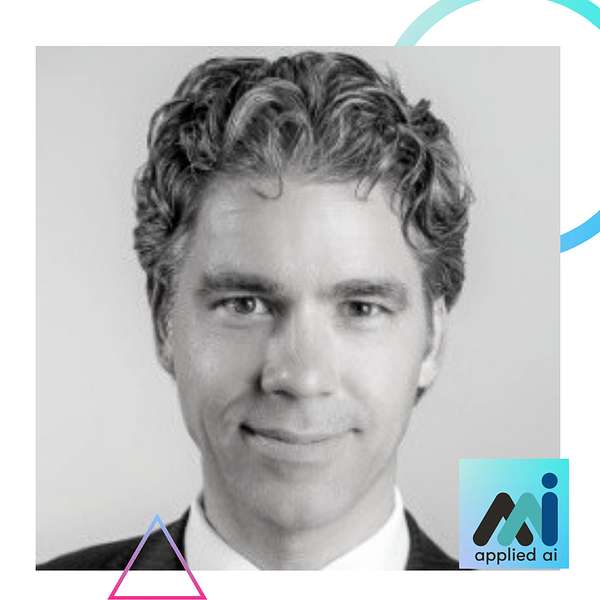
Conversations on Applied AI
Welcome to the Conversations on Applied AI Podcast where Justin Grammens and the team at Emerging Technologies North talk with experts in the fields of Artificial Intelligence and Deep Learning. In each episode, we cut through the hype and dive into how these technologies are being applied to real-world problems today. We hope that you find this episode educational and applicable to your industry and connect with us to learn more about our organization at AppliedAI.MN. Enjoy!
Conversations on Applied AI
Damien Riehl - The Importance of Humanity as We Bring AI and Law Together
The conversation this week is with Damien Riehl. Damien is a technology lawyer who has advised clients on tech litigated tech issues, remedied cybersecurity issues and develop software. His legal experience is diverse over his career, and has been working for a variety of clients from large businesses to nonprofits and individuals. He currently leads the design development and expansion of products at Fastcase. Integrating AI back in technologies to improve legal workflows, and to power legal data analytics. A TEDx speaker, co-founder of All The Music LLC, and on the Minnesota Governor's Council on connected and automated vehicles.
If you are interested in learning about how AI is being applied across multiple industries, be sure to join us at a future AppliedAI Monthly meetup and help support us so we can make future Emerging Technologies North non-profit events!
Resources and Topics Mentioned in this Episode
- Damien Riehl on Twitter
- Fastcase
- All The Music LLC
- vLex
- Natural language processing
- Dolly v2
- The Authors Guild
- Feedly
Enjoy!
Your host,
Justin Grammens
Damien Riehl 0:00
People have asked if AI is going to replace lawyers. And I say, you know, a lot of tasks, yes. But there's a humanity to being a lawyer that really goes to back to lawyers are called counselors. That is, you know, people are in hard times when they have to go to a lawyer, right? They're in divorces, they're dealing with probate with their parents death or something. So they truly need a human touch to be able to help them get through the times that they're going through. So yes, lawyers need to be humans. Doctors need to be humans. And judges need to be human. You could imagine there are enough people that are jaded saying, yeah, that Judge railroaded me, right? I'm in prison because that Judge railroader B. Imagine how bad that would be if they say the algorithm that Google made railroader right, the algorithm that whatever company made railroaded, we need the human to look that person in the eye and say, you and I both understand that you did something wrong, you need to pay the penalty for that. And that needs to be a human and not a robot.
AI Announcer 0:47
Welcome to the conversations on Applied AI podcast where Justin Grammens and the team at emerging technologies North talk with experts in the fields of artificial intelligence and deep learning. In each episode, we cut through the hype and dive into how these technologies are being applied to real-world problems today. We hope that you find this episode educational and applicable to your industry and connect with us to learn more about our organization at appliedai.mn. Enjoy.
Justin Grammens 1:18
Welcome everyone to the conversations and apply to AI podcast. They were talking with Damien Riehl. Damien is a technology lawyer who has advised clients on tech litigated tech issues, remedied cybersecurity issues and develop software. His legal experience is diverse over his career, and has been working for a variety of clients from large businesses to nonprofits and individuals. He currently leads the design development and expansion of products at fastcase. Integrating AI back in technologies to improve legal workflows, and to power legal data analytics. A TEDx speaker, co founder of all music LLC, and on Minnesota Governor's Council on connected and automated vehicles. He's one busy guy, man, I thought I was involved in a lot of things, Damian, but you put me to shame. Thank you so much for being on the show today with your busy schedule.
Damien Riehl 2:02
Thank you so much for having me. I'm thrilled to be on it.
Justin Grammens 2:04
Awesome. Awesome. So yeah, you know, I gave a quick background and really with regards to you know, doing this work at fastcase. You've done this other project called all the music, I'll see maybe you want to start with like, you know, how did you get into this, like, imagine going back in your career and maybe kind of like, give us a trajectory of how you landed where you're at today.
Damien Riehl 2:22
As a music major in college. My undergraduate was in music, I was going to be a choir director that was conducting a Brahms piece and two of my tenors started punching each other in the face. And I thought maybe I don't want to be a choir teacher. So I ended up going to ended up going to law school from 2002. I litigated for about 15 years. So I represented large corporate clients like Best Buy, I did a lot of their commercial litigation. I represent victims of Bernie Madoff. I sued JP Morgan over the mortgage backed security crisis of 2008. So I did a lot of big litigation. But I've also been a coder since 1985. So I have the law Plus technology background. So in 2015, I pitched Thomson Reuters. I said, Here's legal tech that I think could change the world, you should build it and hire me. And they were dumb enough to do that. So I worked for them for a couple of years with about 100 programmers and 50 lawyers building this big thing. And then I left that really cool job to be able to then do my next cool job where I did cybersecurity. And the biggest thing that I worked for a company called stress Freedberg stress. Freedberg was hired by Facebook to investigate Cambridge Analytica. So I spent about a year of my life on Facebook's campus with Facebook's data scientists and my former FBI, CIA, NSA people that worked with me to figure out how bad guys use Facebook data. I did that Monday through Friday, I would fly out of Minnesota on a Sunday night and come back on a Friday, about 50 to 57 weeks in a row. It's all a blur. But while I was doing that, I finished a 14 hour day at Facebook's campus. And then I was at a hotel and I talked to my talk to my colleague, Noah. And I said, Noah, you know how we can brute force passwords by going a B, a C? He said yeah, I said, what if we could do that with music? What if we could go who don't obey don't remain refer until we mathematically exhaust every melody that's ever been and every melody that ever can be mathematically? And he said, Oh, yeah, we should totally do that. So that could that created the all the music project. To date, we've made 471 billion melodies that exhausts every melody that's ever been and every minute that ever can be. And then we've written them to disk. And once they're written to disk, they are copyrighted automatically. What we did after that is we put them on the public domain to be able to help you stole my melody lawsuit defendants like Ed Sheeran, like dua, Lipa, like Katy Perry did a TED talk on that that's been seen 2 million times. Everybody before that has been losing. You stole my melody, lawsuits, defendants, and now everybody asked as one. But then after that gig, I've did my current gig where I'm currently with fastcase and docket alarm and we just merged with a company called V Lex. My dataset right now is about 1 billion with a B judicially filed documents and lawyer filed documents where my job is to help extract the DNA of the law from those documents. So things like motion to dismiss for breach of contract in the Southern District of New York before Judge Smith And the idea is that I can tell you today that there's a 53% chance of winning in front of this judge. And what we're building is we're extracting all the arguments and all of the citations from those to be able to say, perhaps, if you make these arguments, your odds move from 53% to maybe 75%. So essentially, to be able to Moneyball the law to be able to make better arguments and get better outcomes.
Justin Grammens 5:23
Yeah, that's awesome, man. Yeah, very, very cool. Well, so, boy, lots of questions kind of coming on my head here, as you were sort of talking but you know, when you mentioned a melody, how many notes are you talking about here? I mean, you're right. There has to be some some some end, right. Yeah. So
Damien Riehl 5:34
there's, there's the x axis and the Y axis. So you can think about the y axis Doremi Faso? Lotito, that's eight, eight notes that way. And then you might think how many repeated notes makes a melody? And so what I did is I took all of the lawsuits that related to melody, and I said, what's most of the things being sued about, and most of the lawsuits over the melody are 12 repeated notes. So my dataset number one had dodo, dodo dodo the dodo 12 times, right. And then the next one is dodo, dodo Ray. And then the next one, so you just march through mathematically at a rate of about 300,000 melodies per second. And then that just writes all the melodies.
Justin Grammens 6:08
And you have audio records of this, I could sit there and listen to one of these things, or is it just
Damien Riehl 6:12
yeah, they're all in MIDI. Of course, MIDI is much more compact than the wav files or something else. So yeah, so we have all those. And there's a tarball, on the internet, an archive of the first data set of 68 billion, and then the second data set of 68 billion. And today, we've done about 471, that if you wanted to listen to 471 billion melodies, it might take a year, the rest of your life, but you could do it.
Justin Grammens 6:30
Yeah, that's crazy. Very cool. Very cool. And in fact, I was gonna say that, you know, will have links off to your TED Talk and links off to your website, and I'll get the link from you for this tarball. So that's fascinating, really, really cool stuff. Now you're talking about extracting DNA out of a legal documents, it's one thing to say that you do it, but then you have to have an interface to sort of be able to get that information for the Layperson. Right? You guys building chat into this? I know you've been working a lot with Chet GPT and stuff in your, in your career?
Damien Riehl 6:57
That's right. So yeah, we are currently, of course, large language models are really transformative in natural language processing. And the law is completely words, right? That's, that's all the lie is just a bunch of words. So we're using largest language models frequently. But of course, as your listeners probably know, there's this debate between symbolic AI and neural nets, or other types of more ml systems. And so a lot of this work can be done actually through symbolic AI. So for example, you know, a motion to dismiss for breach of contract, how many ways are there in the law to express breach of contract, there's only one breach within two of contracts. So you could actually do a pooling query to be able to find breaches of contract. So all of that's to say that there's a lot of symbolic AI that we've done. And the benefit of symbolic AI is historically has had better precision and recall, then on the ml or neural net side, but anyway, so a lot of symbolic AI, and then we're essentially taking the long tail, and then putting ml and the neural nets and large language models to help that.
Justin Grammens 7:55
Excellent. That's cool. So how long has this company been been in existence?
Damien Riehl 7:59
fastcase was founded in 1999. So the idea was to democratize the lob. So we were 2020 year old startup 23 year old startup that was competing against Thomson Reuters and against Lexus. And just this two weeks ago, three weeks ago, we merged with a company called V Lex, which is worldwide. So they are based in Barcelona, in the UK, in Central America, and South America, etc. So my US dataset is 750 million judicial documents and more about documents. And with the less now we have well over a billion documents. That's cool.
Justin Grammens 8:26
I actually worked at Thomson Reuters from 2001 to 2006. So just thinking about that. So I think before your time, then
Damien Riehl 8:32
that is yeah, a decade before me. Yeah, sure,
Justin Grammens 8:35
sure. But they've got a lot of documents, I will tell you, that's basically what we worked on.
Damien Riehl 8:39
That's right. And when I left the practice of law, I thought, you know, as a data science matter if data is the new oil, I want to go to the place that has a lot of oil, and TR has lots of oil, Alexis has lots of oil. And now fastcase V Lex actually has a bigger dataset than Thomson Reuters and Lexus. I think we worldwide have more data than they do.
Justin Grammens 8:57
So data, yeah, you need this, you need the data in order to train this, that's kind of key to this whole, this whole system to work.
Damien Riehl 9:04
I've been thinking a lot about how large language models affect the business model of word natural language processing. And if you think about data is oil, the refining of that oil through large language models used to be really expensive, were used to have the humans have to tag up the refining to be able to figure out what matters. But now the refinery through large language models is getting close to zero. Right? You with large language models you have, you know, how many GPT 3.5 Turbo things can you do? You know, it's like a fraction of a penny 4000 queries. And then you have things like dolly DL L L, y version two, that is completely free and open source. It's Apache. Anyway. So thinking about the there's the oil that's valuable. And then there's the refining of the oil, which the price of that is going next to zero. So then we as data scientists, okay, now that refinery is zero, really, we have to decide is it going to be gasoline, or is it going to be jet fuel, or is it going to be plastic? And if it's going to be plastic? What To the plastic products that are coming out the other side. And so really, I think that as, as data scientists and as product people, I consider myself with a foot in each, I think that we have to think about what is the product coming out. And now that product is becoming all the more important.
Justin Grammens 10:13
I mean, I guess why now is a question I like to ask, you know, people that are on the show. I mean, we've been talking about large language models for a long time. And we have people that are have got all sorts of experience or some people that listen to this that know what a large language model is. But there's some people that don't but yeah, I'm just curious to know what your take is, is why here in 2023, is it sort of exploded,
Damien Riehl 10:32
I assume that's just the hardware is catching up. I read GPUs are just becoming so powerful. And I think that, of course, open AI has spent the money to be able to run those computations to be able to, you know, just essentially throw spaghetti at the wall and realize, wow, there's some emerging qualities that are amazing. So it wouldn't have been possible without the GPUs being as powerful as they are and wouldn't be possible without the money backing open ai, ai. And of course, that happens from Microsoft investing billions of dollars into them. So anyway, so that's, that's probably the emergence of those two things, GPU speed, and then just a lot of money to be able to throw spaghetti at the wall that I think was why we're here today.
Justin Grammens 11:05
You know, you mentioned about law just being taxed, which I which I totally agree. But then as I was thinking in my head, I'm like, well, but patents have a lot of diagrams and stuff. Are you guys looking at that as well at your at your company?
Damien Riehl 11:15
That's a really good point. And of course, the new GPT the date that we're it's currently April 2023, is now multi multimodal, right, at least GPT. Four is able to take in both text and images. But there's such a low hanging fruit with text right now in the law, that that's really the focus that we're there's so many things to tag up. And so we're tagging those things up first, and by the time we get around to the patents and images, of course, the tech will be even better on that side.
Justin Grammens 11:38
Cool. Well, what's a day in the life of a person in your role,
Damien Riehl 11:41
ultimately, we're at Thomson Reuters, I was a product person that is I would work with the technology people to be able to work through at fastcase, docket alarm, NaVi Lexa, kind of straddle product, I also do a bit of r&d, where I could go through your 10,000 rows of spreadsheets to see if that it's actually working the way it should be. And if not suggesting ways to be able to help make it better. So part product part r&d, and then part I guess, evangelization, where I speak on topics like this to be able to reach out and you know, speaking at many bars, speaking at, you know, various conferences, where I'm able to say, here's all the cool stuff that I'm building with my team, and also talking to customers to say the customer say, oh, wouldn't it be great if you could do x, and then I run back to the product people and back to the r&d people to say, Hey, we should build this because there's a lot of customer demand. Because I'm a tech person that can put words together that also has good product instincts. I wear a lot of those hats. I'm just
Justin Grammens 12:31
kind of laughing a little bit, because I'm a tech person, too. But I'm also the CEO of my company. And I you know, I'm also one, I do a lot of leadership events and stuff like that. But it's it always pulling me back down into the tech, right? I'm always like, playing around with Jupyter notebooks and figuring out well, how is this? How does the transformer model work and stuff like that? Do you find yourself in that same thing? Maybe you go a little too deep?
Damien Riehl 12:52
It? Absolutely. And I have found, you know, I've been coding since 1985. But I'm a horrible coder. So I would say that, you know what, I find myself going too deep, I realized that I should stay in my lane and do a lot of things that are I'm really good at. And coding is not one of those things. But I will say that with GBT four and the other models that are doing a really good job that if I give English input to say, make me an x, and you make me code to do X, and it actually doesn't, I think that's you know, catnip to bad coders like me that can actually bake it, rather than being able to know what I'm doing.
Justin Grammens 13:23
For sure. So are you are you guys building your own large language models here at this company or your own proprietary thing you writing on top of something else.
Damien Riehl 13:30
So I'm hesitant to say too much about what we're doing internally while we're building it. But you can imagine there's two ways you could be able to, you know, hop on to the GPT portraying or GPT five whenever it comes out. That's option number one. Option number two is to use like an open source model, say a Dali to or others to be able to say, you know, I don't want to have to spend the GPT dollars, I just want to be able to get a good enough through Dali, that's thing number two, way number three is actually some researchers in out of NYU, Stanford and MIT are building a large language model, foundational model based on the law. So rather than ingesting from Twitter or from Reddit, like the others that are presumably based on, they would be ingesting just statutes, and just judicial opinions, and just regulations. So that foundational model would just know how the law works. Then on top of that, you could be able to build all sorts of things. So these NYU Stanford and other researchers are building this foundational model that they plan to open source. So anyway, so that would be option number three, either could use GPT as option one Dali or something equivalent to the option two or this foundational models, option three. And by the time our podcast gets to here, there's probably going to be options. 5677.
Justin Grammens 14:35
Sure, sure. So a lot of people are training these foundational models in certain sectors. So they're, they're really, really good at specific things. Bloomberg
Damien Riehl 14:42
finance, for example, they did a large language model that is foundational model on finance.
Justin Grammens 14:46
Yeah, yeah, sure. It kind of begs the question of like, you know, do we have general artificial intelligence yet? Right. I personally think we're very, very far away from that. But people use chat GPT and they think that this thing knows everything, right? I think there's
Damien Riehl 14:59
a lot lot of psychology involved with the thinking that it knows everything. And there's the you know, of course, everyone talks about hallucination problems. So that's not really, really any spark of insight. But I would say that the hallucination problem probably is going to be a blip in the radar where, for example, you know, option one is to say, GPT, give me output, and then it spits out output. Option two is to say, GBT, here's a query of 50 documents, give me the things that are statistically most likely to happen in those 50 documents. So that's kind of a rifle shot in the summary of those things. And the actual rate of hallucination for that is really slim. There's very few. And so really, I think that yeah, hallucinations are probably going to shrink. And as part of that, you know, artificial general intelligence, I agree, are pretty far away. But yeah, there's, there's really a big question as to whether we are there already. I was at Stanford just two weeks ago, and somebody who spoke that used to be on the open AI team thinks that we are at the singularity at this point, we just don't know it. Or at least we don't acknowledge it, that a lot of our actions are algorithmically generated, what we listen to what our decisions on any given day, is that not the machine actually doing telling us what to do? And in a sense, you know what, to watch what to listen to? Who who to believe, right? Are we already at the singularity is the question and then from there, you can go to AGI.
Justin Grammens 16:13
That's true. That's a good point. It's a good point. Yeah. I mean, I don't use Google Maps anymore. I mean, sorry, I don't I don't drive anywhere. I always put it in Google Maps. Right. So yeah, it's telling me where to go all the time, for sure. It's right.
Damien Riehl 16:24
And we and we trust Google News to be able to say, okay, these are the headlines, right? And we trust Twitter to be able to say, this is the things I should be caring about. So of course, I don't think that an AGI is controlling all of those things to be able to make us a ton of buttons. Maybe and I just don't know, right.
Justin Grammens 16:36
Sure. And you mentioned about GPT, five, and six or whatever, as you were talking I mean, what's what's your what's your thinking right now? I mean, there's been this moratorium that they people have talked about, I don't I don't know if it's gonna happen. I don't think it's gonna happen per se, personally, but but like, you know, what, what are some, you know, what's your feeling with regards to sort of the next rev of these models,
Damien Riehl 16:56
if you listen to this as of two weeks ago, so that this might change. But two weeks ago, Sam Altman, I think said that the era of large language models getting bigger, as stopped GPT, five probably isn't going to be bigger than GPT. For, at least that's, you know, that might be marketing, and might be, you know, trying to keep his competitors at bay. But if you take it out its word. Maybe it's that, you know, there are only so many human language sources that can be ingested. And so maybe we've maybe gotten close to the apex of that. And so now we just have to take the human sources that we have left and be able to fine tune that in to be able to make it better, faster, stronger. That's thing number one. Thing number two, is as we reach this kind of post truth world, where we really have to wonder what is truth, what is not truth? And of course, open AI is ingesting all the things that you are either true or not true. What is what's some of the last bastions of human written things that have some semblance of truth? And I would say that judicial documents are those judges are literally in the law called fact finders, they find facts, and how do they find facts, they often spend years in litigation with conflicting evidence, and then the adversary proceeding to be able to determine what is a fact and what is not a fact. And then they produce documents called judicial opinions that provide those facts. And so we have court cases all over the world every day. And that is an never ending supply of human written things that are literally factually true. That's not Reddit, right? That's not Twitter. That's not the BS that is being thrown into the world. But these are human created things that are truly human created, at least until the judges make AI decisions. But that's not gonna be for a while, hopefully. But this could be a source of factual, raw data that is human created that maybe could be used as training models going forward.
Justin Grammens 18:37
Oh, that's fascinating. Yeah, yeah. Yeah, for sure. I can definitely see that. Because what I think it and I think you might be referring to, you know, Sam Walton was on the podcast with Lex Friedman. I'm not sure. Yeah, that might have been the one where he was talking about, I was nodding, when I was listening to this, when all the things that he was saying he was he was making like, really good point. And the thing that I was thinking was was, you know, so many people are generate using AI to generate more content that it almost feels like it's a vicious cycle that AI is generating it in. And it's almost like, if you can I tell people, if you couldn't write like a human, you're actually going to stand out. I feel like at some point, I don't know what you think about that. But
Damien Riehl 19:12
I agree. And yeah, I think that, you know, as we have more AI generated text that makes it in the world that then the AI imbibes, that's like a snake eating its own tail. You know, of course, if you think about what a large language model outputs, it's really kind of a smoothing of or an averaging of what is the most common things. And so you're taking output that is smoothed into average, and then essentially ingesting it again, and that's going to make more smooth and more average, more bland data. So when you think about detect GPT, the way that it detects whether it's a generator of human generated is that humans are not smooth, we are jagged, right? That we as we have anomalies, we have randomness in a way that is not smooth. So if detect GPT says if it's random, then it's probably him as smooth then it's probably machines. So anyway, so that's all the way of saying that to write as a human is to write randomly He is to have weird turns of phrases that are rare, at least rarer than the AI output is. And so I think you're right. If we write like a human and do a turn of phrase that has not happened before, that's going to make that language more valuable.
Justin Grammens 20:11
Do you see AI being used then? And, and maybe this is really what you're saying with regards to like litigation like, but even how would I present the case to somebody right to the judge? Right? You see it going that way? Are you guys touching on any of that? I don't, I'm sort of thinking out of the box here.
Damien Riehl 20:25
Yeah, no, that's That's exactly right. So I can right now give you for judge Smith, I can show you here are the 98 cases where judge Smith granted a motion for summary judgment to dismiss the case. Okay, I can now take those data, that is the 98 documents. And I can run them through a large language model to say, okay, what are now the arguments and the facts that are statistically most likely to win for that judge for that jurisdiction for that cause of action? And then you can take that microcosm of that judge and just say, Okay, for this district, the District of Minnesota, or for this country into the United States, what are the facts that are likely to win? And what are the scenarios that are legal scenarios, that is the cause of action that are most likely to win. So now, these are all quantifiable things. And these are all things that are being counted by a nonprofit that I'm helping lead called Sally. Sally is a nonprofit that's being used by Thomson Reuters, by Lexus by managed by NetDocuments. And it's collecting things like motion to dismiss breach of contract and District of Southern District of New York. And so once you collect all those data, then you're going to be able to say, Okay, now give me winning motions in this district. And then you could be able to run analyses across those in a linguistic way. And with large language models, the refining of that data that is in using the oil analogy, taking those arguments out there is getting close to zero these days.
Justin Grammens 21:45
Could you see an a leveling up basis, because the other side's doing the same thing? Right?
Damien Riehl 21:49
With regard to percent? Yeah. So I mean, yeah, so I'm a plaintiff, I'm going to make this statistically likely arguments there. And then the defendants will do that. And then the poor judge, right has to decide between these two algorithms and really pushed up things. So I'm going to be speaking with a bunch of federal judges, and a few months here, and I'm going to be saying, Not only is this kind of arms race of arguments that we just described, is going to be happening, therefore, you might need some AI to be able to counteract that. But similarly, you know, it's pro se pro se means unrepresented. People that can't afford a lawyer, all of a sudden can say to GPT, draft a complaint about my landlord that does bad thing to me, right. And I don't have to pay any lawyer for that I pay the $20 per month subscription. And all sudden the courts are inundated with these lawsuits that hadn't actually happened before. There's a big access to justice problem in the United States right now that 80% of humans that want a lawyer can't afford a lawyer. So there's an 80%, unmet need of people. If GPT feels that 80% That means that the courts are going to be inundated. Because those 80% can do that for next to $0. And then the courts are going to need a way to be able to you know, they're already backed up, the courts are already got shutter. So it's even going to be more backed up with the innovation of 80% that now can afford it. And also the kind of arms race of the people that can't afford it getting the better arguments that require more judicial thinking to be able to say who wins. So between those two, you have to fight AI fire with AI fire. And I think that's our future.
Justin Grammens 23:17
Wow. Yeah, I hadn't thought about that. I mean, there's, there's the flip side of that being like, Well, great. Now people can use this tool as a democratization people that couldn't afford a lawyer now they can actually do something just like I'm not a great copywriter. But yet I can use chat up to write on my copy for me. But there's there's a downside of that, then it's just going to be flooded. And do you see then lawyers being a eyes? Maybe I mean, sorry, judges being AIS, right? You might actually not have a human behind the scenes. And maybe we're decades away from that. But I mean, I always think about AI as a great way to enable humans to be super humans. Right? Right, take take out a lot of the monotonous work. And so we either need to hire more judges, it sounds like or bring AI into the equation.
Damien Riehl 23:56
I would say that to answer the question, I worked for two judges, one a federal judge and the state court judge. And I would say I hope that day never comes where there is a non human judge. Because I think that part of being a judge is realizing the humanity of the person that's in front of you. And I would say both my judges that I worked for, definitely saw the humanity and there is data you can collect. And there's data that you cannot collect. When I was working at Facebook, there was a poster attributed to Einstein, but who knows who said it but that said, not everything that counts can be counted. And not everything that can be counted, counts, this goes to judges as humans, we need to be able to say, you know, you can't quantify everything that counts that as you can't be able to look at the the tear trickling down this dependent sizing, they were really contrived versus the the person that say sitting stone faced here like they are not contrite at all, they would do it again tomorrow, right? So each of those things is it's hard to collect data on those things, but I'm really glad we have human judges to do that. That said, there are also tasks that those humans do that could be programmatically automated. That is, you know, it could be you could imagine for any given claim. So if you and I have an argument as to whether you breached my contract or not, you have to satisfy four elements. Number one, is there a contract or not? Number two, did you breach that contract? Number three were their damages. Right? Number four, what are the remedies of those damages? Right. So if you don't prove one of those elements, that case goes away. Sure, right. So you can imagine a judge a human judge, using an AI tool to be able to go through the pleadings and say, yeah, they're missing number two, right, that number two doesn't exist. So I don't even have to think anymore, that claim goes away. And that is humans still have to do QA for that, to be able to make sure that that's true. But really, the AI can help make me faster, better, stronger.
Justin Grammens 25:39
Perfect. No, I love it. I love it. And I think, you know, you said you don't want to see the day when judges, you know, aren't human, I would say probably the same way for doctors, right? Health care, I don't feel like that's a profession where you can just say, Well, geez, now this doctor can do a better, you know, diagnosis than than a, you know, RNA, I can do something better than a doctor, human doctor, because there's a whole different side to it. You're talking about the emotional side, right?
Damien Riehl 26:04
100%. And really, that people have asked if AI is going to replace lawyers, and I say, you know, a lot of tasks, yes. But there's a humanity to being a lawyer that really goes to back to lawyers are called counselors. That is we are counseling. We are human counselors to be able to, you know, people are in hard times when they have to go to a lawyer, right? They're in divorces, they're dealing with probate with their parents death or something. So they truly need a human touch to be able to help them be, you know, get through the times that they're going through. And so yes, lawyers need to be humans. Doctors need to be humans. And judges need to be human. You could imagine there are enough people that are jaded saying, yeah, that Judge railroaded me. Right. I'm in prison because that Judge railroader V. Imagine how bad that would be if they say the algorithm that Google made railroaded me, right, the algorithm that whatever company made railroaded me, we need the human to look that person in the eye and say, you and I both understand that you did something wrong, you need to pay the penalty for that. And that needs to be a human and not a robot.
Justin Grammens 26:56
Cool. Very good. Very good. I love it. I was curious about and I should probably know this. But you know, if you're if I'm using charged up to write code, for example, who owns that? Am I Am I can I go ahead and open source that thing? Can I keep it closed? Do you have any idea? I mean, where does the law stand on that?
Damien Riehl 27:11
I happen to be a copyright lawyer. So we I have lots of thoughts on this. There are two questions on the copyright question. One is what is the copyright ability of the large language model ingesting a lot of text that is ingesting all the books, investing all the internet, all of those are copyrighted, right? Eight ingesting all the artwork, ingesting all the music is all that legal. So that's on the input side. And then the output side is what you asked about right day, what is the output is it copyrightable or not? I'm going to talk about the input. First, the input side, there is a case, it's about Google Books. And it's called Authors Guild. It's out of the Second Circuit. What Authors Guild did is said, Hey, Google Books, you ingested all of the books that have ever been created. Turns out all of those are copyrighted, or most of those copyrighted. So was that infringement to be able to ingest those into Google Books. And the Second Circuit said, No, that is transformative use that is fair use. And the argument that was made was that Google didn't use it to be able to say, you, as a user want to read that book, that was not the purpose, the purpose was instead to be able to take an index of that book. So then you could search all the books to be able to then get a search list of those books. So because it is an indexing and not a full reproduction, the court said that is fair use, that is transformative use, that is therefore not copyright infringement. That is the second circuit. And that was about a decade ago. So if you think about that as precedent, and by the way, Google Books will display depending on it, maybe three or four pages verbatim, and those books, right. And if that is fair use, think about what large language models do. What large volumes bottle do is extract the text from it. And they don't actually extract the text, all they do is to say, what is the statistical likelihood of this word, following the next word, following the next word. And once they have extracted that mathematical model from that particular source text, they jettison the source text, now they don't do it anymore, right. So so really, the source tech goes out the window. And so then when you think about the output, the output isn't. And I should also say that in the copyright law, there's a difference between the idea and the expression of the idea. So the idea is not copyrightable. Only the expression of the idea is copyrightable. So when that loads language model is going through the text and extracting the statistical likelihood of this word following that word, it figures out where items are in vector space. So it figures out where that a car is similar vector space as automobile is similar vector space as van, etc. So all those kind of live in the same area. All of those are just ideas, car, van, you know, automobile, those are all ideas, the expressions of the ideas, those goes out the window, because you don't need it. All you need is the model of the ideas. So when you then think about, okay, as the input, you're not doing the Google Books thing of ingesting the expressions, all you're doing is extracting the ideas from those things. And then as the output, it's not doing expressions of ideas, it's just doing the ideas themselves and reconstituting new expressions that are not based on the old expressions. And if they are similar to the old expressions. That's only because those old expressions are so trite and common. So on the inputs, you're taking ideas, jettison the expression, and then outputting the ideas in new expressions. So I would say that both of those, if I were Microsoft lawyers in the open AI, and GitHub case, the copilot case, I guess the argument I would be making is that these are mere ideas passing through that are on copyrightable. And then to your question as to the output is the output copyrightable. The US Copyright Office has very firmly said that if machine created then on copyrightable And so really, they would say that if the machine open AI is GPT, puts out content that I would say that is not copyrightable. At least according to the US Copyright Office.
Justin Grammens 30:40
Gotcha. It should be open and free domain, I guess.
Damien Riehl 30:43
Well, until until somebody changes laws otherwise, yeah, there's real questions as to if I do a prompt that say write me a song and the style of Bob Dylan, is that infringing Bob Dylan? And using our previous analogy, right, when the large language model goes through words about Dylan, it's not actually taking the expressions of Bob Dylan is taking the ideas of Bob Dylan, Alice, and Bob Dylan lives somewhere in vector space in that 12,000 dimensional vector space. Right? And so it takes those ideas, and then outputs the idea, Bob Dylan, is that any different than you or me saying, I'm gonna write a song in the style of Bob Dylan? Right, right. If I if you're I did that Dylan can sue us, because how many singer songwriters from the 1970s? Sounded like Bob Dylan? I would say all of them. And he couldn't sue anybody. Right? So if a machine does something in the style of Bob Dylan, is that any different because Bob Dylan, this is just an idea, not an expression of that idea. If I take a cover song of Lady lady lay, or you know, some some other Bob Dylan song, that expression is copyrighted. But if I write a song in the style of Bob Dylan, that doesn't take any expression away. That seems like that's fair game.
Justin Grammens 31:47
Good point. Good point. Yeah. And you talked about going through use of Google Books, right? I think the same theory applies if you're actually going through GitHub, for example, right? I mean, somebody could point out that and say, hey, you know, the language model, essentially stole my code, right? That ended up writing it. But at the end of the day, it's just still taking the expression that codec generating,
Damien Riehl 32:07
I think the real question is, you maybe if you put those two side by side, you say, look how similar they are, right? But then really, that's not the whole story. Because you'd have to take the entire corpus of GitHub and say, How often does that string show up? If the answer is 10,000? Times? How original is your string system? And the same thing with music? That song sounds this song sounds like that song, cool. Hear the 20 other songs, or the 50? Other songs or the 100? Other songs that also sound very similar? So really, the question is not Are they similar? But the question is, are they original left to be copyrightable? Or are they mere ideas or lingua franca? In the courts? They call that Swansea fair, that means like, you know, if you want to write a Western, there's a guy in a white hat, and then there's guy in a black hat, that's just the way it is, you can't copyright those things. So that's just sounds a fair. If you write code, and you're writing a JavaScript function, this is just the way it's done. Right? You don't get copyright in that.
Justin Grammens 32:57
Yeah, sure. That's great. That's great. Well, good. I love it. I love asking these questions, because they're so far out of my domain, I have no idea. Like you said, I think the law is also kind of changing along the way too. So it's good to have somebody who's sort of up on that, you know, if somebody was just coming out of school today, for example, or just getting into the field, maybe they would make a career change? Or maybe there happened, and where do you suggest they kind of go to sort of get the update information? Where should they learn from? What are some suggestions that you would have as a mentor?
Damien Riehl 33:22
That's a good question, I would say that, you know, learn by doing is probably the best way to that I would recommend that is you know, take Coursera courses, you know, just build these large language models, be able to, you know, code and be able to build. And so I would say that, number one learn by doing number two, probably, you know, I have an RSS feed through Feedly. To be able to say here are trusted sources that if I want to know what's happening in large language models, or I want to happen in in the legal tech space, I have a list of about 150 sources that I trust that will be keeping my well fresh, if you will, of all the things that are happening. So make sure that you have those trusted sources. And if you can keep them in an RSS feed like thing like Feedly, that you don't miss things, I would say that those are the two things I would say, you know, be able to do rather than learn. And also to keep up with the latest developments.
Justin Grammens 34:07
Great suggestions, for sure. Well, good, I will definitely put all of these links, everything you've talked about in our liner notes, we do a full transcription of the whole conversation too, as well. So people can go back and find those, but I'll make sure that they're listed in there. Where can people get a hold of you?
Damien Riehl 34:20
I'm on Twitter at at first name, last name. So that's at Damien real. I'm also on LinkedIn. I like talking to people on both,
Justin Grammens 34:27
right, perfect. Well, is there anything else you want to say? I mean, anything we didn't cover here or anything? Any questions, maybe I didn't ask that you wanted to?
Damien Riehl 34:33
I would say that we are at a strange and exciting and terrifying point in history with large language models. And in you know, AI, of course, and data scientists have a leading role in figuring out what our future is going to be and these strange and terrifying times. And so I would say that, you know, we as coders, and we as lawyers, all we do is symbolic representations. That is lawyers just do words and coders just do code each of those are symbolic representations that large language models do really well at replicating what we as humans do. So as we think about what is our future, we should be thinking about what can we do that the machines can't yet do. And then we should chase those things. Because think of it like a tidal wave that the tidal waves come in, right, you have to run ahead of that tidal wave. But eventually that tidal wave is going to beat us. But we at least have a head start to have some runway to say, what can the machine not beat us at yet, chase those things. And that's what I'm doing as a lawyer, what I'm suggesting people to do as a lawyer, but you know, eventually we as data scientists are going to be helping the tidal wave, take, take more of humanity, because we're building these systems. So we should really be thinking about, you know, if GPT and large language models can write code today, what can I do to be able to build a top that that the machine can't yet do? And maybe we have five or 10 years where we can actually Chase ahead of that tidal wave before they comes crashing down on us?
Justin Grammens 35:56
Sure. Well, I think it's going to cause a leveling up, you know, people are just going to have to get better at what they do. And they're going to have to be more creative. And I, I view all of this stuff as a tool, you know, that it's, you know, you talk about crashing down, I actually think it could be something where it, maybe you're surfing, right, and then it it actually gives you an accelerant, you know, going forward, I guess I'm hoping I hope that's
Damien Riehl 36:17
true. And you know, you think about there's two kinds of futures in this that a friend of mine, as a coder that thinks that copilot gives them either a 10x, or 100x improvement on his code, he could do it 10 times or 100 times faster, better, stronger than he could before. So let's say 10x, let's say if a 10x is u. So now can I work 10% of the time to get 100% of my current output. That's maybe option number one, maybe option number two is that my employer is going to require me to work 100% of time for essentially 1,000% output of what I currently do, right? So that's, that's maybe option two, maybe option number three is I work 50% of the time to get 125 or 150, or whatever percent, and then they just fire a whole bunch of people. Right? And that and then to be able to show that their shareholders say that, hey, look, we're getting more output. And we're also saving costs by firing people. Right? That's maybe one of our futures. So really, I think the question as to whether we're crashing down on us, or whether we're surfing is what the employers of the world do they decide do we require, you know, 10x on top of the 100%? If so, then we're going to be surfing. Right? Yeah. Or if they say, you know, what, what percentage below that and how much layoffs are we going to be dealing with?
Justin Grammens 37:24
For sure? Well, in any case, it's going to have an impact for sure. I would 100% agree. I think both of us could agree on that. And it's because the impacts coming
Damien Riehl 37:32
100% agree. Yeah, the buckle up. It's gonna be weird. Well, great, Damon, I
Justin Grammens 37:35
appreciate your time, wholeheartedly. Thank you so much for sharing with our listeners, all of your experience. It's really great to have somebody with such a diverse background here that can sort of talk the tech but also knows the legal world. So good luck to you and your future. It sounds like you guys have just a really a lot of awesome things going on it fast case and we look forward to keeping in touch with you in the future.
Damien Riehl 37:54
And thank you so much for having me on. I really appreciate it.
AI Announcer 37:58
You've listened to another episode of the conversations on applied AI podcast. We hope you are eager to learn more about applying artificial intelligence and deep learning within your organization. You can visit us at applied ai.mn To keep up to date on our events and connect with our amazing community. Please don't hesitate to reach out to Justin at applied ai.mn If you are interested in participating in a future episode. Thank you for listening