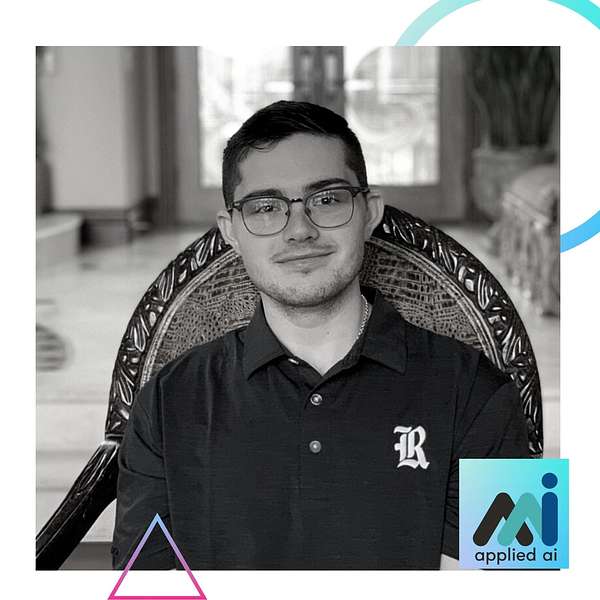
Conversations on Applied AI
Welcome to the Conversations on Applied AI Podcast where Justin Grammens and the team at Emerging Technologies North talk with experts in the fields of Artificial Intelligence and Deep Learning. In each episode, we cut through the hype and dive into how these technologies are being applied to real-world problems today. We hope that you find this episode educational and applicable to your industry and connect with us to learn more about our organization at AppliedAI.MN. Enjoy!
Conversations on Applied AI
Cameron Wolfe - AI Personalization Services
The conversation this week is with Cameron Wolfe. Cameron has a passion for leveraging academic research and industry to solve large scale industrial problems. He is currently the director of AI at c, personalized search and recommendations platform for D to C e-commerce brands, where he works with an amazing team of engineers and researchers. He holds a PhD in computer science from Rice University, and a bachelor's in computer science from UT Austin. Finally, he's an avid fan of Mixed Martial Arts MMA and is actively training in Brazilian jujitsu. He enjoys exercising, going for walks, runs or hikes nearly every day, preferably with his dog Theodore and his fiancee, Sara. Some of his other interests include brewing, roasting coffee, reading books and cooking.
If you are interested in learning about how AI is being applied across multiple industries, be sure to join us at a future AppliedAI Monthly meetup and help support us so we can make future Emerging Technologies North non-profit events!
Resources and Topics Mentioned in this Episode
- CameronRWolfe.me
- Cameron Wolf on Twitter
- Rebuy Engine
- Freshman Research Initiative UT Austin
- Neural Networks Research Group
- Deep (Learning) Focus Newsletter
- Cloud Bigtable
- Dask
Enjoy!
Your host,
Justin Grammens
Cameron Wolfe 0:00
All of these different tools that you see from different like search and recommendations widgets that are on the website. All of them tend to be powered by AI. And they're generally referred to what we call like a personalization service, which just refers to the fact that taking the generic shopping experience in E commerce and personalizing it based on your behavior on a website.
AI Announcer 0:23
Welcome to the conversations on applied AI podcast where Justin Grammens and the team at emerging technologies North talk with experts in the fields of artificial intelligence and deep learning. In each episode, we cut through the hype and dive into how these technologies are being applied to real world problems today. We hope that you find this episode educational and applicable to your industry and connect with us to learn more about our organization at applied ai.mn. Enjoy.
Justin Grammens 0:54
Welcome everyone to the conversations on applied AI Podcast. Today we're talking with Cameron Wolfe. Cameron has a passion for leveraging academic research and industry to solve large scale industrial problems. He is currently the director of AI and Rebuy, personalized search and recommendations platform for D to C e-commerce brands, where he works with an amazing team of engineers and researchers. He holds a PhD in computer science from Rice University, and a bachelor's in computer science from UT Austin. Finally, he's an avid fan of Mixed Martial Arts MMA and is actively training in Brazilian jujitsu. He enjoys exercising, going for walks, runs or hikes nearly every day, preferably with his dog Theodore and his fiancee, Sara. Some of his other interests include brewing, roasting coffee, reading books and cooking. So I love it. It's gonna be a great conversation Cameron. Sounds like you've got quite the rounded life. And thank you for being on the applied AI podcast today.
Cameron Wolfe 1:44
Yeah, definitely. Thanks for the invite.
Justin Grammens 1:46
Cool. Well, you know, I talked a little bit about, you know, being the director of AI and ribeye, but maybe you can bring our listeners up to speed with regards to, you know, kind of how you got into that position, and maybe what the trajectory of your career has been.
Cameron Wolfe 1:58
Yeah, definitely. So I got into AI quite a while ago, but pretty recent, compared to a lot of other people. But when I started my undergrad in 2016, at UT Austin, I came in as like a mechanical engineer, I guess. So I knew I wanted to be some form of engineer, but I had no idea what discipline. So I kind of did that at first and then quickly realized that I really enjoyed computer science through a research lab that I became a part of. So at UT Austin, one of the cool things about the university is that for freshmen, they have this awesome thing called the Freshman Research Initiative, is like a first year undergrad to get involved with legitimate academic research. And I joined fri or the Freshman Research Initiative, and ended up in a stream or they call it a stream. It's just like a lab for freshmen that did research in neural networks. So I had taken pretty much zero computer science classes at that point. So I went in kind of backwards, I started with neural networks, and then learned about everything else afterwards. But yeah, I got involved in that stream. I was doing research and optimizing neural networks with like gradient free methods. And I loved it so much that I ended up switching my major to computer science, I continued doing research with the lab that I was affiliated with throughout all of undergrad, published a couple of papers with them, and just got really interested in as my been kind of addicted to all of AI stuff ever since. So in terms of ribeye, basically, the way that I got into that position is somewhere during undergrad, I think after my junior year, I got an internship with Salesforce on their commerce platform. So I wrote during that internship, some of their content based recommendation algorithm. So content based means just, you know, recommending products that are similar to products that were users looking at. All of that was just based upon, like different forms of Bert models that use like images and product descriptions and text as input. So I worked on recommendation algorithms at Salesforce for a very long time. After that, I was an intern for two years almost year round at Salesforce, I stayed on after like the first summer that I interned there. And that internship, just working with them went into my PhD. So I worked for them for quite a while work for a different startup during my PhD. And then once I graduated my PhD, I wanted to get back into the E commerce space. So now I'm the director of AI at ribeye, where I am again, working on recommendation algorithms and search search algorithms of different comments.
Justin Grammens 4:31
That's awesome. Yeah, we'll get into ribeye here a little bit later with regards to what specifically you know, the product does and how your team works on it. But I'm just kind of curious, you know, you kind of went through the whole academia side, getting your PhD and everything like that. Did you ever consider maybe staying in academia and teaching this stuff or what kind of drew you into the industry? Yeah, definitely.
Cameron Wolfe 4:50
So I enjoyed my time doing research, especially, you know, during my PhD when I got to, you know, devote full time effort to doing research I guess kind of part time because I did have a job during my PhD. But yeah, I definitely enjoyed it. I think the main thing that turned me off from it was the publication process. So coming in is a first year PhD student. And I think within my first like, three or four semesters, I tried to publish four or five papers. And typically, what happens is that your papers get rejected multiple times, and then you have to constantly revise them and resubmit, and so forth. And since there's so many people doing AI research, the bar for papers that get accepted is just incredibly high. And the review process is very noisy, because they have to ask a lot of people to review, even if they're like, first year PhD students, for example, because there's so many papers being submitted. So the review process in general was just like very noisy, very difficult, very demotivating. And throughout all of that, I realized that I love doing research, but I really dislike having to formally publish that research inside of like a conference, for example. So yeah, I mean, part of like any position, even in industry, you're doing research, it's just you might not have like publications associated with it, you're mostly just trying to find what works and deploying it into whatever platform you're working on. So for me, that's kind of like a perfect mix. But I think the interesting thing about myself is that I really enjoy teaching AI to other people. So I have a newsletter that I write, for example, where I try and distill, you know, topics and research to make them more understandable to people. And I love doing that. It's one of my favorite things. So I could see myself, for example, maybe teaching classes at the university, eventually, if I can figure out a way to do that, but probably unlikely that I'm going to be devoting like very significant effort towards publishing papers and conferences anymore, just because of my experience, it kind of took the fun out of it. For me personally,
Justin Grammens 6:56
totally, totally get it. No, I think you and I have actually have a fair amount of things in common. I mean, I actually am an adjunct at the University of St. Thomas here in St. Paul. And so like you, I think I do actually like to give back and, and teach people about this stuff. But it's not something I would want to make my career out of, you know, maybe once I get to be 65, or something like that. I want to kind of ride off into the sunset, maybe teaching at a university and doing doing research. But I'm really more about I think hands on applications, right. And actually, being able to move fast. It feels like sometimes, at a university, you can be tied in especially if you're on salary and all other things say no, but I would consider being an adjunct as possibly something in your future, you can kind of have the best of both worlds. Yeah, the other thing is the newsletter we haven't applied yet newsletter. So I'll definitely send you a link for that. But we will include you know, the links to whatever links you want to send me so all of our listeners can subscribe to your newsletter. So anything we talked about here in this program, we'll make sure to have links in the show liner notes. What was your thesis on for your PhD? Was it around this area of recommendation engines?
Cameron Wolfe 7:55
No. So a little bit of it was I had some papers that I wrote at Salesforce that were more specific to recommendation engines. But for the most part, like including those in my thesis was a bit of a touchy subject just because of IP issues. So they're out there on archive, you can find them on my website, but they weren't formally part of my thesis, I guess. But my thesis in general, it's a bit of a thing. Frankenstein thesis is what they call it. So it doesn't have one unified topic. But in general, it's focused on the idea that I really liked, which is just how can we find practical and simple tricks to make training and using neural networks more easy. So there are a couple of different things. But in general, when I look at the space of like things that people propose, and what actually ends up being used, there are three major considerations that go into whether some something that you propose is actually useful. One of them is like cost. So if it's like super expensive, people aren't going to use it. Similarly, like time is like an issue. So there are multiple forms of costs. The other is performance. So whether it performs really well obviously, if like something that you propose doesn't perform well, then nobody's going to use it, because it's kind of worthless. And then the final one is just simplicity. So oftentimes, you'll see like modifications or techniques that people propose and research, and they work really well. But practically, the cost of implementation is very high, and the risk of bugs is like very high or something like that. So the adoption would be less so than if it was like a simple technique that achieved similar performance. So all of the different papers that I propose throughout my thesis or something, kind of in that area, I have a couple of different pruning papers. So just taking large neural networks and making them smaller without sacrificing their performance. Most of those papers were kind of provable pruning techniques. So they come with theoretical guarantees, but are so practically usable. I looked into quantize training techniques for neural networks. So right now we see a lot of post training quantization with lol and so forth. There is some like during training quantization. But my research looked into basically treating the level of precision that you use during training as a hyper parameter schedule, so that you're constantly and dynamically varying the precision while you're training the neural network, which is pretty interesting. And I have some other papers on hyper parameter schedules as well. But in general, hyper parameter schedules are just something that's super simple, that there's a lot of cool things that you could do and get some gains and efficiency. And then finally, I looked some into streaming learning, which is basically instead of just performing a bunch of epochs over a fixed data set like we normally do to train a neural network, you consider a stream of incoming data. So you're getting one new example at a time, and trying to figure out how to train your neural network that way. And I have like a big paper where I talked about just simple techniques for applying that to deep learning using like data augmentation and something called replay mechanism, which just caches data and kind of forms mini batches by sampling data that you've seen so far. And that one actually has like proofs with it as well, which was pretty interesting, because I tried to do it thinking that it would be impossible to prove something in a setup like that, and then ended up getting something which was cool. So a lot of different stuff. But like at a high level, it's just looking at common problems that you might have with neural networks and trying to propose simple stuff that you could use that would be practically valuable. Yeah,
Justin Grammens 11:24
fascinating, really cool. spans the gamut of a lot of different areas. Yeah, the, the class I teach at the university actually is focused around machine learning and IoT. And so really talking with students about how to make their their machine learning models as small as possible, because it's usually running on something that might be battery powered, or something that is a cellular connected. So you want to make sure it's you know, that the model uses the least amount of memory as possible and as the smallest possible. So some of these methods that you're talking about sort of I think ring true, although I've not thought about it, doing it while you're training, right. A lot of these things get optimized after better run it right through an additional step. So fast. Yeah. Or Is any of this stuff published at all? Maybe I mean, after this, you can definitely send me some links. If there's anything you want me to write to include around these papers you did for your thesis?
Cameron Wolfe 12:12
Yeah, most of it is published. I think there are probably well, not most, I would say about half of it is published. I think it was five papers in total, and three of them had been published so far. So yeah, we're getting there. The other ones so need to just be resubmitted over and over again until they get published, I guess.
Justin Grammens 12:29
Yeah, sure. And that can be, that can be a big process. The other thing too, I'm not sure if he knows that we do monthly meetups with the planning group. And we also have a conference so we should touch base with you at all want to present anything that you have or somebody at ribeye wants to talk about how it's being applied to definitely like to get you guys on the schedule. Sounds like you've gone deep on a lot of this stuff. So let's, let's talk a ribeye, maybe you can enlighten our listeners regards to what what ribeye
Cameron Wolfe 12:55
does. Yeah, definitely. So ribeye is an E commerce company. So basically, we're trying to take all of the tools that an E commerce site like Nike would have with respect to AI, and provide them in an easy to use manner to anybody who is not Nike. So if you go on Nikes website, which I'm just using this as an example, given that Nike has a huge engineering team, and an awesome like aI power personalization service on their website, basically, like you can search stuff, it will use like aI powered search to bring up relevant results, probably learning from things that you've looked at on the website to optimize the search results, and so forth. And then the same thing for any recommendations that are displayed on the website. They have aI powered recommendations, widgets that are displayed, whether it be for like, complete the set. So displaying bundles of products that go well together, or just you know, populating top selling products, products that you're likely to want to buy based on what you've viewed in the current session, and so forth. So all of these different tools that you see from different like search and recommendations, widgets that are on the website, all of them tend to be powered by AI. And they're generally referred to what we call like a personalization service, which just refers to the fact of taking the generic shopping experience in E commerce and personalizing it based on your behavior on a website. So you can think for example, if you're someone who goes and buys your coffee from like an online store, if you're someone who typically buys whole bean coffee, and then you go to the search bar and search Kona coffee, for example, the first thing that comes up should not be K cups. If you have like a good personalization algorithm, it will pull up Kona coffee, but it'll pull up the whole bean coffee because that's what you tend to purchase in the past, right? So there's a lot that goes into it. But the entire goal is trying to take search and recommendation algorithms and basically personalize them based on data that we have about a user. Typically we don't use like any cookie data as well which like people That used to be the way that people do it. But cookies obviously are not as widely accessible now. So the cool thing about rebuys, we don't really depend on that. All of our stuff is based on just session data. So if you're logged into a website, and we have purchase history, that's great. But most of it is just based upon how you're interacting with a website. And it will dynamically decide like what products to show you based on that, which is really cool. So that's what personalization is ribeye specializes in that area for search and recommendations. But practically, how it's actually used is mostly on Shopify, we have a multi platform offering now. So it could be beyond Shopify. But rebuys core product, as of right now, is a Shopify app that you can download and put onto, you know, whatever website is selling stuff on Shopify. And really what you can do is take some of the tools that we provide, and configure them to exactly how you want your recommendations and search to work on your website. So it's not just a one algorithm fits all type of thing. So we provide a bunch of different tools that you could use for personalization, we provide a default, like recommendations and search algorithm. But within our portal, we for every like widget or just like front end recommendation section that you put on your website, we provide what we call data sources. And really what this is, is just the ability to define exact business logic of how you want recommendations to be served on that page. So you could just like us, our default recommendation algorithm, but oftentimes, if you're a SaaS provider, and recommendations and search, one algorithm is not going to work well for 7000 different merchants, right, everybody is going to have unique considerations, and everybody is going to want to customize the algorithm in one way or another. So through our data sources, basically, they can go in. And for every place where they're, you know, providing recommendations or even for the search experience, you can personalize that as well. You can go in and define rules based on all of the metrics that we have on your website, and just configure exactly how you want your search recommendations to be served. So for recommendations, this could be, you know, like just saying if this product is what the user is viewing right now, so if it's on this product page, you could serve one algorithm. And then if you're on a different product page, you could serve a different algorithm, you could customize it based on collections, there's pretty much an arbitrarily like number of ways that you could customize it, you could even define rules on the URL, where like, you know, if you send a text message marketing campaign, you can have multiple different URLs that like redirect to personalized populated card and recommendation pages based on different URLs so that you kind of can segment customers in personalized in that manner. And then same thing for search. There's a bunch of different customizations. You can define what keywords synonyms, do product promotions, based on keywords, just all of the different things that you would need to personalize and merchandising ecommerce store.
Justin Grammens 18:02
sounds super powerful, actually. And so just with regards to the Nike analogy, you guys are bringing them that power, everything I talked about kind of to the to the small to the SMB market, right? Anybody with a Shopify? You know, ecommerce website now has all these tools at their disposal. So they don't have a full team like Nike does, right?
Cameron Wolfe 18:20
Yeah, exactly. And the beautiful thing is that you don't need really an AI team to make any of this work. So we actually just launched an AV testing studio within our platform as well, which is really cool. Because my view for how rebar should work is that basically, we provide all of these different AI powered tools, I can optimize a website, so that someone who is running like, you know, some SMB brands, now we have like bigger brands. So it's not always the case, sometimes our customers have engineering teams. But like you could think that if somebody is running their brand, they're not an AI expert, but they want to use these cutting edge personalization tools that Nike has, my vision has always been that ribeye would kind of be the tool that can plug in, that you can optimize and implement all of these policies without having any AI expertise. And the way that we do that is by making all of the tools kind of intuitive to understand. So providing health documents where people can really understand what the purpose of each tool is, without an AI background, which an E commerce a lot of the times is more than possible. And then basically providing very detailed metrics, analytics and AV testing functionality, so that people can try these different functionalities, and then pick the best ones that work for their website over time. So really, using rebuys somebody could you know, implement different policies, try out different AI powered tech to personalize their website. And then slowly over time, optimize what they're doing based on you know, rigorous analytics on their website, so that they know what they're doing actually works and is yielding some kind of ROI in terms of like average order value, customer retention or something like About.
Justin Grammens 20:00
Yeah. And so I'm just thinking about the amount of data that you probably have access to people. And what what do people have to do? I mean, do you ask them to kind of open up their, you know, like their purchase history for people like it's, again, I'm not super familiar with Shopify with regards to like, can somebody just do one click? Here you go, you have all, you know, all my data? Or are you guys ingesting data from other data sources that a company might have to open up and give access to other databases and what?
Cameron Wolfe 20:27
Yeah, so I think like the types of sources of data that we use would be pretty typical for a recommendation service, like we definitely have very stringent policies around PII and so forth. None of our machine learning algorithms use like any form of PII, which is cool, because we were very keen on being data compliant and not doing anything that's going to get us in trouble or be viewed as a negative by any of our merchants. So any machine learning related technology that even is kind of seen as something that somebody would be upset about, we definitely are very cognizant of that and make sure that our machine learning practices are basically just not questionable, I guess. So we're very careful about that. But basically, what we'll use to serve recommendations is what you would think of for like pretty much any recommendation service, we use the product catalog. So just features of the products, all of the information that we have about, you know, descriptions, titles, images, and stuff like that. And then we also use interaction data from the user on the website. So if they're inside of a current session, and they click on a certain product and add to cart, you can take that data and kind of use that to personalize what they're going to see next. And it's pretty easy to kind of learn dynamically, how you should personalize their experience based on what they're interacting with on the website. And then that for the most part is like the core of what we're going to use, the primary reason for that is that like there is data available, or there could be data available in terms of who the customer is, and like what they purchased in the past. But typically, when somebody visits in e commerce website, just with how the general flow exists right now, they don't log in prior to searching, browsing and purchasing stuff on the website. Typically, the workflow that you see is that they come on to the website, they're anonymous, they're going to like add stuff to cart, and then proceed to checkout. And then at the checkout part is when they would actually like login to retrieve whatever payment details that they out. So it like that information is there. But the majority of the time users that are kind of shopping and expecting a personalized experience on a website will actually be anonymous for the majority of the time that they're on the website. So you have to kind of use dynamic interaction data that you get personalized rather than relying upon having this vast history of things that people have looked at before.
Justin Grammens 22:53
That's interesting. Yeah, no, I hadn't even thought about that. But yeah, you're right. I myself do the same thing. I'll add a bunch of stuff to cart browse around. So it's I have no idea who I am until they actually sign in. So that that that is an interesting challenge. And, you know, it seems like you guys probably wrote all your own proprietary technology. I'm sure you're writing. I'm using some open source tools, I guess. Can you share in any of those? Or is it all just pretty much from scratch everything?
Cameron Wolfe 23:18
Yeah, definitely. So I mean rebuy in general, there's some things that we use that are closed source, mostly just like GCP tools like big table and stuff like that. So there's some things that we use that are closed source, but in terms of the ML team, we're very heavily emphasizing open source tools. So like for all of our data, exporting and warehousing jobs, we rely upon preselect, we use Dask, for distributed compute. And then pretty much everything that we have is built upon that we also use like ML flow for a model registry and so forth. But yeah, I think for most of the ML stuff that we do, we're very heavily emphasizing open source just because it tends to, in my opinion, be maintained and faster adapt to stuff that's happening in the ML space, because things tend to change. And now all in deep learning so quickly, it's very important to have tools that will adapt to those changes and kind of meet the expectations of people who are working with them.
Justin Grammens 24:16
Yeah, true. True. True. Yeah. I mean, it sounds like a challenging problem, I guess. I mean, one that's been probably, you know, around since Amazon first launch, you know, basically, it's like, how are we gonna, you know, recommend something to somebody? And I mean, you kind of have to come up with the answer pretty quickly. This, this system needs to be pretty performant. And it needs to be highly tuned, I think, to certain users at the same time. Are you guys going through sort of a continual retraining of this thing? I'm just trying to think kind of how the system would need to be architected?
Cameron Wolfe 24:44
Yeah, so I think like E commerce in general is a beautiful space to be in because it's kind of a sandbox for different AI techniques. And one of the really wonderful things I love about e commerce is that there's a balance between stuff that needs to be computed offline, and stuff that needs to be computed online. So one thing that's like very notable in this space is that all of the products data, typically is something that is not changing super quickly, there are some things that change like stock levels. But most of that is not super related to like deep learning, maybe you can have that as a feature. And like a downstream model, where it's like, if the stock is super high, you can kind of promote it a bit more. But for the most part, most of what you see in the product catalog is not changing super dynamically. And because of that, you can use very, like, I guess, expensive, but just large neural networks to form your understanding of products, which is really cool. So any embedding models or different models that you use to extract information about products, you can use really big models and run them for offline batch inference. So that information is available in real time for other applications. So that's like the first part. And that part of like forming product understanding, which in a lot of ways is the core of a lot of things that you will do in terms of personalization services, you can use really big neural networks computed all offline, and there's not like a latency issue, which is really cool, then the question is, how do you take that information and use it to dynamically adapt, you know, the website based on what a certain user is doing? And the answer is that typically, you're using real time ranking models, right. So what you typically see and recommendations in search, they're pretty similar problems, but also different in some ways. But both of them and this is argued by some people, people like kind of propose more complex ways to look at this. But in general, ranking and error recommendations and search are a two stage process. The first stage is retrieval, which means basically, I have some information. And I want to use that information, go through all of the products that we have. So could be tons of products, and efficiently identify, you know, some subset of 100, maybe 1000 products that would be most relevant to this scenario. So as a concrete example of that, maybe you're someone who's sitting on a product page, so you're sitting on the page for one particular product on the store. And that's your context. So you want to say, this is the user, this is the product, go find me a subset of things that might be relevant. And this is called filtering or retrieval. So that has to run very quickly, because you're going in retrieving over all of these different products. So there are a bunch of different algorithms for that, like the typically for recommendations, it's going to be either content based or collaborative. So collaborative filtering is like a retrieval algorithm. It's very famous originally proposed by Netflix. And then content based retrieval is just similar products, engines. So one way of doing that is like getting an embedding vector for a product and then running a vector search to find like the 100 most similar products or something like that. So I see all of that is like very fast, you can run that retrieval algorithm, and we'll go get stuff give you like 100 or 1000 products that are roughly kind of considered to be relevant to your current context. And then after that, the question is, how do you decide which one of those products are like the first, second and third products to be shown, so you have to re rank them or just rank them based on how relevant they are to the current scenario. And that ranking component is really where the real time stuff comes in. So for retrieval, you can do a lot of stuff offline, you can compute vectors. And then the retrieval is just running, you know, vector similarity search online, which is fast. And then the ranking, basically, you just have to train a smaller model that can take the information that you have, and rank all of these results based on relevance. So there are like a million different ways to do this. Like, one way to do this would be to train just like a regression model that takes the embedding for the current product, and an average embedding of like, all of the products that a user has looked at concatenates them and then just predicts, like, what is the likelihood that the user is going to purchase this product, you could train that over just like a ton of purchase data, and that will predict like likelihood to convert. And then you can just like sort based on that score. You could also use like learning to rank models, which are typically like decision tree based models, where you just take all of the information that you have. So it could be, you know, the vector similarity score, it could be the collaborative filtering score, and then information about the product information about the user. And then you just throw all of those into an x g boost model that predicts like a score, and then you sort based on that score. So typically, like all of that will happen in real time, so you're constrained to small models or decision trees or something like that. But by kind of dividing sort Archer recommendations into those two components of filtering and then ranking, you can separate it and see that most of the stuff that you do for filtering is offline. There's only one part that's online, which is basically using those offline computed features to run a search. And then most of ranking happens online. But it's just with smaller models more or less.
Justin Grammens 30:23
That's fascinating. That's cool. I mean, and who would have known if you go onto a website, all that stuff is happening behind the scenes. And so you guys are sort of, like you said, like a plug in on top of Shopify. So essentially, API calls that are coming into your your guyses data center to do all that turning of all that data and sending it back to, to the website to present it, right.
Cameron Wolfe 30:44
Yep. And we're not 100% Just on Shopify. So we also have a multi platform like API offering that we're building out more and more every month. But yes, like, for the most part, all of the ML component is just a set of API's that each have like different functionalities. So we have products API's that will ingest products and form like all of the vectors that we need for them. We have events and analytics API's that are taking in like purchase behavior, and, you know, kind of learning from that, search API's, stuff like that. So it just all of these different components that you would need are kind of packaged up so that you could easily like a normal developer could use them to do all of the personalization stuff without knowing anything about AI.
Justin Grammens 31:28
Yeah, yeah. Cool. That sounds like an interesting challenge to to kind of try and support a number of different commerce platforms, I guess, right? I mean, probably a smart way to kind of pick one of the biggest and sort of cut your teeth on that. But, boy, I mean, how are you going to support all these other ones? And even just kind of mom and pop shops, right? I mean, it's gonna be Yeah, well, again, you're working on it. And I will put links to I mean, is this kind of like in beta? Could I Sign up today for a platform that maybe that I've already written? Yeah, so I
Cameron Wolfe 31:57
mean, rebuy is not in beta. So on Shopify, you can go on to Shopify now and download ribeye, where like the top recommendations, and soon to be searched platforms on Shopify, we have like over I think, at this point, like 7200 merchants that use ribeye, which is really cool. A lot of the multi platform offering is in beta, because it's something that we just started working on at the beginning of this year. So until that becomes more developed, I guess it is in beta for now. But yeah, like the strategy there was basically that we built this large customer base on Shopify, Shopify is dominating the space of like, just the E commerce SAS market. So just this space where people are trying to build ecommerce stores for people or make that really easy to do. And there are other platforms like I work for Salesforce commerce, which is another option. There's also like big commerce and so forth, there's several different platforms. But the strategy that we had was basically we built revive for Shopify, which is a good thing because Shopify is very dominant in that space. And it's a great ecosystem to grow an app like that. But building ribeye, like individually and tightly integrating it and to every other platform like we did for Shopify is not like super reasonable to do. So we built rebuy for Shopify, we're going to maintain that and definitely build upon it and emphasize making that product the best that it can be. And then we're going to build rebuy for everything else, which is just an HDI centric solution to where anybody who wants to use our tools can kind of use them via our developer API's. And yeah, we're doing our best to create really good documentation, similar to how Shopify creates awesome documentation for all of their API's. We want to make it kind of easy to work with revives the developer.
Justin Grammens 33:45
Yeah, sure. Yeah, I can see you guys kind of be in like the stripe, you know, you know, Stripe can integrate with anything, just through API calls, if you want to do payment processing. So you guys could be kind of the recommendation. And search engine. A lot of that stuff, though. I mean, so if I want then I then I need to make API calls it sort of ship you my data, right. So here's this customer, and you guys need to ingest all of this stuff. Yeah. So we would have to ingest product data, and then just like customer analytics data, so that we could adapt to what they're looking at while they're on your store. Cool. Cool. Well, how many people have been talking about, I mean, it has the word chat GPT and large language models. And some of that showing up in where you guys are looking, particularly probably around around search where customers asking for some of these types of tools to Yeah,
Cameron Wolfe 34:29
totally. So language models is like one of the areas that I specialize in, and I love working on them. So pretty quickly after you know, chat GPT came out and people were really psyched about language models. We started developing what we call ribeye asst, which is our language model powered discovery platform. So really, what it is is just basically an agent system of language models that can ask the shopper questions, kind of understand their preferences and lead them to the products that would be most relevant them. So it's really like a discovery focused search tool. So instead of like typical search, where you put in very specific keywords and expect, like a very specific product to come up, revise this, and it's something that you could use when you're not like completely sure about the product that you want to purchase. So you can come to us with a question of like, I'm going on a camping trip soon. What do I need? And does this store sell the products that would be, you know, important for that? And then it can ask you more questions better understand what you're looking for, and guide you to the relevant products. So yeah, we build that it's been shipped right now. It's actually part of our checkout cart experience. So ribeye has this checkout cart called Smart Cart, which is like an optimized checkout card on Shopify, that has a ton of one click recommendations, you know, incentives and stuff like that. It's currently our top selling product, I guess people use it. And they love it because of all the different customizations that you can do to optimize your checkout cart. And right now, rebuy assistant is deployed inside of the checkout cart, where it will just display a chat bubble, or a chat section, I guess, and say, did you find everything that you're looking for today or something like that, so that inside of the checkout card, if there's anything that you missed, or you like might have purchased that you didn't see, while you're on the store, you could talk to rebuy assistant to try and find other products that might be relevant. And for sure, we're planning on expanding that beyond the checkout card that just happened to be like the first place that we shipped it. But overall search is becoming more of a hybrid experience over time, I think there are different reasons that people will typically go to the search bar, whether it be like discovery focus, or searching for something very specific. And language models are incredibly powerful for those discovery focused applications. So when a person doesn't know what they're looking for. So over time, I'm sure that we're going to integrate it more tightly with search, find ways to expose it as like a widget on ecommerce websites, or just basically find the balance and the most intuitive UI to allow people to use it. Because it is very useful, but only for a particular type of search. So if you if you know exactly what you want, and then you go into a chat bubble and start talking to a language model, it actually might be more annoying to go through that than to just perform a normal search. So yeah.
Justin Grammens 37:21
Cool, cool. Those are the buzzwords of the day. And I'm sure you guys were already looking at thinking about search for Chechi. He kind of came on the scene. You know, one of the things that I do like to ask people that are on program is, you know, do you have any advice for somebody who's maybe coming out of school today and maybe wants to get into this into this field? Or their their books that you've seen or their classes that that you may want people to take sounds like they have an awesome program at UT Austin, but are there conferences? You know, how would you advise people on that?
Cameron Wolfe 37:51
Yeah, I think there's a ton of people that can give like really good advice for how to become a data scientist. Yeah, so I tend to like, recommend people to look at advice that different people give. But the unique aspect of what I've done, I mean, there's typical advice of like, you need to go read papers, you need to implement stuff, because learning by doing something is way better than just reading about stuff. You need to publish projects on the internet that you've done, find a mentor, so forth, definitely done all of those things. But I think the main unique thing that I've experienced that I started pretty early, that helped me to kind of more quickly gain opportunities at like Salesforce and beyond, was probably making sure that I'm always writing about the stuff that I learned on the internet. So one thing that I started doing very early is that when I got my first internship, after my sophomore year of college, I learned a lot, I did a ton of reading about how machine learning works, and so forth. And as I was reading about this, I wrote overviews of what I learned on medium, and then just publish them. And then after I wrote a few overviews, I got picked up as a writer by Towards Data Science, which I've been writing for them to this day. So that was really cool. And it looks really good on a resume if you have, you know, experience writing for a publication like that. But I've kind of continued that trend of taking the things that I've learned and read about and writing about them constantly and publishing them for people online. I've continued doing that to this day, whether it be on Twitter, or via my newsletter, stuff like that. And through that, I think it's gained me a lot of opportunities, simply because people start to see your content. And they'll start to contact you to talk to you about various different kinds of things. So it could be VCs contacting you about like starting a company. It could be people contacting you to work for them could be consulting opportunities, but overall, just taking what you've learned and writing about it, and then publishing it on the internet, to me has been hugely beneficial almost to the point where I can't overstate ate enough how important that would be. And it's something that not a lot of people do, because it's hard. And it requires a ton of effort. Like I publish a new newsletter every week, I probably spend like 10 hours on it every week. And if you have a job, but as a data scientist 10 extra hours a week is not not trivial. Because, you know, data scientists have a hard job to get to begin with anyways. So it's hard to do. And because of that, not a lot of people will do it. And because of that, you're more likely to meet people or gain recognition because of that. So it's, it's hugely beneficial.
Justin Grammens 40:34
Okay, no, 100% I can't agree with you enough. And I, I think some people, they get done with school, their, their degree or whatever, then they're like, I don't need to learn anymore. And I've found later in life, actually, I enjoy learning all this stuff. You know, more actually, because I'm learning it, and exploring it sort of, on my own. And that's what this for this podcast really is just to meet awesome people and talk with them and just kind of jump into the field. I've been doing this podcast for more than three years, but learn so much. And again, back to what you say you got, you got to regurgitate it, get it out there and let people see it. And a lot, a lot of doors will open for sure. This is a complete 180 or whatever. But I did want to get back to your MMA stuff. Right? And I'm just kind of curious more personal stuff, you know, how did you how did you get into this? And are you actually finding I'm a, I'm like an avid runner, I really got into running marathons about three years ago. And, and so I go out in the morning, and I'm always training for something and it forces me to be focused in the morning, and I take on the task that I hate the most kind of in the morning way, which is getting up early and getting, you know, a nice long run in do you see any overlap? See, do you see yourself getting into this? Mixed Martial Arts? Because of because of the work you do? Or because the work you do you got into it? Do you think it plays at all into this?
Cameron Wolfe 41:51
Yeah, totally. So first of all, totally agree with getting out of the way the stuff that you don't want to do in the morning. For me, I cannot work out in the morning. So I don't do that. But what I do do is the first thing every every day that I do is work on my newsletter for like an hour, an hour and a half. Because to me, writing about stuff is always a creative mental friction, it's like one of the hardest things that you could do. So I always do that first thing in the morning because if I try and do it like after work or something, there's no chance I'm just going to be too demotivated to actually do it. But in general, my advice would be that for anything that's going to be difficult to do. So learning about AI becoming an expert now is extremely difficult. And it will take a long time, it requires a lot of focus on that. But at the same time, I found it to be hugely beneficial to have something outside of your main focus that you can also focus on. And the primary reason for that is because, you know, your endeavors and AI or whatever else you're pursuing, are not always going to be going well, you know, all of different pursuits, all of life is kind of a sine wave where you have good times, you have bad times. And what I found is that by having something else that you're kind of focusing on, there's a greater likelihood that if you're kind of down in the dumps in your efforts on AI, you feel like you haven't made progress, you're struggling to get like your next job, maybe your papers are getting rejected, there's at least a high likelihood that at the same time, your efforts in like jujitsu or MMA are going pretty well, maybe you have like a good, good session where you're training with people, you're being successful using new boobs and stuff like that. And that has a huge likelihood to kind of, you know, avoid you from getting too upset about, you know, something else that might not be going well. So that's one thing, if you have multiple things that you're doing, the highs and the lows, like tend to kind of balance each other out. The other thing is that I think there's a ton of benefit just to being active and doing like mental work. So a lot of people especially now in AI, as people will talk about, like burnout with like too much stuff going on at once. People talk about just working constantly and stuff like that, to me, there's like diminishing returns with the amount that you work. I try and work a you know, a good amount, make sure I'm constantly making progress. But to me, it's just very important to supplement that work with being active going out and doing stuff and having time away from work, primarily because it will kind of keep you from having burnout, which nobody wants to have that kind of just pushes you in the opposite directions of your goals always. And oftentimes if you're like stuck on something going and being active, whether it be working out going for a run doing jujitsu or whatever. I think a lot of people would be surprised how many of their problems they figure out during that time away from their work. So it's important to kind of detach and have that opportunity to think about passively or subconsciously and kind of see if your problems can work themselves out. And that for me has been huge. And when I started YouTube During my PhD, I guess I wrote three papers that have pretty massive like mathematical proofs inside of them. And for all three of them, components of those proofs have been figured out during jujitsu, or during working out, you know, I'll get stuck on something for multiple days. And the solution never comes when you're like actually working on the proof that comes when you're kind of thinking about it subconsciously, and doing something else. And then you figure out, oh, my gosh, this is how you should like, actually solve this. And the same thing applies to any other form of work, just having that time to do something else and detach will give you a lot of clarity in the other areas of your life.
Justin Grammens 45:37
But totally, totally agree, man. Yeah, this has been a great conversation, Cameron, how do people get a hold of you? What's the best way to reach out?
Cameron Wolfe 45:46
Yeah, so I have a website, it's just Cameron r wolf.me. And you could go there, and it will have links to all of my social media channels, and so forth. I'm on probably most of the social media channels. So I have a medium page where I write for towards data science, I have a sub stack, which is what I use to host my newsletter. And then I'm also on Twitter, LinkedIn. And now threads is like the new thing. So yeah, if you go to my website, links, all of those different things.
Justin Grammens 46:14
That's awesome. Perfect. Well, there any other topics or things like specifically that maybe you you wanted to touch on? Or you think we, we covered it all, but I usually always ask, Hey, you know, I had some questions here. But if there's anything that I might have missed, you're free to bring up? No, I
Cameron Wolfe 46:29
think the only thing that I like to say just because a lot of people don't talk about this, but what we see in the AI space right now is that for the first time member, AI is not just a research topic anymore, you know, my parents are using live chat TPT, or my my mother in law uses chat GPD. She's a real estate agent. And she'll rewrite some of her listing descriptions, which at GPT. So for the first time ever AI is bridge to like the gap of being a research topic. And it's now something that appears in popular culture. And because of that, it's now on AI researchers and practitioners, people who know how this technology works, to develop their ability to discuss these topics with other people and explain them very clearly. So that's something that I like to always say is just like anybody who's working on AI, think about how these things works. Think about how you can explain them to your parents, like it's your duty from now on to make sure that people who use this tech understands how it works and aren't using it and like malicious ways or whatever. So yeah,
Justin Grammens 47:31
that's a whole topic. I think we could talk about the malicious use of AI maybe the next time, next time you're on the program. But yeah, I think you're right, the unveiling of Chad GPT kind of democratized I guess, this tool to allow anybody basically to come in and start typing on it. And the fact of the matter is, obviously, it's been around for quite some time, right? I mean, it was GPG. Three, that there were three other incarnations before it, right. So it was a unique, obviously, a unique company did it, they got enough data, and they made it in such a way that was easy to use, which is, which is awesome. But yeah, I'm 100% with you with regards to AI again, and also not letting people go off the deep end either, like, kind of rein it back in a little bit. You know, this is just mathematics really, at the end of the day. It's just It's fancy math, and a lot of ways it's choosing the next best word to use. But people can totally go way too far with this when they don't really understand it. Yeah, totally. That's what you're saying. That's what you're saying is we got to be, we got to be good stewards of it. We got to explain it in a practical terms, what it means. Yeah,
Cameron Wolfe 48:31
and I think there's a lot of just knowledge that a lot of people that work on these things have that would be useful to other people. And, you know, it doesn't always take, you know, beautiful, intuitive explanation of language models to communicate useful information to people. It could be even as simple as you know, these models have a tendency to hallucinate, which means that they can make up factual information. That's not correct. And a lot of people are using these models treat them like a search engine, for example. So even at the most basic level of just taking facts that are practically useful to people, they're using these models that they may not know, if we can learn to communicate with other people and just kind of find ways to make this information intuitive for them to understand it can be hugely beneficial for pretty much everyone.
Justin Grammens 49:19
Well said. Well, thanks. Thanks again, Cameron for for your time for being on the podcast today. I really enjoyed the conversation and yeah, we'll drop links to all the stuff that we talked about here on the stuff on tour data science and your your newsletter, everything that will that goes here for people that subscribe so thanks again. I appreciate the time.
Cameron Wolfe 49:37
Awesome. Thanks for having me.
AI Announcer 49:40
You've listened to another episode of the conversations on applied AI podcast. We hope you are eager to learn more about applying artificial intelligence and deep learning within your organization. You can visit us at applied ai.mn To keep up to date on our events and connect with our amazing community. Please don't hesitate to reached out to Justin at applied ai.mn If you are interested in participating in a future episode thank you for listening