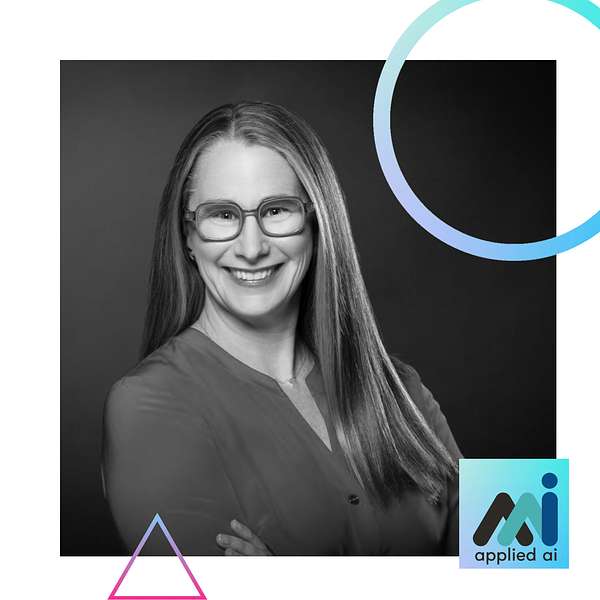
Conversations on Applied AI
Welcome to the Conversations on Applied AI Podcast where Justin Grammens and the team at Emerging Technologies North talk with experts in the fields of Artificial Intelligence and Deep Learning. In each episode, we cut through the hype and dive into how these technologies are being applied to real-world problems today. We hope that you find this episode educational and applicable to your industry and connect with us to learn more about our organization at AppliedAI.MN. Enjoy!
Conversations on Applied AI
Beth Rudden - How Linguistics, Semantics, and Artifacts Improve Our Understanding of AI
The conversation this week is with Beth Rudin. Beth is the CEO of Bast AI and a global executive leader with 20 plus years of IT and data science experience. Previously, she has held roles such as Chief Data Officer, Chief Data Scientist, and Distinguished Engineer. In 2023, she was recognized as one of the 100 most brilliant leaders in AI ethics. At Bast, Beth and the team developed software that allows humans to create their own AI, redefining the experience of the human for our shared future. She believes we have a vested interest in creating AI that can explain itself to humans.
If you are interested in learning about how AI is being applied across multiple industries, be sure to join us at a future AppliedAI Monthly meetup and help support us so we can make future Emerging Technologies North non-profit events!
Resources and Topics Mentioned in this Episode
- Bast AI
- Abstract semantic graph
- Ontology
- Kubernetes Clusters
- Natural language processing
- Structured Query Language
- Imaginable by Jane McGonigal
- AI Superpowers by Kai-fu Lee
- Robin Wall Kimmerer
- The Inmates Are Running the Asylum by Alan Cooper
- AI for the Rest of Us by Phaedra Boinodiris and Beth Rudden
- The Information by James Gleick
- The Overstory by Richard Powers
Enjoy!
Your host,
Justin Grammens
[00:00:00] Justin Grammens: Greetings, Applied AI Podcast listeners. This is Justin Grammens, your host of the Conversations on Applied AI Podcast. Just dropping in to let you know about a very special event we have coming up on Friday, November 10th. It's the Fall 2023 Applied AI Conference. You can learn more by going to AppliedAIConf. com. This full day, in person conference is the only and largest artificial intelligence conference held in the Upper Midwest. It will be in Minneapolis, Minnesota on November 10th. We will have more than 20 speakers with two tracks covering everything from AI, business applications, chat GPT, computer vision, machine learning, and so much more. And for being a listener to this podcast, use the promo code of podcast when purchasing your ticket for a 20 percent discount. So here are the details. Go to AppliedAIConf. com, that's AppliedAIConf. com to see the full schedule and register for the only and largest artificial intelligence conference in the upper Midwest on November 10th.
And don't forget to use the promo code of podcast when checking out to receive your discount. We look forward to seeing you there. And thank you so much for listening. And now on with this episode.
[00:01:02] Beth Rudden: I always tell people this, a career is something that you look back on. It's not necessarily something that you plan.
And when you can look back after 20 years and go, Oh my goodness, I'm now using linguistics and semantics and, you know, understanding where artifacts come from. It's a whole different ballgame.
Welcome to the Conversations on Applied AI podcast where Justin Grammens and the team at Emerging Technologies North talk with experts in the fields of artificial intelligence and deep learning. In each episode, we cut through the hype and dive into how these technologies are being applied to real world problems today. We hope that you find this episode educational and applicable to your industry and connect with us to learn more about our organization at appliedai. mn. Enjoy!
[00:01:53] Justin Grammens: Welcome, everyone, to the Conversations on Applied AI podcast.
Today, we are talking with Beth Rudin. Beth is the CEO of Bast AI and a global executive leader with 20 plus years of IT and data science experience. Previously, she has held roles such as Chief Data Officer, Chief Data Scientist, and Distinguished Engineer. In 2023, she was recognized as one of the 100 most brilliant leaders in AI ethics.
At BASC, her and the team developed software that allows humans to create their own AI, redefining the experience of the human for our shared future. She believes we have a vested interest in creating AI that can explain itself to humans. Thank you, Beth, for being on the program today.
[00:02:28] Beth Rudden: Thank you so much for having me, Justin.
[00:02:30] Justin Grammens: All right, excellent. Well, with the intros out of the way, at least kind of giving a little bit of a highlight of where you're at today. What I like to typically ask is, is, you know, how did you get to where you are today? What was sort of your path? And I know you don't have a degree in computer science per se, so maybe you can sort of walk us through how you got to kind of the trajectory of your career.
[00:02:48] Beth Rudden: So in 1978, I was living in Lakeland, Florida, and I was digging up my backyard sod and I found what I thought was like the most amazing thing because there was this marble cache that I found, but it was just what people had done in order to lay the tiles in the bathroom. But, you know, I like to kind of tell that story because digging in the dirt is something that.
You know, I come by very honestly and I did my undergrad in Greek and Latin and really got into linguistics and, and understanding where things come from, where words come from, was an archeologist and then did my graduate work in Denver. The archeology is something I always tell people this, a career is something that you look.
back on. It's not necessarily something that you plan. And when you can look back after 20 years and go, Oh my goodness, I'm now using linguistics and semantics and, you know, understanding where artifacts come from, it's a whole different ballgame. So what I really try to You know, get people to think about is when somebody puts an artifact on the ground for a specific reason, or it gets buried in strata where, you know, years and years and years when we were digging in Italy, we would blow through 15th century Lombardy to get to fifth century BCE stuff.
And you would just go deeper and deeper and deeper because the dirt gets packed on top. So it's really important to understand where the data comes from or where the artifact comes from, what strata it is, what is it correlated with, you know, what did people do all day from that culture at that time, what kind of memes, tropes, and mores did they have, what kind of things did they wear, what kind of music did they have, and it's through all of these correlations that the archaeologist really builds the picture of why did that artifact get into that strata at that time.
And, you know, after Selling out. I was a programmer. I was an information architect. I was a engineer. I was a data engineer. I loved visualization. I think visualization is one of the most amazing ways to be able to show data and information. You know, I was thinking back all this time about the archaeologist and I used to have this joke.
That's probably not so funny, but you know, it just gets people to really understand. We don't know or anytime we need to say, why did this person put this artifact in the ground? We're accessing our creativity. We're making up a story. And the joke was, well, oh, obviously it must've been religious. It was so far back.
We don't really know. Right. But when you're doing that with data and my conceptual model of data starts with a very, you know, very intentional. definition of data in that it is an artifact of human experience. Somebody put that data there or created the data in the system or created the system to create the data.
And when you think about how an archaeologist had to make all of these choices to be able to construct the story, that archaeologist is infusing their bias into that story. And we have tons of examples of this where in the 1800s when The proverbial white man was running around to Papua New Guinea and running around the world saying, well, Oh, this must've been the king or this must've been, you know, whatever their culture is.
Exactly. And so when I look at, and I do a lot of work with data systems and have. pretty much all my life. I, you know, built databases for shards and sherds and then really love the idea of like, you know, cataloging and classifying. But when you're doing that, you're making a choice on what that context is for that data.
And so fast forward all the way to today, where we have large language models that are scraping huge amounts of data And divorcing that data from the context, and then regurgitating that data into a different context, it really reminds me of the Tower of Babel. And, you know, the story that God came down and mixed up all of the languages because they built a tower that was too high to the heavens.
And it's very interesting to me because I think that If we start to think about data as an artifact of human experience, and data is personal, like, you know, if you're having a bad day, and you're filling out a form, you're putting that data in a bad way, right? Or if you're having, you know, you just got really, really good news, then maybe you're like writing all this stuff.
I actually was on the phone with a support today. And I was arguing with support about how their algorithms were Not very specific. They're very sensitive, but not very specific. Anyway, I digress. But what I want people to think about is that the promise of AI is hyper personalization, hyper personalization, where we can all have an understanding of how to use AI like we use our GPS.
It's our pocket brain. It can know when I say, Oh, hey, you know, I'm thinking about what I did yesterday with that thingy thing, and the AI will understand that. Because it understands the context in which I personally was thinking about the thingy thing, and it will be able to interpret that. But until we get to human beings that understand how to take ownership and understand how their own data artifacts are persisting, it's going to be very difficult to get to that promise of AI that is hyper personalization.
And right now I have a prediction that I think that not even in five or 10 years, people look back and say, you used an artificial intelligence that couldn't tell you what evidence it used to give you the prediction. How did you guys do that? Like, why, why would you do that? That sounds so silly. Have you ever heard?
There's no hand waving in math. Yes. It's mathematics. It's statistics. Yes, exactly. If you don't provide evidence, if you're not providing lineage and provenance of your prediction, you're not really using that to make it accessible by other human beings. And that's one of my main goals, is I want to see the promise of AI, which is hyper personalization.
And I want people to understand that they should be participating in this AI revolution. By understanding... What data, what information is out there that they are putting out there? What are they exhausting? If they're a customer service desk agent and they're putting in tickets the way that they're putting those tickets in, that completely changes that data in the interpretation of that data and We do really silly things like the people who put in the data are not the people who analyze the data and the people who analyze the data and then put it into training and test sets for models or scraping it from the internet are not the people who would be using the output of the model.
Yes. It's like playing a game of telephone. It's absolute silliness. Yes. So that's where I'm at. I know that was like kind of a long, uh, windy story. But. If we are going to get to a representative sample of humanity, 8 billion people, we need 1. 6 billion humans who are AI literate. And we are nowhere near that.
Nowhere near it. Yeah. And
[00:10:20] Justin Grammens: is that what you're helping at BAST in some ways to do? To make people more literate about artificial intelligence and the data that they have?
[00:10:28] Beth Rudden: We are building a platform where we can allow people to push their own data in. And we use really old school semantic graph technology called ontologies in order to extract the entities and relationships.
to ground the data and provide the source for those entities and relationships. So for instance, if you had your grandfather's journals of like, great, great, great grandfather's journals of the 1800s and you wanted to understand them. That's a different language that they used in the 1800s, different idioms, different language entirely.
So if you took those journals and you grounded the language with a graph model, and then you built a language model of the common phrases and the language that they used in the 1800s, You could then interpret that, those journals against the language of the 1800s. If you try to do it against the language of today, you're going to miss a lot of those signals.
Because it's not going to be interpretable against a context of today. It's going to completely change. So if you want to do something... Well, you really need to have the ability to, to ground the context by which you use to understand the information. There's no understanding if you don't know what perspective to understand it against.
Am I talking about a jumper that I'm wearing? a piece of clothing because I'm from Britain, or am I talking about a boot of a car because I'm from Britain? So it's so different, and context matters, and this type of specificity is really difficult for machines to do. Machines do, you know, sensitivity very well.
They do the ability to see large scale patterns and predict the next sentence shape word, but You know, in reality, language is far more subtle, and when we are speaking and we're giving language, we're giving off our educational, where we are in our education, we're probably giving off our political association, our sexual association, our, you know, our culture about what we believe, what we think, and that's something That, you know, using ontologies and using graphs, it is the, the nature of your reality based on the language you use.
So when you're grounding that reality, then you can see things against that particular reality, or you can see things against other people's realities or other people's knowledge graphs. And where we're applying this first is so super cool, and I, I'm like blown away by being able to get. To do this, we have an enterprise partner that we're working with Simworks and they are ex special forces.
They found me after a podcast that I did and said, we need your technology. And what we're doing is we're grounding protocols for Rangers or for special forces people in theater in order to have a medic co pilot. And the very first principle that I use in all artificial intelligence is all AI augments a human.
So you better have a human being that you're going to augment. And how we want to augment the medic is we want to reduce the cognitive load. So if you're dealing with multiple patients with multiple injuries after four hours, that medic is fried. And so if the medic. can go to our cat, which we call our product conversational AI technology, a cat to really disassociate it from a bot because it's so much more.
The cat and the medic goes, Hey, what do I do with penetrating chest wound? And the cat pulls out the information from the process flow or the protocol that that medic is trained on. To just reduce the cognitive load and be able to give the medic an understanding that they are pulling directly from the approved protocol and give them the assurance that it's fully auditable and this ability to pull out like the next step in something in a process, it's a great way to augment people because we're not even thinking about it.
We're still thinking about like, Well, a lot of other people are thinking about how can I take all the data in order to be able to build the model to make the money on the model? That's not what we're doing. What we need to do is think like how a human being use... Do you remember the old GPS's? Like Tom Toms?
Like you had to look up, follow the Tom Tom.
[00:15:24] Justin Grammens: Yeah, right. Yeah. You might take you in the lake. That's right.
[00:15:27] Beth Rudden: Exactly. So I'm like, where we are with AI or where I see it going is not the right place where we should be going is we should be thinking about how do we augment people and enable them to work with.
The artificial intelligence so that they can do a better job. So the other piece that I really want to point out was what we're doing with our technology is because we. For two reasons because we have intent and we know exactly how we're training our model so we can take the model and say okay Here's a medic in Iraq.
Here's a medic in Afghanistan So it's specific it's again specific to that context and we ground to that particular context and Then we do a lot of search and retrieve to make sure that we're really auditable and we have full precision in that sense. We do it so efficiently that we can deploy it to a smartphone that does not need internet connectivity.
And then we train it so effectively because we understand the software engineering on how to use Kubernetes clusters and cloud services in order to really put it into a very
And what a lot of people are not thinking about is when you are training a model on a specific set of data that you understand exactly the intent that you use to augment that person, would you like to pay 100, 000 a month for your AWS bill? Or do you want to pay 100? And why are you using all of that compute for something that doesn't have An actual reason.
So it's that intent that we really are looking for as well. Wow.
[00:17:19] Justin Grammens: A lot of, a lot of thoughts here. I've been notes. I've been jotting down here as you've been, you've been talking. Cause I mean, a lot of it does come down to transparency, you know, and the feeling that whatever this AI says, we're just going to sort of take for truth.
And I was actually attending an event that had a panel yesterday and, you know, people seem to sort of poo poo away this whole idea. I mean, it came up at the beginning, but this whole idea of hallucinations, right? And where today, I think, I believe, you know, large language models and chat GPT and stuff like that are, are very good at just getting information out on the page that to help you sort of think through other ideas, be creative, you know, maybe rewrite your stuff to make it sound more, more real, but it's, it's terrible when it comes to Logic and math, that's been shown.
You just ask it to add some numbers, multiply large numbers, it's wrong. But it sounds like it's right. And the other thing is, like, a lot of research, too. You know, you ask about case studies and stuff like that, and it'll rattle off stuff left and right, and then when you go and try and actually do the research, you realize that...
Those URLs don't even exist or, you know, there, there's really no proof sort of what's going on underneath the cover. So it's still on us, the humans to sort of fact check this stuff, right? And I guess it feels like that there's two ways to do it. One is you get the information and then you as the human have to go back and fact check it all, which sounds like a lot of work or the solution that you're providing.
And, I
[00:18:42] Beth Rudden: mean, I usually teach that there are three components to NLP. And I've been building natural language processing models. Things that stem words or take the lemma to understand the morphological understanding of the word within the sentence. Or to do, um, tokens. Tokenization is a, A big thing that people will listen to when you're building large language models, just minor consideration, people's whose heads are full of linear algebra often don't have linguistics or semantics in their background.
Yes. So, I mean, there's, there's a lot of, you know, interoperability that may or may not be there. And I don't think you need large language models, but I do think you do need to understand the aspects of a language model. So when we were building out and we have our own code, but we also look at what's out there and say, okay, well, we'll pull from spacey today because their tokenization process is better than ours and then do an evaluation or whatever.
So the very first thing that NLP in natural language processing has three aspects. So you have natural language understanding. Natural language classification, which is your prediction, and then natural language generation. Generative AI is really great at generating variation. And generating those transformations, like really, really, really great.
It's not so good at classification within context, because it has no context to classify against. And it really sucks at understanding. Because it has no context to understand against. So, what we do is we extend the generative model. And we use the generative model to generate all the transformations, all the fluff in between the words.
That's a great use of it. Back in the day, when we were, like, creating conversational AI, we would hand, like, 10 questions to the IT group and say, give me a hundred variations of these. The IT group who had no domain expertise. And so it's like, it would come out where I was like, Oh my gosh, you gave it to India.
They're like, how'd you know? And I was like, look at the language. You know, they use the word kindly a lot, which I think is beautiful. But like, you know, you can tell. And that's where I think that people aren't thinking about this quite effectively in how we can practically use these generative models.
To really accelerate the ability to build out these specific models, use it to augment your process. And, you know, the sensitivity versus specificity, this comes from a data scientist or, you know, somebody who's out there all the time talking about this. And she says, human beings are great at understanding specific logic and specific instances and specific contexts.
And what I'm trying to do is I'm trying to use that to get people to think about the contextual awareness so that we can again get back to that promise of AI. It's personal. How do I personally use this? And on my website, I have various examples. All of those examples fail when you try to go to chat GPT because it doesn't have context.
A nurse goes, Hey, I'm going to put a 24 gauge needle into an adult. Chat GPT says, Great, wash your hands first. Right? Instead of, um, that's only for an infant. Sure. Sure. You need to use the right gauge needle against specificity or somebody's grandmother was told by their, you know, AI doctor. Oh, hey, you should reduce your sodium.
She's like, I don't use salt. No, grandma, stop using soy sauce and stop using like, you know, fish sauce. So again, specificity. And this is where I don't see enough people thinking about that. So it's not just about providing evidence. You know, it's not just about providing your source. Providing your source allows you to understand that somebody is doing the job to listen to you, listen to your data, understand your personal need.
And that to me is, is the reciprocity that we've been missing in all of these different technologies where they're owned by corporations and they're created by homogeneous. You know, groups of people, we need diversity big
[00:23:06] Justin Grammens: time. Do you see then a world, and again, I've heard a lot of people in this space and I completely believe it, you know, in the coming years, we're going to have our own personal assistant, right?
That's right. Ask it, we're gonna ask you questions, book me meetings, you know, respond to these emails, all that sort of stuff. You can have this assistant, but. You know, where you're coming from. I mean, so does everyone have their own sort of trained ontology is probably what you're
[00:23:28] Beth Rudden: looking at. Yeah, we, we build ontologies behind the firewall.
Enterprise is our first focus. We're in the middle of a raise that's going very well. And I really think that that's the place to do it because you need the money in order to be able to scale where I think we're going next is you mentioned like, you know, schedule your meeting, you know, respond to your email.
What I found, and we've been doing a lot of work with nurses, and that's like my next thing, where we want to get nurses in education because they're doing this in China today. Everybody in China, medical students walk in, they get their own AI assistant, and the assistant does things like schedule their meetings, you know, do their emails and things like that.
Take their notes, yeah. Take their notes, ask them questions, so I think we need to do this in America, and I'm working with Maryville University in St. Louis. Actually, it's, it's a fantastic business. We increase their educational product. They increase enrollment, nurses get access to AI. But what we found is that you need to have, you know, that, that 100 percent certainty.
But we have our AI system also allows them to have some coaching for some mental health language. Like what's the difference between depression and acute stress reaction, right? Like a specific instance versus a, um, something that is more long term. Or my kids are scheduled, or my kids need some activities for the summer.
How can I take their schedule and match it with my nursing schedule? Those are the things that humans need. And that's what my mother was asking me. She's like, you know, how do I interpret this flood insurance policy? You know, that's where I'm trying to give people the understanding of how it works. And then where we're going with our platform is mom can upload her insurance policy and have a conversation with it.
Nurses can upload a coaching book to understand the six habits of coaching that they can use in order to Help themselves or help other people. It's this using validated sourced information to augment their, again, personal knowledge graph of how they're understanding what they're doing. And it's not just about scheduling and emails.
Those are things that we do in the digital world as part of our work, but think bigger and broader about the things that you could probably do. So much better if you could have it relayed to you in your language so you can have a conversation with any unstructured document. Yeah.
[00:26:09] Justin Grammens: Well, yeah, yeah, yeah, for sure.
And there are a number of plugins actually that OpenAI has that allow you to actually push documents in and then start asking questions about it. Just basically fine tuning sort of the model now. You know, I wouldn't ever say to anybody that they should ignore what their psychologist says and then just start talking to Chad GPT out of the box.
But I feel like there are some areas with regards to this document you're talking about, like, Hey, I got this, this real estate document. Like, isn't it plausible that somebody could just use Chad GPT to sort of push this document in and ask questions sort of like out of the box and in a very sort of small, small use case.
Right.
[00:26:45] Beth Rudden: But they wouldn't be able to do it against your context. Right. And one of the things that drove me, many things drove me. So I left IBM after a 22 year career and a lot of executives leave organizations, start their own business because it's very difficult to innovate in a large corporation. You have the innovators dilemma after a pop.
And many organizations, I think Salesforce is probably the most avert about it where they shove their executives out
[00:27:14] Justin Grammens: of the nest.
[00:27:15] Beth Rudden: Yeah. But you know, the thing that really drove me was that our healthcare system is so poor and my sister is very sick, undiagnosed, autoimmune and in and out of hospitals.
And I would sit in the hospital with her. And because of what I do is I would take all of her data, line it up, show it to doctors, show it to the nurses. And they're like, Holy crap, it's on a timeline. That's so much easier to consume. You're right, she should be off this medication and on to this one. And it wasn't that I showed her anything else other than putting the data in a way that the nurse, the domain expert, could interpret it and understand it and see it because my sister's case history is so long.
Just putting it on a timeline. Worked really well having a grass model where sister can like interoperate with her medical records One of the things that we do is we meditate every single day and sometimes when she's having a bad day I remind her and like it's only been two days You know, you, you were okay last week.
That type of augmentation, I think, is what we should be thinking about using AI for. And it should be personalized. Do you see?
[00:28:27] Justin Grammens: Yeah, yeah, yeah, yeah, absolutely. And, and I love the, I love the summarization idea. You know, it really kind of brings back to your idea of data visualization. Right. It's kind of funny, kind of went full circle.
You said you love data visualization at the very onset of this conversation. And it feels like in some ways, kind of what you're doing, you're getting all the information out so people then can make better decisions, at least in that particular case, you know, in that, in that one use case. Well,
[00:28:51] Beth Rudden: graphs are very visual.
And, you know, growing up being just highly technical for an instance, like SQL or structural query language is based on set theory. And it's, it's very structural where it's like you ask a question and then you have a predicate of like, you know, what kind of question or what kind of filter do you want from this data set?
Then we went into not only SQL databases, which is both, you know, columnar as well as row, and you can do big data lakes and stick lots of unstructured data in. Graph models changed my brain. It's like n dimensionality of understanding that one word, it's a, it's an open world view where one word can have many different meanings.
depending on context. So, in these protocols, like, we know that the next best action is to apply pain management. In one protocol it could be fentanyl, in another protocol it could be morphine. Those are things that we can do very simply because a graph can handle lots of metadata. So that you can have that specificity for that contextual understanding.
For that specific protocol, this is what I see is the big future is like probabilistic graph modeling, because we're really thinking through the end dimensionality and using the machine for the sensitivity and then using the human for the specificity. I
[00:30:18] Justin Grammens: like that. I like that. Yeah, yeah, yeah. I mean, the human is the person understands the domain kind of bring it back into the domain expert.
They're, they're the ones who are going to know exactly. How this can actually be applied. So,
[00:30:30] Beth Rudden: one of the people I follow is Jane McGonigal. She wrote a book called Imagineable. And she would know, she does lots of future simulations, but we're probably looking at 1 or 2. 6 billion climate refugees by 2050.
And I believe very strongly that the cure always grows next to the cause. And if we think about why we need to use AI right now and get the maximum amount of people using AI right now, it's because this world that we are borrowing from our children. Our children will be faced, the next generation will be faced with things that we can't even imagine.
So how do we make them the most powerful, the most powerful humans on earth? And that's something that I see every single day where the ability to extract the tacit information from the aging knowledge workers and make that explicit in a conversation. That's where in America, we haven't made things in 20 years and we're losing that tacit experiential information.
I want to run around and like extract from all of the old plumbers and the people who can build these lathes and these, like the, the things with their hands, how do we get that information? To that next generation at a time that they desperately need it and not to get biblical. But I'm like, we need an arc.
We need an arc of knowledge, of knowledge, graphs of, of understanding these things. And it's not to control or to make money. It's because there's so much necessity that I see coming with. All of the challenges and all of the problems of not only globalization, but climate refugees, where people are going to go and try to figure out how to subsist again.
And that's, that's a massive thing that we have coming our way. How are we going to deal with that? Right.
[00:32:30] Justin Grammens: Yeah. Yeah. And when you say refugees, yeah, there's just literally places on the earth where Humans just can't live anymore. So they're moving into different climates now that are, that don't go through a lot of these crazy seasonal things.
And I'm a hundred percent agree with you. I mean, and I think anyone could think about the climate when they were younger and think about today and just how it's changed. I mean, I'm, I'm in Minnesota and it's just, we don't get nearly as much snow that we did in the past. We get much less. It's not as cold people will point to say, well, yeah, but you know, yes, there are spikes where it gets cold for sure, but nowhere near what I imagined was a little of what I remember and Frank, as I, me imagining it, it's the data, right?
Climate data is showing this. So in order for us to do this, I'm a hundred percent behind you. Like we need this technology, whether it's AI or I deal a lot with sensors and internet of things and data around that. We need to make sure that we have the best tools possible because yeah, it's, it's not going to get any.
easier. Do you feel like the U. S. is behind? You know, you mentioned China and globalization, stuff like that. Do you feel like we're sort of behind in this game, right?
[00:33:30] Beth Rudden: Yeah. Kai Fu Lee is a Apple executive. He wrote AI Superpowers and he talks about what they're doing in China. And this was written in like 2018.
And then in what he says, I think is correct, where AI and the Americans really paved the way for discovery. But if you want to talk about implementation, it's China and they are implementing and executing in ways that were hampered by and I was at an AI medical conference and a professor was talking about, um, in Shenzhen, they are building huge facilities in order to train nurses, medical doctors, you know, people who have this, this hands on experience because I think they see the same thing that everybody else sees.
There's a huge necessity for caregivers and caregiving cannot ever be automated in any way, it's a very human, human thing. So what he says is we have these facilities that we're, we're dumping billions of dollars in. And I know when I was at, I, IBM, I did a lot of research studies and we interviewed 2, 500 people, 16 levels of management, and we could put people into four quadrants, teams, countries, um, anybody.
People apply AI myopically, just do it so I can get my budget. It's a, it's a sexy word. Uh, they can apply it risk based. I'm in a, you know, risk based, I'm going to do the minimum so that I will be auditable and can pass my, you know, pass. The audit strategic or opportunistic, which is where I like to tell people, if you explain what the model's doing, you can get more adoption quicker.
You, your, your change management goes like that and you can debug it. It's so much easier if you know what to do. And there was only one country that is really in strategic and that's China because nobody builds AI models without explaining what it does to the government. They don't have that type of competitive.
They have the central force that says we're going to push it out everywhere. They have a very different viewpoint on data and privacy and individuality. And this is why I'm like, America, wake up. We've got to get to the execution, and we're way far behind because we're debating on what it is, instead of how do we use this safely, how do we put this in play in a way that really amplifies the very people who need amplification right now, the caregivers, the first line responders, like the people who are Who are doing the human work, how do we get it to them first?
[00:36:07] Justin Grammens: Gotcha. Gotcha. Yeah. You're enabling, I guess, all these various personalization aspects to have, and it sounds like healthcare and I said, enterprise is kind of the first place to start cause that's kind of where the money is, but that will help you fund it then all these other areas and. You know, you could see this being used, you know, in rural areas in Africa in the future and all this type of stuff, but it seems very, very interesting specifically around how humans are going to interact with this.
So how do you change? I mean, you had said earlier that, which I 100 percent agree with, like, you always want to have a human there because. These are, should be assistance to humans. Are you at all afraid, or worried, or thinking about, you know, okay, 2050, 2060, stuff like that, where we completely do automate stuff.
Do we think we can build systems that, in some ways, people talk about, you know, the human race? It's kind of the, I guess, the lesser when these AIs get so good.
[00:36:56] Beth Rudden: Am I afraid? No, because that would be interesting. You just don't believe it'll happen? No, no, I don't believe that. So not in this current frame. So it's never one thing.
And a lot of the work that I do is I combine semantics and statistics. So I do, I do have a pretty strong point of view, especially on things like causal inference and, uh, spreading activation and some conceptual hierarchy on how humans learn. Is still very different than a computer and you know, the, the statistical models, this is a distraction, this entire conversation about, you know, the existential threat of an AI superpower.
It has nothing to do with the machine having agency and, you know, becoming conscious. I would ask people who does it benefit for people to believe that AI can only be done with large compute and large data. Who does that benefit? And then we're back to the fact that we know through proxies and through a lot of people doing really hard work and getting fired for doing it.
Like an average large language model training cycle takes all of the electricity in New York City for one month to run. Or 15 swimming pools full of fresh water. Like, this is insane. Yeah. And there's no intent, by the way. What was the user, who was the user in mind when they created these models? Why did they take so much without giving back?
So there's some really common rules of nature that Every single human on earth has to abide by in one way, shape, or form or another, because we're part of this ecosystem. So when you start thinking that humanity is not directly tied and connected to the ecosystem in which we are acting, I think that that's where you're, you're going wrong and you're, you're going down a rabbit hole for.
Potentially a, a reason where people might have outraged you to keep you engaged. . .
[00:39:04] Justin Grammens: That's a good, good way to be, say that. Yeah. Yeah.
[00:39:07] Beth Rudden: And, and so I would ask people to maybe consider, how do they know they're really angry about this? Or how do they know they're really afraid about this? Like, really like, just ask, ask yourself some of those questions.
And what do you think about like, you know, maybe if we built models that fit form to function, that used reciprocity than only use the compute that was needed in order to perform an action that is equally necessary. So, you know, when we look at like photosynthesis, we still don't even know how it works.
It's like the most efficient process in nature. So I'm a huge fan of biomimicry, if you couldn't tell where it's like, we can look at these models that we see all around us and make things. So much more effective and an author I follow religiously is Robin Wall Kimmerer. She's actually a botanist as well as a citizen of the Potawatomi nation.
And she combines indigenous knowledge with, you know, Western science and. You know, what she is really thinking about and, you know, through some of her work, I would love to pull that into the, you know, computer science fields, because the way that we can engineer things, I know the way that my engineers work through how to distribute load on a Kubernetes cluster in order to make it more effective.
That could be the same thing on, you know, how bees perform their function within hives. So we have these, these like swarm theories, you know, things that are out there. And I think that we, we really have to start thinking about building those multidisciplinary teams, people who are coming from all over with different ideas.
We have to start building like this, this open mindset versus a fixed mindset and really, how do we pull in all of these things? How do we build this world so we can leave it a little bit better than we got here and a heck of a lot better for our future generation?
[00:41:09] Justin Grammens: Yeah, absolutely. Yeah, that whole idea of, you know, nature is probably the most efficient thing on earth.
It kind of has to be in order to survive, right? So how can we look around and see what's going on in our nature and sort of. Map that and with regards to like, we still don't know how the human brain works, right? I mean, we're just we're trying to model it. We're
[00:41:28] Beth Rudden: cave drawing like we're cave drawing with sticks.
We have no idea what we're doing Yeah, so much left to discover and this is where I get so excited because I mean There's a couple ways that I talk about this But you know, there's seven to eleven ways that we communicate What are the names of those? We don't know yet. We haven't named them just like gravity before Newton.
We didn't have a name for it. AI can help us discover this hyper personalization, knowing that you live in the Midwest, I can correlate new information to you using that knowledge, using something that's a part of your existing mental model and reduce your period of disequilibrium. I can make you learn faster by correlating new information to your existing mental model.
We know we can make people learn faster. We're not doing anything about that. We should be. Because again, I think this next generation, what are they going to have to solve? How are they going to do it? How are we going to build infrastructure? How are we going to, you know, get past all of these distractions?
Because if we're going to go down to like a de civilized subsistence level, how do we help? Everyone in, in those areas. Have you ever heard of a book called The Inmates Are Running the Asylum?
[00:42:50] Justin Grammens: I've heard the book, but I've heard the term. Absolutely. Yeah,
[00:42:53] Beth Rudden: it's actually, it's a fantastically researched book, and in there they say that 7 percent of any organic ecosystem can create change 7%.
I find that fascinating because if you think about humanity as an organic ecosystem, how do we enable that 7 percent to make the changes that are necessary to stabilize the ecosystem for the future?
[00:43:17] Justin Grammens: Right, right. Yeah, yeah, yeah. And, uh, where my mind was going, you just, you kind of like reminded me of a prior podcast that I had with a woman named Amelia Winger Bearskin.
Her focus was, and this is just for people that are listening to this podcast as well, is she talked a lot about using empathy to create responsible technology. So it's this idea of basically, you know, you can do harm to systems without actually understanding what you're doing until you understand them and bring sort of the end to the end of the conversation.
I was going to let you know that, you know, all the, all these books that you're bringing and all this sort of stuff, these are, these are awesome. And we'll make sure that we put those as links in the transcript and in the liner notes as well, because we've covered so much here. And one of the things that I love to do is to have our listeners sort of get a bunch of different perspectives and then I'll pick up some of these books and read them.
Then be able to apply them because, you know, they, they may not be the people training the model. I mean, I've got such a huge array of people that are just not even in the data science field. They're just kind of curious and trying to see where things are going. And there's two sides of this question.
One is maybe I'm just coming out of school, for example. So sort of cast back when maybe you and I got out of school, what are some things that they should be exploring or, or conferences they should be attending or books or anything like that? Maybe areas. That you might give some sage advice to, and then again, maybe others who are just sort of just entering the field, right?
Maybe they're not even out of school. Maybe they're in their fifties and they're like, huh, this AI thing, I'm reading about it all the time. Where are some areas that I can kind of get more information?
[00:44:40] Beth Rudden: So I have written a book. So I'll give you that one. It's called AI for the rest of us. And my coauthor and I, Thedra Boinaderas really put forth because we want people to understand there's an alternate narrative to AI, but.
There's two books that I almost always recommend. The first one is called The Information by James Gleick. And that is a history of information all the way back to Ada Lovelace. You know, she's Byron's daughter. She's a poet and you know, she understands the poetry of mathematics. And that's also just, it's a history of flood, a deluge by James Gleick.
And then the second one, I was, I was around when we, or when IBM acquired Red Hat. And I got to know the chief of staff for Dan Whitehurst, and he was very interested in, in climate change as well as sustainability. And this is a book that I recommended for him because it talks about AI in a way that I think everybody should start to understand, and it's probably one of the most.
Beautiful books that I've read in the last decade. It's called the overstory by Richard Powers. And it talks about trees and it talks about the nature of time, you know, to trees and then how the nature of connections and the network that trees have. And there's lots of fantastic stories woven in between, but that is, that is my huge recommendation to people of all walks of life because it's just a beautifully written book and a beautiful set of stories.
[00:46:10] Justin Grammens: That's awesome. For sure. Well, great. Great. How do people get a hold of you, Beth?
[00:46:15] Beth Rudden: LinkedIn, Beth at bass. ai. Connect with me. I'd love to know what people think. I'd love to know what you want. Like, how do we, how do we use AI? Like once, once my platform starts getting launched, I really want, as you rightly said, I want to get this to, you know, teams and people and nonprofits.
And I really want to help enable and that 1. 6 billion people. I really want to see that happen because we don't have enough diverse. Thinking humans or diverse neocortexes participating in this AI revolution. And it matters when the first Alexa came out, it didn't recognize my voice because there weren't enough female engineers.
This is stupid. Simple. We need diverse. Perspectives working on AI, the designers that would walk in the room going, I don't know what stochastic means, but what you're trying to do here is wrong. You have so much more of a great output when you combine people, interdisciplinary, multidisciplinary people from all walks of life.
We need that. That's what I want people to know is that everyone is part of this AI revolution. Everyone.
[00:47:33] Justin Grammens: Yeah, for sure. You know, I didn't know what Bast, you know, meant. I actually kind of had to look it up, right? Is it, it has to do with plants and like the, the fibrous nature of them. So you're kind of rooted in nature in some ways.
[00:47:43] Beth Rudden: That's interesting. Um, actually it's after the Egyptian cat goddess, which is why, we build cat. But yes, Bast is also the fibrous plant. Thank you for that reference. That's
[00:47:55] Justin Grammens: pretty cool. Well, I was looking, I, you know, I was looking at your website. You basically have a plant there. And so, so people can go there and there's a button to sort of book a, book a demo with you.
Right. You, you welcome anybody to come in and set up some time and then you kind of walk them through the platform. And it feels like, yeah, I mean, I mean, it sounds like you're looking for more data and more applications. I guess being a good CEO, which, which we didn't even talk much about that. I mean, I don't know if you have a fair amount of time, but you know, to, just to maybe go down that thread a little bit, you worked in this large organization and then all of a sudden.
You started your own business and now you're the CEO and frankly, you're, you're a woman in data and also a business leader and CEO, which is, you know, it's unfortunate, but there's not enough of you around, right? There, there's, it's usually a male, white male dominated type of space. And I'm super, super happy to interview people that are not that, right?
That have gone and sort of like done this. I should've started with this question now that I'm thinking about it now that we're actually, you know, 52 minutes in, but how's that journey been a little bit? If you have a, if you have a couple more minutes to talk about that. It's
[00:48:55] Beth Rudden: thrilling. It's absolutely, you know, a friend of mine told me years ago, she goes, the perfect job is something that scares the living, you know, crap out of you, 50 percent of the time and something that you can do in your sleep, 50 percent of the time.
And I was a very good technical executive in IBM. I built businesses. I grew businesses, but that was internal. And, and I had tons of help where, you know, I had offering leaders and my go to market materials was, you know, created for me. I used to do delivery enablement and sales enablement. So I'm very used to doing this at scale.
And I think that. You know, when you're out in the startup world in the startup landscape, one thing I'm noticing is a lot of my connections in the business world typically have connections with government and with education for pipeline, but the startup in the VC culture do not seem to at all. It's like two different worlds.
And, you know, what I would say is like, as an experienced business woman in. in growing AI and I have created large amounts of ROI for my clients and customers at IBM. I would say that we need to bridge that gap because we need more entrepreneurs, but we need more entrepreneurs that are connected to the community of education and government and connected to the communities of how business really works.
Business is relationship business 100 percent. It's all about Making sure that you are delivering on your brand and your relationships, and they are only as good as the relationships that you can support and maintain. And that's where I think we have. An imbalance right now where we're putting a large amount of money into the hands of people who are not holding accountable for what they're doing with that.
And that's like a big, huge recipe for disaster in my book. So if I handed my 19 year old 1, 000, 000 and said, go wild. I mean, I would not be very pleased with what he probably wouldn't do with it. Sure, sure. So I think it's a maturity. I love being mature. I love being experienced, but I also I'm learning a whole new language with venture capitalism and I love learning.
So it's fascinating. Do you hear me on the bridge? How do we how do we bridge that gap? They feel like two totally different worlds and they shouldn't where we should have economies of scale where we are including a lot of the startups into more of the seated mature business.
[00:51:29] Justin Grammens: Yeah, and to me it might just feel like risk mitigation, you know, where a lot of these large enterprises just ready to take that kind of risk because it's so driven by profits more than by, by doing good to the world.
Does that make sense?
[00:51:44] Beth Rudden: Well, absolutely. And those that are applying AI myopically or in risk, you know, just for risk mitigation, I think they're going to be left behind. You know, there is a first mover principle here. So, you know, the, the companies that start measuring the man plus the machine, those are the companies that will win.
Just like, you know, an AI is not going to take your job, but a human using AI. Absolutely. Absolutely. Well, the same boat for companies. So companies who are trying to use AI to control their population because they're stuck in a industrial or colonial model of organizational management, that's going to be very vestigial.
That's probably we're watching the Neanderthals in that, in that moment where you're going to have to start treating people as people. What I'd love to see is I'd love to see big, large company businesses. Competing to get well performing teams and showing those well performing teams, their resume, the company's resume of what they have done for the employees in their communities.
That's what I want to see. I want to invert the model so employees are not asking a corporation for a job because they need healthcare, which is ludicrous. Yes. And if you study why people stay in organizations and it's because they need healthcare, that human being does not have physical or psychological safety.
They're not innovating. They're not doing more than participating to get physical and psychological safety. It's very archaic, medieval.
[00:53:21] Justin Grammens: Very, very true. Very, very true. And we break that cycle. And I guess in some ways, yeah, just open it up for, to let people to be able to explore. Try out these, I mean, I, I hear some companies that are just like, nope, we can't even use chat GPT within our four walls, right?
Or no, you can't use, um, copilot, you know, you can't use any of these tools that are affecting them that are going to make you become a better, I guess a more productive, you know, employee number one, but some of the companies don't see that because they're too worried about intellectual property and data leaking.
But also in a lot of ways, it actually opens up doors and other ways for them to learn. You kind of touched on that earlier, you know, geez, if this code completion tool writes out a. A method in a different way that I hadn't really thought about, I maybe I won't apply it today, but it's like, huh, that's a different way to look at the problem.
And it
[00:54:06] Beth Rudden: also solves your cold start problem where a lot of people, um, I was an anthropologist. I studied everyone because that's what I do. Most developers go through three stages. They learn to copy and paste code, and then they start with a blank page. And then if they get mature enough, they may learn to read other people's code.
But that blank page, that's a great use of chat GPT is the cold start problem. Like, you know, it, it really, as you said, it starts with, with things that it can be very, very helpful. And I understand the security concerns. I get it. I, and it, there's also a commercial license that you can do through APIs. You can come to me and I can write you an interface so that you cannot use, at least use it through a commercial service where they cannot take your data and use it for the training set, or if they do, maybe these large companies could sue them, use their powers for good, but I don't know.
I. Do you think that we are in a time of transformation and yes, it's hard to take those plunges and change. But in this particular time, you need to elevate that 7 percent of your ecosystem right this very second if you want to survive to the next region, because we're in this hyper time of change and we see these cycles all through history.
So it's not something where everybody always says, like, the history is written by the victor, right? Going back all the way full circle to what I started writing about or talking about with you is actually it's the archaeologists hundreds of years later
[00:55:44] Justin Grammens: that are writing
[00:55:44] Beth Rudden: the history and that archaeologists hundreds of years later might have a very different culture that you can actually impact.
today. So take a longer view of what you're thinking about. Instead of going, okay, I need another yacht. So, and I need to get my kids to the next Ivy league school. Come on, wouldn't you want a legacy where it's something so much bigger for longer periods of time?
[00:56:13] Justin Grammens: Agreed. Agreed. Yeah. And that's, that's the archaeologist mindset you're talking about.
Just looking over spans and spans of thousands of years to sort of see how things influence over time. Well, Beth, we've talked for more than an hour here. This has been great. We've talked on so many different subjects. This has been just a treat. A really, really great conversation and really sort of, you know, kind of how I structured this podcast is really just conversations, right?
So. Kind of just let it, let it go with regards to, we're going to talk some tech. We're going to talk some history. We're going to talk about corporations and venture funds and all that sort of stuff. So yeah, we've covered a lot today, but I appreciate your time. I appreciate you sharing your story with all of our listeners and, and I appreciate the fight that you're out there doing.
It feels like day to day, right? Basically trying to educate people on this. And how we can use artificial intelligence to our advantage. When I came into this, frankly, thinking about it more from just a, a view of explainability, but it's, it's so much more than that. I think as you and I have talked about it, it's, it's really more contextual and allowing us to use tools in a contextual sense to really help the next generation.
A lot of ways.
[00:57:16] Beth Rudden: It's personal. Yeah,
[00:57:18] Justin Grammens: so hopefully I summed it up pretty good with that statement, but I'd love to have you back on at some point in the future and I appreciate the time again, Beth. Thank you. Thank
[00:57:26] Beth Rudden: you very much, Justin. This was delightful. You've listened to another episode of the Conversations on Applied AI podcast.
We hope you are eager to learn more about applying artificial intelligence and deep learning within your organization. You can visit us at AppliedAI. mn to keep up to date on our events and connect with our amazing community. Please don't hesitate to reach out to Justin at AppliedAI. mn if you are interested in participating in a future episode.
Thank you for listening.