then what Savvy would do is auto create the recipe for that machine learning, including the API endpoints, or even JavaScript endpoints. Then, that product manager can literally copy and paste the documentation for those endpoints into a Jira ticket, and then developers can use them, and basically, you're on your way.
[00:13:53] That is kind of where Savvy comes in. takes hold from a usage perspective, then we have other users like a [00:14:00] data analyst may also be in that similar role, oftentimes reporting into the product team, and then sometimes you have what we call pure analyst users, because one thing that we've bolted into our product is like an Excel like interface, where we now have people who basically use Google Sheets or Excel as their primary workspace, can now interact with Savvy and get true machine learning.
[00:14:22] Thank you. into their Excel spreadsheet for things like predictions or decision automation. Neat, neat,
[00:14:28] Justin Grammens: yeah, yeah, yeah, that's, that makes a ton of sense. So start, start with the business problem, like you're saying. It's the product owners or the, you know, the people that are working on the product side are the ones who actually have the issues or they have the vision, right?
[00:14:39] They kind of say, I want my product to do X, Y, or Z. And they can easily kind of get up to speed and start getting, I guess, get the, get the end points even set up, you said to begin with, or at least show some examples. You guys have really, really good API documentation where you're talking about, you know, predicting ice cream amount ordered or term loans by month.
[00:14:57] I mean, I'm curious to see how [00:15:00] it's being used in various industries, how our customers seeing you guys plug in.
[00:15:04] Alex Muller: And you are completely right that there's nothing about our product that couldn't be used in almost any industry. Having said that. You know, because we are a startup and we only have so much resources, our main focus from an industry perspective has been financial services and fintech with insurance and logistics next.
[00:15:21] The reason that has been our main focus is because banks and financial institutions and fintechs have the three most important things. for machine learning applications. One, they have data rich environments, they have operationally relevant problems, and those problems recur all the time. I'll give you a good example.
[00:15:40] One of our clients, uh, uses us to determine if they should accept or reject an ACH payment. They have to accept or reject At this point, thousands of ACH payments a day because they're enabling payments in all sorts of industries. And because they're guaranteeing, in many respects, some of those ACH payments, if they [00:16:00] accept a payment that does not go through because the person doesn't actually have the money on the other end of that payment and only find out three days later, they're on the hook for that.
[00:16:08] So much like a credit card decline, they have to have a decline process. They used to use just a decision tree. That's basically if X and Y, they then went on to using machine learning and they found that they were able to reduce their decline rates by 30 percent saving them hundreds and hundreds of thousands of dollars per month at this point in order to get a better outcome.
[00:16:30] And what's really funny is they've maintained because our product lets you do both machine learning based goal optimization and also put guardrails so that you can be comfortable. And also, this is where I'm going to talk about the cold start as well. So they did what we call a cold start. In other words, they did not have all the historical data in place to pre train any models.
[00:16:52] So they just wired everything in vis a vis the APIs. So they built out their use case, it auto generated the API microservice, [00:17:00] they put, they connected to the APIs, and then within a few weeks, the model started to build. And then within a couple months, they got to a 20 percent reduction. Then six months later, they got to a 30 percent reduction in NSF events.
[00:17:13] And do you know what they did between wiring and the APIs and six months later and a 30 percent reduction? Absolutely nothing. They just let it get better and better. Every day, the system would collect more data. Every day, the models would build. Every day, the models would build a little bit better.
[00:17:28] Every day, the system got smarter. And now, it's almost like fun. Well, I find it fun. The other day I was just saying, every once in a while I look at their data and I'm, you know, I was looking at, you can look in our product and say, oh, here it made a rejection decision. I wonder why. So I clicked into the rejection decision and found that somebody was using a routing number.
[00:17:48] And I'm not going to put, say, the routing number, but let's just say this routing number was built to actually fake. Banks into thinking there's an actual account number and money on the other side of that [00:18:00] routing number. So it's like, use this routing number. Somebody thinks you just made an ACH payment.
[00:18:05] Now, the reason that routing number exists is because. A bank needed a testing situation to test production ACH without actually moving money. Oh, sure. So he created a, essentially, think of it like a fake bank. Somebody knew about this fake bank and used it to create a synthetic fraud situation for this ACH payment.
[00:18:26] What happened is... Maybe the first one was accepted because it didn't know any better. It realized it got rejected, and so it basically learned. After a couple times, it learned to reject anybody coming out of that routing number. And you know, it's like there are thousands, if not millions, of ways of creating bad actors doing things like that.
[00:18:46] And the only way to find those people, you're just never gonna keep up from a human perspective. Only AI can find that. And that was an example of how, like, Wow, AI is learning, you know, it's learning the millions [00:19:00] of events that people just could not track. You could not, in a million years, learn as much about different people making payments as the AI has learned from the hundreds of thousands.
[00:19:11] Of transactions at the process, the model is super powerful.
[00:19:15] Justin Grammens: Yeah, no, it reminds me of like, you know, AI is learning how to play the game. Go, right. Because it's seen enough of it and it knows the parameters and essentially it's learning the underlying rules to figure out what the moves it should make.
[00:19:29] And so in this particular case, yeah, there was no human in there. Putting in this special routing number, it kind of picked that up over time that it should be a rejection.
[00:19:36] Alex Muller: Exactly. And you know, it's funny too. It's like a lot of people like, even in the Go case, and this is where it's interesting to debate.
[00:19:43] Is it learning the fundamental rules? Or is it just learning the probability that in this scenario, the next best action is this? Without fundamentally understanding anything. Like, in other words, the AI doesn't know that that person is a bad actor. It just knows [00:20:00] that routing number leads to a probable failure.
[00:20:02] It has no judgment about the person. Or the underlying thing, it just has the probabilistic outcome understood. Sure. Yeah,
[00:20:11] Justin Grammens: which ultimately at the end of the day, right, it's just, it's just fancy math that's going on inside of these models.
[00:20:15] Alex Muller: And it's so funny because as the fancy math gets more and more sophisticated, human beings who reason in a very different way, I mean, I guess our intuition is purely probabilistic, but we don't like to admit it.
[00:20:26] We like to think about ourselves as being, like, reason based thinkers. And in some respects we are, in many respects we can do things computers cannot. Because of that, you know, even when ChatGPT does something super sophisticated and everyone's like, it just passed the bar, or it just passed the MCATs, it's still just...
[00:20:46] wrote memorization, reciting the highest probable answer at any given or the next best probable word in any given paragraph. Right. And what's wild to me is that it creates this illusion [00:21:00] of intelligence when it's really Yeah.
[00:21:06] Yeah,
[00:21:06] Justin Grammens: you're right. And that's, that's the crux of a lot of these debates, right? The whole idea is it, is it sentient or not? Is it actually understanding the underlying structure or is it just essentially being programmed what it's seen, you know, a million times before? Which is what humans do too, right?
[00:21:22] We have to experience things and then hopefully we get more and more intelligent.
[00:21:27] Alex Muller: Yeah, my favorite example of that. Are you a football fan, Justin? Can you answer, and maybe you can, maybe you can't, the time between the hike and the throw for Tom Brady, the average time of his Uh, a couple seconds maybe?
[00:21:40] Exactly. 2. 18 seconds. In that time, on average, he does seven progressions, and he was once asked in an interview, how do you decide? He goes, and I think he said in this interview, I don't, and what's reality there is like, you know, his brain has been trained. by hundreds, [00:22:00] maybe thousands, maybe tens of thousands of throws.
[00:22:03] Yeah. Now his brain will automatically make several predictions as somebody's running down for each progression. Can I make the throw to that person? Is that person likely to capture it? Is that ball likely to get intercepted? He makes these three predictions in his brain for every progression. And the one that is optimized the best, he makes the throw.
[00:22:23] Yeah. And, you know, I'm definitely not a Patriots fan. That's, that's, that's amazing. Like, he made it to the greats of all times because his brain was probably best trained. to run those three predictions on each of those progressions. Yeah, for sure. So to me, humans have two layers of thinking. You know, there's the intuitive, trained response, which works pretty much the same neural, deep neural net, you know, in his brain, like any other human brain, where there are a trillion base pair relationships, right, trillions of base pair relationships.
[00:22:53] You know, it's pretty comparable to the trillions of neural nodes[00:23:00]
[00:23:01] Yeah. The compute's not, you know, at least we're in the same order of magnitude. Yeah. Right. Right. And then the humans can do something else, which is really fascinating, which is we can then layer in reasoning logic. And I, you know, what's funny is I was talking to a bunch of computer scientists, this is the next evolution is, can we go from.
[00:23:19] really great intuition in computers to go to, can they create underlying reason, right? Things like solve, that gets to a place where you're, you're basically doing like math proofs and things like that, which they don't do now, really. Right.
[00:23:35] Justin Grammens: Yeah. And they, they can't add numbers or multiply too well, you know, either because back to your point, they don't understand the underlying structure of what multiplication actually means.
[00:23:43] They've just seen enough examples. Thanks. to simulate it in a lot of ways, but...
[00:23:47] Alex Muller: Exactly, which is, by the way, we do it too, in a sense, like, we can then do complex math, but for simple math, if someone asks you what's 2 plus 2, you say 4, you don't think about it. That is now a trained response. Right, [00:24:00] yeah.
[00:24:00] Remember when we were young and going through, like, One through 12 in our math tables, all those became trained responses. You know, we didn't go, okay, well, three times three is nine, three times six is 18. Like we didn't start doing it that way. Right, right. It
[00:24:18] Justin Grammens: takes practice. I mean, think about learning a new language, right?
[00:24:20] If I were to pick up Spanish 10, but A couple times, three or four times through it, maybe I'll start picking up the, just the trained response.
[00:24:30] Alex Muller: Well, what makes human intelligence super interesting and why we love to combine human intelligence with machine learning in our product. So we, we say here, here's where you put in your goals and your decisions are made by machine learning.
[00:24:41] But please add, if you'd like some guardrails, I'll give you a couple of great examples where it's like machines are great in the center of that data bell curve. Right, where they can learn, you know, with a lot more fidelity what's likely to happen. But you go to the outside of those kind of like edge cases where there aren't a lot of data points.
[00:24:59] [00:25:00] That's where humans can do something machines can't. Humans can intuit what would likely happen even when they have no fundamental data and they haven't trained on anything yet. And that's where it's like, if you can start, like in a good example is in that same ACH use case, early on, we saw something that was kind of funny.
[00:25:17] One time the, the ACH system allowed somebody with a negative bank balance to kind of go through and you're like, why would it let a negative bank balance go through? And it's because it was the very first time it saw a negative bank balance. It had no idea that it was likely to fail. If we would have let it just kind of go on, it would have eventually learned that bank balances are likely to fail.
[00:25:42] But I'm like, why did we even need to bother with that initial learning? We could have just set a rule. If the rule, if it has a negative bank balance, just reject it outright. Yeah. Right? And you're just like, and that's a good example of like, you can reason that, you don't need experience for that. Yeah.
[00:25:58] Justin Grammens: Yeah, sure. So your, [00:26:00] your tool allows for those sort of just hard, you know, hard fast rules or facts or whatever to be like, you know what, reject this or make this prediction because of this. That's awesome.
[00:26:08] Alex Muller: Those guardrails supersede the AI. And what the reason we put that in is number one, what we learned at the bank is nobody wanted to go live with AI without housing some guardrails in place, right?
[00:26:19] You didn't want to get that gutter balls.
[00:26:22] Justin Grammens: I like the idea of guardrails.
[00:26:23] Alex Muller: Yeah, for sure. Exactly. And that's why we call it guardrails. Part of me was joking, maybe we should call it training wheels because that's kind of like, you know, what, but guardrails are a better term. The idea here is we just want to make people comfortable also going live with AI and the easiest way to make them comfortable is like, look, your AI will bound its decisions between A and B, right?
[00:26:42] So you can be completely comfortable with its decision it's making and look for optimizations. And then when you're really comfortable with the models, then you can kind of widen your guardrails and that way it gives people more comfort. That the AI is going to behave the way they want it to. Sure, sure.
[00:26:58] I mean,
[00:26:59] Justin Grammens: do most people use [00:27:00] it for kind of, I mean, are they labeling their data and it's more of a supervised learning sort of, uh, application? Or are you guys looking at any sort of reinforcement, unsupervised learning, isn't it? Actually,
[00:27:10] Alex Muller: I would say most of it is unsupervised reinforcement learning.
[00:27:14] Really? Okay. So when I think of labeling data, and maybe you have a different view in mind, I think of like, I have an image and I'm labeling this image and I'm trying to train it that way. In our cases, they're like mostly decisioning use cases, like what truck should I roll? Which warehouse should I process this order from?
[00:27:31] Which offer should I show you? And the reinforcement is what actually happened. So our product has basically two APIs or, or if you use a JavaScript, it's got like two JavaScript functions. First is get the decision. The second is what actually happened. And by hitting both of those APIs, we have the cause and effect, and by capturing cause and effect every day, every night, every hour, reinforcing the data set and retraining the models.
[00:27:58] So [00:28:00] now, some of our clients go with, well, with that in a purely unsupervised way where the, the effect is the actuality and that. It's retraining the model itself. And what's great about that is it's really self learning and then you don't need human in the loop there. Right? Yeah. And people love that because no human in the loop means no effort and just benefit.
[00:28:20] However, there is another thing called fleet learning, which I also love, which some clients are doing too, where now the difference is. Instead of making a decision, it's making a recommendation to somebody else who's a subject matter expert who then can agree or disagree with the recommendation. I will call that human in the loop learning.
[00:28:39] And when you think about this in terms of like, let's say you have a bunch of HVAC experts helping people understand which type of air conditioning unit they should use in a different situation. This is the kind of thing that Most people don't have a lot of knowledge on, and it's good to get like an expert's advice.
[00:28:58] Somebody calls in and [00:29:00] they say, here's my situation. Here's how big my floor plan is. Here's this. I'm in this part of the world. It rains this often, and the HVSE expert says, okay, this is what you should use, or here's the part that you should use. If you wire that decisioning and you support it with the decisioning or recommendation ai, what happens is the 10 people you have on the phone helping thousands of customers.
[00:29:22] are now training a model on how to be one of those 10 people. And so now what you can do is as you grow, you may not have to add another 5, 10, 20 people to that business unit because the AI can start to recommend and then auto decision for some of these people in some of the scenarios. And we definitely have had clients who, they themselves have had, you know, one client uses us to classify transactions.
[00:29:47] And they want to determine what's short term income, long term income. Or not income at all to give people a broader loan package, right? Instead of just using what's on, it's really admirable. Instead of just using what's on their W 2 [00:30:00] income, they want to use the fact that they might be driving Ubers, right?
[00:30:04] Some 10 gig economy, or they may want to use the fact that they get 2, 000 every month from their grandmother, right? As part of their view of income so they can give them better loans. And in this case, it was a classification AI where they have human beings reading all these transactions. Now, what they've done is into that UX that they had where a human makes a decision, they wired in Savvy, so that Savvy, A, started recommending the decision.
[00:30:29] And then Savvy would tell it the confidence of the recommendation. So Savvy's like, I'm 95 percent sure that this decision should be short term income. They were like, great, we're going to automate everywhere it's already confident and just serve where it says either unknown or low confidence. Yeah. And now the same five people have 5X the traffic and they haven't had to grow people because the more they train the model, the more the model drives the rest of it.
[00:30:59] And so, [00:31:00] you know, we're now served like almost two, two to three million different classifications automatically. Which, even if each one takes 10, 15, 20 seconds, you're talking about almost a million dollars of cost savings. Yeah. Yeah, for
[00:31:14] Justin Grammens: sure. And like you said, like, people could build this in house if they wanted to try and stand up all of this stuff, but guys are sort of doing the legwork behind
[00:31:23] Alex Muller: the scenes.
[00:31:24] And you know what? All our clients do feel it's in house, because we're just a tool. So the best part about it is... And this is a lesson I learned. If you build good tools, you are helping companies build in house, and that means you're better from the psychology, like, we're not a consultant saying, let's figure out the AI for you.
[00:31:44] We're saying, here's a tool that you can use to figure out AI for yourself. And you just don't have to worry about heteroscedasticity in the dataset. And I think that's kind of our, you know, it's funny, when somebody builds a Shopify [00:32:00] e commerce site, they say they built it, right? They pick the UI, they pick what goes in what page, right?
[00:32:08] That's how we want people to feel. Yeah. So
[00:32:11] Justin Grammens: over time, some of these outputs can also change though, right? I mean, so I guess what I'm trying to say as well is, is the dataset might change, right? There might be different outputs that you hadn't thought about to begin with.
[00:32:22] Alex Muller: Absolutely. And this is where, you know, because the tool in our case is pretty easy to use, we recommend an iterative approach.
[00:32:28] Mm hmm. Sure. Where you take a client. And the system will eventually level off at a level of intelligence depending on how much data it's getting. And it'll say, okay, you know, it's getting this predictive. We have this savvy predictability score. It's like you're reaching this number. And then the client's all kind of, it becomes a game, like what more data can I add to get it even more predictive?
[00:32:49] Yeah. And so like, okay, maybe I'm going to start adding weather data from some shopping patterns or maybe I'm going to start adding interest rate data for some debt collection patterns. [00:33:00] And people are like, well, more data to get it smarter. And because the product will tell you right away if that data is making an impact in its predictability.
[00:33:09] And so it's kind of like people think about it that way. And I think that's where this is going to, like. And there is an interesting thing, sometimes too much data actually works against you. That's something we don't realize, like if you have too many columns against the number of rows, it creates a lot, a really noisy model.
[00:33:26] So sometimes it's about like I'm putting in 20 different data elements, what we call influencers. We don't call them model features because we're trying to get away from the language of data scientists. And if you call something, a feature to a product person, they're like, yeah, that's checkout checkout's a feature.
[00:33:40] You know, age is not a feature. . Right. Yikes. .
[00:33:45] Justin Grammens: That's true. That's true. The younger you are.
[00:33:47] Alex Muller: Yeah. Age is an an influencer. Right? It it's an influencing data point. Or it's a data input. Yeah. So the more you here is that, well our goal was to like make that process simple, but also give [00:34:00] people some rules of thumb.
[00:34:01] So we have this like notion of like. If you put in too many columns, but you only have 10, 000 records and you put in a hundred columns, you're going to have really bad what we call data coverage, right? Where it's like. You're not in, in the 10, 000 records, you're not going to cover all the different parameters of 100, 000 columns of data.
[00:34:21] So we'll say here are the 10 columns that matter the most. Consider turning off these other columns or just create noise. Yeah, exactly.
[00:34:29] Justin Grammens: Exactly. As you were talking, I was looking through some of your, your examples and one of them was this ice cream picker, right? So. You know, you decided between chocolate, strawberry, vanilla, raspberry, you know, maple, walnut is what you have as examples.
[00:34:40] And those, those things might change over time. I might actually introduce a new flavor or I might remove a flavor, right? So it feels very fluid. You can kind of choose what the decisions are along the way and the model will adjust and
[00:34:52] Alex Muller: go over time. And the model will self adjust and that's a great point.
[00:34:56] And it's funny because we, um, we use the ice cream picker as kind of the conical [00:35:00] example because everybody likes ice cream. And it also gives us a good example of where you'd set a guardrail, right? Where, like, for instance, one of the ice cream is, I think, maple walnut, and clearly if you have a nut allergy, it doesn't matter what the AI thinks of your taste recommendations, it says if you have a nut allergy, don't show them maple walnut.
[00:35:17] Yeah, right. And, and. Just shortcut it. Exactly. Let's make sure we don't get somebody sick, even though there's nothing about your allergies that kind of imply your taste profiles. Yeah. So this
[00:35:27] Justin Grammens: is a question that comes up a lot when I talk to, you know, clients of mine or you know, anybody that's loading data into a, a system, are people asking you about security and you know, or what are you doing with my data and how is it protected and safe?
[00:35:41] Alex Muller: Because we came from a bank, we've architected our own product with bank level security in mind, right? And so, you know, data is never, so our product is essentially single tenant. What does that mean? And every time a new client gets on Savvy. We spin out a new set of servers. To support that client [00:36:00] and it auto spins out their own environment, storing their own data, own models, own API gateway, which is also great for, uh, for a load balance because now we don't have to load balance every client has their own end point.
[00:36:12] And so we don't have to worry that one client's driving thousand requests a minute while another client was driving 10 was a lesson. We learned the hard way at GP shopper when we had a multi tenant system. And so one of the things here is that because no data is ever intermingled and you could if you want to move that entire, we call those things client containers into your own VPC, you know, we think of data, you know, as we think of all the different processes, rules by which a bank can't buy software, and we've met them.
[00:36:42] So we're SOC 2 compliant, and we don't intermingle data. Data is always encrypted at rest and in motion. And, you know, we also highly, we never recommend PCI or PII data. Week one, it's also terrible to model on. If you give me a social security number, it's unique. [00:37:00] There's nothing about a social security number I can use to make a machine learning model.
[00:37:03] Yeah,
[00:37:04] Justin Grammens: so you might as well just use any generic ID, you know, in some ways.
[00:37:07] Alex Muller: Yeah, well, you don't use an ID, don't model on IDs. Like, you want to model on attributes that people can share and so that you can find a pattern. So for instance, if you're doing a marketing application, you can say, okay, I'm, I'm looking at gender, I'm looking at age, I'm looking at location, I'm looking at job types, right?
[00:37:26] But if you're doing a financial thing, some of those are illegal. You can't use gender and age, right? But you can use things like what's in the bank balance or. You know, number of transactions per month or different things like that, that are all about, all about the legal things that don't have any fair lending law implications.
[00:37:44] Justin Grammens: Yeah. It makes sense. Makes sense. Yeah. I just think, and as a person sort of travels through somebody's website, they probably want to tie it. And again, I don't know if you guys have this concept, but I mean, if it's a recommendation engine, for example, it's probably going to want to know there's this amorphic person who kind of buys these certain [00:38:00] types of things or.
[00:38:01] Or doesn't, and they have this sort of foot traffic through an e commerce site, trying to predict like what they would go and buy next. It's, it's, it's an interesting thing. Like, you know, do you want to tie that back to a specific idea or does it, or like you say, does it, does it really matter at the end of the day?
[00:38:16] Alex Muller: So I'm going to challenge this concept that you're, you're thinking about a person at all. And are you thinking about, because even me, me 10 years ago is not the me now. One of the challenges we had at the bank was Trying to build machine learning models when they had this huge data warehouse and one of the problems is they kept overwriting the current status of that user because from a analytics perspective, from a human being perspective, you're like, tell me about that user now.
[00:38:45] Oh, this is our current FICO score. This is their age or this is what's going on with them. This is where they work or whatever, but from a machine learning, I wanted to know who they were when they made decision A 10 years ago versus who they are now. Yeah, sure. [00:39:00] Sure. And so one of the interesting things here is, you know, in financial transactions, I'm like, let's stop evaluating the person.
[00:39:08] And let's start evaluating the transaction as it currently is identified. And even you could say the same for recommendations in e commerce. It's like, let's not think about me as an Alex Miele. It's really hard because humans definitely want to think about like, let me think about Al and Betty, right?
[00:39:22] I'm sure the A B test people like, right? Let me tell you, Al is different from Betty. And I want to think about this thing called personas. Yeah. But you know, what machine learning doesn't really care about Al and Betty. Machine learning cares about The representation of Al right now can be, you know, someone who is 32 coming in from this location who happens to identify as male and blah, blah, blah, blah, blah, right?
[00:39:44] Those attributes, we don't actually care that that is Al, right? And that person right now, as associated to those attributes right now, makes the transactions. And three years later, the person coming in who happens to be 45. may look slightly [00:40:00] differently, and even though it is the same person, we don't have to care that it's the same person.
[00:40:04] And if you're, one of the biggest challenges is To kind of change how people sometimes think, because if you want machine learning optimization, you kind of have to think about how the machine learning wants to learn. And it's different than people. And what it can do is pretty fantastic if you just let it fly at those attributes and stop thinking about this the way you would want to think of this, this consciously.
[00:40:29] And it is, it creates a little bit of friction, but eventually people get it. And when they get it, it's just like, A big aha that a computer doesn't have to learn the way we learn. Totally.
[00:40:39] Justin Grammens: Makes sense. I love it. I love it. Yeah. Yeah. Yeah. Yeah. I've heard it thought about what, like we're a carbon based system and all these computers are silicon based systems, I guess.
[00:40:48] They say, Oh, I guess it remains to be seen who's, who's the superior one in a lot of these ways, but we all do things our own way, right? As as carbon humans,
[00:40:57] Alex Muller: you can, you can already see [00:41:00] there are differences. So, and I'm not getting into the whole, you know, ASI, Artificial Superintelligence, I'm like, we as humans cannot process a million rows of data.
[00:41:12] We just can't. We would summarize attributes of that to create kind of higher level, logical buckets, at best 20 of them. Yeah. And that means that we're only going to have so much fidelity when there are a million different records of data with a million different scenarios. Right? Our, our fidelity will cut into those 20, essentially.
[00:41:36] The machine learning one could literally come up with tens of thousands against that million. Or a million against a billion. Like, it is such a different scenario. And with that fidelity, the AI is going to be a little smarter in the high data realms than the humans. And the humans are going to be a lot smarter in the low data [00:42:00] realms.
[00:42:00] And that's where you got to bring those marriage together. You got to, it's like, it's kind of like when you're running, you have a team. Right? You have a member of your software team. Let's talk about software teams, right? I have a UI designer, I have a UI developer, I have a database designer, I have a full stack developer.
[00:42:17] You know, I have these different roles. And I don't take the UI designer and say, Hey, design the database. Right. Yeah. And so once you understand how the AI tool, which is really just a toolkit, fits in the overall tools you have to get your goals accomplished, Then everything becomes easier and you don't think it's human versus AI.
[00:42:38] It's always human with AI. Like, I love the quote that's like, AI won't take your job. Somebody working with AI might. Totally, totally agree. Yep. Yeah,
[00:42:47] Justin Grammens: yeah. And I view it as a companion technology, I guess, that the whole goal is to make us. More efficient and better humans in a lot of ways. Yeah. This has been awesome, Alex.
[00:42:56] Are you guys, well, I guess two kind of final questions, I guess, that I [00:43:00] usually kind of, you know, end off with is like any advice for people that are sort of getting into this space? Are there any sort of books, things that you've read, conferences attended, projects you found interesting, I guess?
[00:43:10] Alex Muller: All right, so I was looking at your question.
[00:43:12] I want to talk about one project I find interesting just because it's fun. Yeah. Because I think one of the questions you had, what are some interesting uses of AI? Like everyone talks about self driving and the generative, but like, if you want something I think is kind of fascinating, AI has gotten awesome at language translation, right?
[00:43:28] Like we can remember five, six years ago where like, if I speak Spanish natively, so When I saw the English Spanish translation, I'm like, Oh, whatever. It's not very good. And it turns out like the big shift was AI, right? That used to be real space translation. And now it's really like, they just translated a whole bunch of things with machine learning because they had the English version and the Spanish version.
[00:43:47] You know, it learned the best translation. Yeah. So the thing I'm like, what's the next AI application? I was starting to hear a couple of podcasts about it. It's inter species communication. I know it's a little out there, but like, [00:44:00] when we translate English to whale song and vice versa, what will we learn?
[00:44:04] Like, that, that, that to me is fascinating. Yeah. And the whole other use of AI that like, that could be just different. Like, what will, like, and I know right now it sounds fanciful, but there's nothing actually stopping it, if that makes sense.
[00:44:20] Justin Grammens: No, no, you're right, there isn't. I mean, I was even thinking about, like, Star Trek The Next Generation.
[00:44:25] I always kind of laugh because these, these, you know, they basically went to other planets. And even just original Star Trek. But yeah, they could communicate with people. Like, everybody spoke English
[00:44:33] Alex Muller: somehow, right? That's what I
[00:44:37] Justin Grammens: mean, of course they have to have that, right? And so it's not too far fetched, I think, at all to be able to, you know, if you can do one languages, why can't you do, uh, and you can probably translate to Klingon right now actually.
[00:44:48] Alex Muller: Yeah. So absolutely. Like right now, it's like the iPhone is essentially universal. The applications that sit on the iPhone can act as a universal tribe and the Android devices too, right? But when we do interspecies, like [00:45:00] that's, you know, because, cause then it also brings Absolutely. The basis of language, it's a lot of like, it's more complicated because it turns out almost all human language have enough commonalities that the translations are starting to work very well.
[00:45:13] I do think when you get into like interspecies communications, like. It seems so crazy, such science fiction, but then, you know, almost everything we're doing, if you went back 50 years ago, already feels like science fiction, right? Like 50 years ago, imagine somebody seeing an iPhone or a cat GPT or, you know, like just, you know, I love it when you take.
[00:45:33] When my grandmother and I put her in the Tesla and I turned on the full self driving and it's just like, holy cow.
[00:45:38] Justin Grammens: Yeah, no, things are advancing so fast. So yeah, I would not be surprised. And the other thing I was going to say was that part of this podcast here, we'll go through and, you know, add liner notes and stuff like that, links to your website and stuff like that.
[00:45:49] I've been looking at through this. There's a lot of interesting videos actually out on YouTube talking about decoding animal communication and listening to Earth's feces. I mean, this project, these projects are already sort of going on right [00:46:00] now.
[00:46:00] Alex Muller: Yeah, they've been going on, but, but they've been going on for a long time in this kind of manual way.
[00:46:04] And they've kind of like, I was listening to a podcast. I don't remember which one, but they're like, they hit a wall until they got into deep learning. And now like, it's like That wall just got obliterated and now all of a sudden they're making all this progress, which is exactly what happened in human to human translation.
[00:46:20] Like it was this really manual, like hundreds of thousands of lines of code thing, the problem that turned into. Neural net solving with only a few lines of code. And, and I think this is fascinating. That's where we're at right now for
[00:46:35] Justin Grammens: sure. That's an awesome project. Yeah, like I say, we'll put some links in the notes to some of these projects that are going on right now today.
[00:46:41] The stuff that you mentioned, links to your website, which leads me to the last question. How can people get ahold of you,
[00:46:46] Alex Muller: Alex? Sure. So granted, interspecies stuff, but I'm really good at recommendations, decisions, predictions, and classifications for financial institutions and insurance and logistics and things like that.
[00:46:59] But you can get a [00:47:00] hold of me through alex. savvai. com. It's savvy spelled with an I, S A V V I A I. com. And then if you just search S A V V I space Alex Mueller, that's without the E M U L L E R on LinkedIn, you'll also find me. And if you're interested in this particular podcast, just if you find me there and just message that you listen to this, I'm much more likely to accept.
[00:47:26] That's
[00:47:26] Justin Grammens: good. That's good. Well, cool. I really appreciate you being on the program today. You know, we, we talked about a lot of different stuff and this is really what I enjoy. This is actually the funnest part of what I do here. Basically interviewing people and kind of going down these interesting paths, you know, outside of the product that they've developed and their history, uh, with AI, it's like just learn so much.
[00:47:46] So I appreciate you humoring me and talking to our audience and enlightening everybody with regards to your product. Well, let's keep in touch, Alex, and wish you nothing but the best.
[00:47:55] Alex Muller: Yeah, coming out west. All right. I definitely will. Take care. [00:48:00]
[00:48:00] AI Voice: You've listened to another episode of the Conversations on Applied AI podcast.
[00:48:05] We hope you are eager to learn more about applying artificial intelligence and deep learning within your organization. You can visit us at AppliedAI. mn to keep up to date on our events and connect with our amazing community. Please don't hesitate to reach out to Justin at AppliedAI. mn if you are interested in participating in a future episode.
[00:48:26] Thank you for listening.