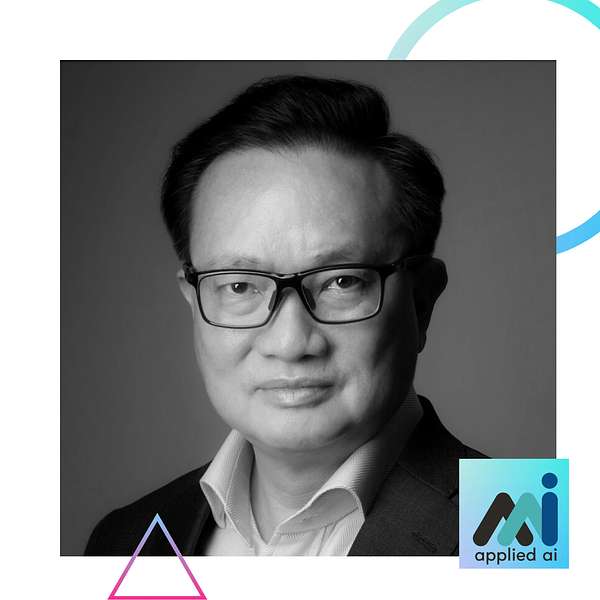
Conversations on Applied AI
Welcome to the Conversations on Applied AI Podcast where Justin Grammens and the team at Emerging Technologies North talk with experts in the fields of Artificial Intelligence and Deep Learning. In each episode, we cut through the hype and dive into how these technologies are being applied to real-world problems today. We hope that you find this episode educational and applicable to your industry and connect with us to learn more about our organization at AppliedAI.MN. Enjoy!
Conversations on Applied AI
Christopher Nguyen - Skillsets and the Foundations of Industrial AI
The conversation this week is with Christopher Nguyen. Christopher is CEO and co-founder of Aitomatic, where he's helping businesses harness the power of knowledge-first AI for solutions in predictive maintenance, energy optimization, demand forecasting, and more. Outside of his deep experience in AI, his background includes strategic executive leadership, hands-on software engineering, management, and highly available low-latency services architecture.
If you are interested in learning about how AI is being applied across multiple industries, be sure to join us at a future AppliedAI Monthly meetup and help support us so we can make future Emerging Technologies North non-profit events!
Resources and Topics Mentioned in this Episode
- Aitomatic
- Atomic Layer Deposition
- Knowledge transfer
- Large language model
- Augmented Intelligence
- Maxwell's equations
- K1st World 2023
- Useful Sensors Inc.
Enjoy!
Your host,
Justin Grammens
Christopher Nguyen 0:00
The challenge for us hiring in Silicon Valley is that, for the most part, you know, people have gone completely computer science. And I'm partly responsible for that, right? Certainly that is very valuable. But for the kinds of things that we do, you know, this this brothy that some people refer to as industrial AI read the industrial economy. We really need that foundation in physics, electro engineering and mechanical engineering. And so, actually, what I like to say is when I talked to some of my friends, that are mechanical engineers, I said, you know, it's coming back, right, you guys are gonna be coming hot.
AI Announcer 0:36
Welcome to the conversations on Applied AI podcast where Justin Grammens and the team at emerging technologies North talk with experts in the fields of artificial intelligence and deep learning. In each episode, we cut through the hype and dive into how these technologies are being applied to real-world problems today. We hope that you find this episode educational and applicable to your industry and connect with us to learn more about our organization at appliedai.mn. Enjoy.
Justin Grammens 1:06
Welcome, everyone to the conversations on applied AI Podcast. Today we're talking with Christopher Nguyen. Christopher is CEO and co founder of Aitomatic, where he's helping businesses harness the power of knowledge first AI for solutions in predictive maintenance, energy optimization, demand forecasting and more. Outside of his deep experience in AI, his background includes strategic executive leadership, hands on software engineering, management, and highly available low latency services architecture. Thank you, Christopher, for being on the applied AI podcast today.
Christopher Nguyen 1:36
Justin, thank you for having me here.
Justin Grammens 1:38
Well, good. I know you're doing a lot of stuff in AI. It's a booming industry, I give a quick background around here. You know, your work with leadership and hands on software engineering management, I know you've probably been in this field for quite some time. But maybe you could give us a short background with regards to your trajectory to how you got to where you are today.
Speaker 1 1:54
Oh, if we start to where I started. I feel the whole podcast just because of the age and you know, I I was a refugee child was dropped in Silicon Valley, San Jose back in 1980. My first PC was iti 99, for a which predated the Atari. I haven't stopped packing since that would be the compact version. But I've I've been fortunate enough to be part of multiple waves of technology all the way from semiconductor, Intel, Xerox, HP, and so on. I've been a professor, I did my undergrad at Berkeley and grad school at Stanford in a Christian earring, of course, it was hard to do just easy. So it's actually ECS. After the PhD, I went off to Asia, Hong Kong, that was a brand new university starting at the time. This is in the early 90s. The Hong Kong University of Science Technology I helped I was one of the earliest founding faculty members there. And I started a department called the computer engineering department there. But then realized that I actually wanted to build a company. So So left and build a couple of companies, again, at the beginning of the internet commercialization, sold those and then came back to the US. And Google was also just starting. So I ended up joining as a director that left again and built another company. And that's my current journey. Right. The interesting thing about what we do today is you think about how Silicon Valley has gotten to, you know, fully digital, my Software is eating the world kind of mantra, this project that I have automatic is about applying machine learning AI to the very physical world, the industrial companies, because my last company was acquired by Panasonic. So that's where I picked up a lot of the industrial AI credentials.
Justin Grammens 3:45
That's awesome, you're speaking to a lot of things that I find fascinating. So my background has been in a lot of Internet of Things started my business about Mauboy, almost 10 years ago or so working in IoT before was even called IoT. But what's been interesting is, is companies have started to put these IoT sensors, stuff in all sorts of places, whether it be in your home in your car, but the industrial spaces is is very unique, right? And now that we're getting this data, now we can start applying a lot of AI and machine learning to it. And you got the perfect background, I was gonna say, you know, computer engineering, those are the types of people that we usually hire out of the local university here, because they have that good sort of mix between excetera understanding the hardware side of it, but then also understanding the software side. So maybe you could touch a little bit on like, how are you guys then positioning your product to be differentiators in the market? Then a company would just you know, maybe say, I'm just gonna buy some off the shelf or I'm just gonna develop my own. Where do you guys sort of sit in that space?
Christopher Nguyen 4:38
I'm gonna put that you know, segue from what you say, which is exactly the directory and put it on on fast forward and go right directly to where I say I don't care how much data you're collecting is not enough. And this is the problem that I ran into when I you know, this would be in 2016 17 When When Panasonic acquired my company, and I helped run the global AI there and we tried to solve a lot of these industrial challenges by mandate was to apply AI right across the global business units. And we ran into this problem very quickly. And it's the problem that I just described. And that is the knowledge that is needed. The information that is needed to solve these physical problems do not wholly come from the sensor data that you have of your IoT, it turns out that you really do need domain expertise, you need people that have been learning the physics of these systems built up experience over 2030 years, the data that you have is sufficient only for certain tasks, but not for many of the real challenges. For example, you take predictive maintenance, predictive maintenance, people talk about that a lot. The real formulation of that problem statement is, can you tell me the probability that this particular hump is going to fail over the next few weeks? Right? Most of what you know of I'm going to call it Silicon Valley predictive maintenance, right, just as a analogy for the digital only approach is actually just half of that it is anomaly detection, right? Meaning it learns enough about the sensor data so that it establishes what is normal operation. And then when there is something that is out of the ordinary, it'll alert, if this is an anomaly. You notice that where I positioned that is only half of the predictive maintenance problem, because that's, that's saying, hey, something looks different today, right? Hey, are you wearing your glasses, or something's different about you? But it's not saying that you're going to get sick? Or exactly, you know, your heart condition or something? Right? It turns out that most predictive maintenance is doing that have to kind of short list of things to look at today. And then throwing it over to a human expert, right? Because a human expert is the one that can make content that has the actual knowledge and information to solve it. So so the holy grail in problem like predictive maintenance today, is how do we automate? How do we codify what the expert knows? How do we automate that? And how do we scale that because the main main business problem really is that these experts have become fewer and fewer in numbers, particularly in the US, right? Where we have essentially ceased making things for about two decades. And now we wanted to bring it back for geopolitical reasons, for economic reasons and so on. But whatever it is, it is a very interesting, exciting challenge. Right? But how do you then, you know, take the few experts that you may or may not have, hopefully, that you do, and you scale their talent across the entire fleet of vehicles? The entire, you know, set of machinery that you have, and you do manufacturing operations.
Justin Grammens 7:48
Yeah. And again, it depends on the industry, right? If you're talking talking about a company that's been around for 50 years that has been doing manufacturing, that they had said, Well, there's people on our floor that can the shop floor or the manufacturing floor, that could just sort of hear lawyers, motors about ready to go. So they're like, how can we capture that and of course, there's a digital side to it, where you sort of watching, you know, the the current draw, but there's a human side to it, where it's just these, these people have that innate knowledge.
Christopher Nguyen 8:16
That's right. And their knowledge. And the reason knowledge is required to make sense is the state of machine learning today is that if you change the model, the engine that you put in there, the data copy is completely different to that machine learning model, it might as well be, you know, something operating on Mars, right? There's no transferability between, you know, one context to another. So then every time that happens, you say, Okay, well go collect more data. And by the way, collect two years worth of data, three years worth of data, well, I changed the model over here, I changed the workload all the time, I changed the case of we also do in refrigeration systems, in supermarkets and so on, the workload is different, the climate is different, all those things are different. And yet the physical intuition and the physics that is in the head of these visuals, should experts, they transcend these different machine models and so on. And so they're able to say, Well, if the pressure has been dropping for two weeks, and yet the temperature has remained the same. I'm going to take a look at the compressor. Right? Yeah. So so they built up the server futuristics over the years. And by the way, you know, semiconductors going back to the US. The air is not a matter of heavy machinery, but precision. There are systems called atomic layer deposition, ALD where you're literally putting down 10 layers of atoms across a wafer surface that is, you know, 12 inch. The precision that is required is we like to say it's technology, it is art and it's also a bit of voodoo. That's
Justin Grammens 9:54
that's some small scale stuff for sure. So, so in that case, you're looking at quality control. quality as
Christopher Nguyen 10:00
measured by consistency across, you know, it's almost like Earth, you care about a height of maybe knee knee deep right as to be completely uniform.
Justin Grammens 10:12
That's an example of a system then that you would come in and help a company using your technology, I guess, to be able to implement quality at that sort of micron level, I guess.
Christopher Nguyen 10:21
Right. And the way we do it is that we don't, you know, we're technology provider in that context, right, we don't actually do the, the ALD physics. So that's the domain of people like Tokyo electron apply materials, or what we help them with is, if you think about the amount of expertise that is required to build machine, they call them tools to build these tools, and then test them, qualify them in volume and then putting them out in the field and then have other experts, but different kinds of experts use these systems, right? So they would sell into the TSMC is, and then the charter semiconductor and so on. How do these two groups of experts communicate with each other? When they are bottlenecked, by time, they are bottlenecked, by the numbers. They're also bottlenecked by intellectual property, right? Like, they're not incentivized to, to, you know, Intel doesn't want to tell ply material that I'm going to, you know, do this many wafers to do that purposes. So, there's sort of a virtual expert in between, where the toolmaker can share all of their knowledge about building this equipment, where that the process user, the process expert, can leverage that knowledge and do their own work. And now, it's much easier to explain what we do, because there's Chet GPT, a year ago when I was talking about this, and people say, Well, there's one group to say, are you building expert systems? And said no, then what kind of magic is this? Right? But but now you can see these large language foundational models can help us translate the unstructured expertise, whether it is written Word documents, or spoken language, into a structured form. And then from that structured form, the other people on the other side, right, can query that can ask questions. Currently, what they do is they would look up these large documents, right? And then find major sections, very slow process to try to find the answer if there's an answer in there at all, but that now they can get much more consultative, right, by us translating this expertise. On the one hand, the other thing they do is they would build models, AI models, in fact, machine learning models that can leverage both the knowledge as well as the data coming off of the tool.
Justin Grammens 12:40
That's super fascinating, because, you know, I was looking at your website, and you know, one of the things you, you, you clearly say is no complex coding required. Right? So you guys are really kind of trying to democratize, I guess the ability for people to do some of these things is something as complex as natural language processing? And that seems like that's probably one of the key differentiators you guys have? Is that? Is that right?
Christopher Nguyen 13:04
That's right. So with respect to the two use cases that I mentioned, explicitly, one is I call it human to human right? How do I transfer my knowledge to you without actually having to require that you book a meeting with me, right? And there's only one of me and hunted the view so that the humans human knowledge transfer and the other sort of the human to model, right, knowledge transfer? How do you share your knowledge? Have it translate into some structured form, and that becomes kind of an API, that the programmers on the other side can say, Oh, we got a call to do that API. And, you know, ask questions, or, and I don't mean just chatting kind of questions. I mean, like, given this pressure level, what is the ideal temperature?
Justin Grammens 13:46
So you guys have an interface like that? You can just Yeah, once the knowledge is gained, then through an API, you can essentially simulate what a human would say in that question that in that, in that situation,
Christopher Nguyen 13:56
and human expert may refer to some physical equation.
Justin Grammens 14:00
Gotcha. That anything, he just said, chat. GPT. Because yeah, I feel like that is opened up people's eyes to be like, Wow, this is this is what's really possible. And so you get scanned the entire internet, right? And get, you know, billions of parameters and whatnot. But do you guys have a plus after platform that we send all of our data into your system? So it can learn it? Or do I train it and then upload the model to you, maybe talk me through a little bit how you'd how it interface will reference
Christopher Nguyen 14:23
GPT? Because now, a lot of people are now familiar with that, right? There's a chat interface. But behind it is this eCola large language model, right LLM? Or generally I call them foundation models, because it may be images, maybe video and everything. Right? So the chat interface is just a user experience. The real I'm going to I'm going to loosely call it knowledge right is in the mind. If you think about carrying that mental model, what open AI and Google and made a build these large language models that leverages say the body of knowledge that It is available on the internet, called out the foundation layer. But then above that, we add domain specific. So for example, we stick with semiconductor when I play with GPT. And I started saying, you know, what do you do about semiconductor and so on? And I said, you know, and now if I, you know, what's the best flow rate for dichlor? On site, ln, if I want maximum absorption, you know, at 600 degrees C, and I say, What the hell are you talking about? Christopher? Right, right. Now it could No, that's what we do. We add domain specific, you know, information. You can think of it as textbooks, industry documents, and so on. So that layer, that model that we'll call fine to model, now know something more about that world than the generic base model. And then, in each company, each situation in that industry, they have their own proprietary institutional knowledge is, right. So they would add that, and that becomes their model. So now you have a model that knows something very specific, but also very general about the industry. And then from there, you can say, you can generate structured information out of that. And that's where the interesting work begins. The reason I say that is everything that I said so far, is in the world of marsh language models. But what most people don't realize who tried to build these things, that's only half, right. The other half is still very much formal architectures of how to combine this knowledge stream with the industry, right? I spent five minutes saying you don't have enough data. But that doesn't mean you don't need it, you do data? How do you combine the knowledge together with the data so that you could make these predictive decisions? I share one example to make that concrete, right. When use case is fishing, I mean, the fishery industry, how does it fish get from the ocean to your to your dining table, there's a whole industry that supports that. So before first is you catch the fish, right? So we work with a company called Felino. To do to do fish classification, through noise is a large, global giant reading technology. What they do is they shoot this, you know, sonar beam sideways into the ocean, straight down, and then the echo that comes back, right, it forms an echo gram that you can print out, you look at the screen, you and I as amateurs looking at that we have no idea what we're looking at. But a fisherman, right with 20 years experience would look at that, combined with whatever is thinking going on in their heads about that. That time of year, that time of day, that location. In fact, they're better than photo engineers, right? Because real engineers don't necessarily have all this all this domain knowledge, they can look at that. And they say that looks like it's cool of macro, right? Or they can say that's your SAM or something like that. Okay, if you try to replicate that, and you do it as a computer vision model. Remember, the computer vision model only has the pixels of that, that does not have the knowledge context that that fisherman has. And again, I don't care how high resolution how many images you, you take, it's just not in there. Right? We can combine what the computer vision model says, based on all the standard machine learning techniques, where you annotate the image, you train it, and so on. And so the data model will have an assessment of what it thinks it's looking at. And in machine learning world, essentially, it says, let's say you're looking at five possible species, it outputs five probability numbers. And the likeliest one is, let's say, macro, based on what what is. Now you have another stream that is coming from domain knowledge. And let's say one piece of knowledge, I'm gonna make it very simple, let's say in Hokkaido, right, this is northern Japan, in November, there is no micro just gonna make that up. So So then this knowledge model is also outputting five species with different probabilities where they will the one corresponding to macro is 0%. Like there's no chance. So you can see now you can sort of combine these things specifically dot product, right? And so no matter what the computer vision model says, I'm sure it's at present macro the human model of the dollars model was saying no chance. Yeah, so So the decision the prediction turns out to be more correct than good what the computer vision model along this I hope that makes sense. Yeah,
Justin Grammens 19:45
no, no, I totally makes sense. And as you were talking, I was trying to remember the name of the TV show it was on the Discovery Channel called the Deadliest Catch. Yeah TV I remember it forever remember that show? But you know, they they had film crews and they were out doing Alaskan, you know, like lobster is what it was. but it was always interesting because a it was so much human intuition, right? They were saying, well, at this time of year, these types of C's, you know, and there's a storm coming. And we know that it's going to push the lobster this way, then it was, you know, the goal was to try and catch the most lobster. But it wasn't a computer vision problem. Yes, you need to identify where they're at. But it was much more, these people had been fishing their entire lives out there. In fact, their parents and the grandparents had been fishing as well. And they've handed down all this knowledge, right? So the captain of the ship really, was the key differentiator on a lot of these things.
Christopher Nguyen 20:33
I like to bring that up. That is it's such a great context, right? Because if you watch that show, you see some of these decisions. Or at least, you know, life and death, or at least you know, livelihoods, right? You call you go home and empty handed?
Justin Grammens 20:49
Yeah, exactly. No, it can, it can seriously be, you know, are you going to have a good year as a company? Are you going to go out of business? So it feels to me like there's this idea of augmented intelligence? Right, I've been hearing more and more people sort of use that term, where people get all worried about my jobs going away, and I'm gonna be everything I'm gonna be, you know, outsource because of this artificial intelligence. But I feel like we're working more in this area of augmentation, I would do you feel that same way? It feels like your product speaks to that.
Christopher Nguyen 21:16
I actually like to say that we're not indeed AI business when the IA business intelligence, augmentation.
Justin Grammens 21:22
Good. Yeah. Well, it's good. Good. I see you guys are hiring. I mean, I want to miss out on your careers page, part of this podcast, obviously, is just to get the word out companies that are growing and stuff, do you have any in particular roles you're looking to fill or
Christopher Nguyen 21:34
So the challenge for us hiring in Silicon Valley? Is that, for the most part, people have gone completely computer science. And I'm partly responsible for that, right? Certainly, that is very valuable. But for the kinds of things that we do, you know, this this broad thing that some people refer to as industrial AI, right, the industrial economy, we really need a foundation in physics, in electro engineering and mechanical engineering, and so on. Actually, what I like to say is, when I talk to some of my friends, that are mechanical engineer, as I said, you know, it's coming back, right, you guys are gonna be coming hot, you know, hard to find. And it's true. Coming back to the semiconductor example, in Silicon Valley today, I should say this with maybe a somber tone, you know, there's, there's been a lot of layoffs, right. And people read about that. And, you know, but you realize it's actually a very specific industry, it's very large industry, that's the computer software industry. If you look at semiconductor, right, talent is so hard to find, that these companies are sort of trying to poach from each other, and you know, vertically through the stack, but also horizontally, and so on. And so the semiconductor guys is suddenly in high demand again, but we're certainly what we're what we're looking for, as we're looking for people with that combination of computer science or, you know, sufficient software engineering, background, but equally important, and I'm not going to say machine learning because that sort of, can can be picked up heart that is very hard to train on the job is sort of foundation of physics, you know, Maxwell's equations and so on, in order to formulate, you have to even emphasize with our customers, right? Yeah. Provide them with solutions.
Justin Grammens 23:17
Yeah, no, that's really good. That's really good. So yeah, if there's any listeners that are listening and want to check it out, you guys have a link right at the top of your page.
Christopher Nguyen 23:25
We gotta go engineers whose existence on elixir engineers that have you know, enough CS to be dangerous. That's what we're looking for. Not the other way around.
Justin Grammens 23:36
Sure, like you said, so many other skills can be taught, you know, you could take courses on machine, that stuff up foundational stuff is what's really important. So what is the day in the life of of your job? Is your CEO and co founder of this business?
Christopher Nguyen 23:47
What do you mean, apart from speakers on podcasts?
Justin Grammens 23:49
Yes, yeah. What's, what's some other fun stuff? You do these podcasts? You know, they're probably not great.
Christopher Nguyen 23:54
Well, there's standard CEO stuff, p&l, you know, talking with customers, visiting customers, fundraising, sometimes half the time as necessary, you know, team building and so on. But I think I think the thing that say across I've built a bunch of companies and teams, and I've been a large scale corporate Exactly. for that. I think that I love about this. And I hope you could sort of feel it as I as I talk about it, right? Is the discovery and discovery while solving somebody's you know, real world problem. I have a team working on on. So I mentioned phishing, I've mentioned semiconductor and so on. Another example is where we're working also with a industrial company on predicting adverse or extreme weather, right for the purpose of pushing that button as they evacuate. You know, it's very clear that in the last decade or so, there have been a lot more extreme weather events. Whether it's here in the US, we call them atmospheric river, you know, elsewhere. They call them linear rain belt that have resulted in floods that are, you know, unprecedented in places that you don't expect, like downtown Seoul, for example was flooded, right? So one project is about trying to, you know, predict that. And of course, you don't want to push the button all the time and cause evacuation of the full city of Seoul. But if you if you don't do it, then the people die. So that's an example where we're the team and I feel very motivated. So while you know, this is actually cooler than inducing people to click on one more ad?
Justin Grammens 25:34
Sure, sure. I love it. I mean, the best jobs that I've had in my career were ones that I felt like we're impacting the world, there's no one size fits all with this, right? You're kind of going into these companies, and having to help them on a unique custom basis. Is that true? Or do you have some sort of basic framework that you can use or stuff like that? Right,
Christopher Nguyen 25:54
so we are a software company. So everything that I've said, the domain specific stuff that doesn't come from us, right, we provide them with this tool. And at the core, what the tool does is enabled is translate translation of, of domain knowledge into the structured form, and then be from that structured form can be queried by other engineers or their software people, or built into a model right and predictive model, that would be much more accurate. Let me put it in a simple way, then then if you did not employ human knowledge. So that's the tool that we have, as a software platform. No doubt, inevitably, as we work with our customers, we pick up, you know, domain knowledge. And so, you know, my own background, for example, I really understand semiconductor. And that's what my PhD was about. So as we deploy this, across the market, there's going to be, you know, we can't boil the ocean. So we're going to develop some vertical relationships with different industries. So that's whether that's semiconductor oil and gas coaching, meaning the refrigerator supply chain, and manufacturing, but there are others that we don't yet engage in, because we don't have the bandwidth.
Justin Grammens 27:08
Yeah, it seems like yeah, there's people are gonna need some hand holding along the way. I mean, we talked about working in some of these specific things, you guys, maybe you guys have a professional services team that maybe helps get people going. Once they understand the tool, they can kind of be off on their own. But that's exactly where I was can stay engaged with them begin with?
Christopher Nguyen 27:24
We call that team, the AI solution engineering team. They work with our product team.
Justin Grammens 27:29
Asha, where do you see your tool, then going in the next three to 510? Whatever years? Well,
Christopher Nguyen 27:35
510 years, hopefully everywhere, right. And the interesting thing is that we are the pioneers in this point of view, at a time when most of Silicon Valley is still saying, you know more data, you know, better data collect better than it's so true, it's necessary is that sufficient, but I have no doubt that others are thinking the same way. And they're intuitively building this direction as well. That reminds me that there's a there's a conference that we helped sponsor manage, called the knowledge first conference, and the first one was in 2022. November one that's coming up is going to be October. And that's where it's kind of a birds of a feather gathering. People that are really interested in industrial AI, have been solving or trying to solve it the different ways. We come and we share ideas and so on. My favorite comment from a gentleman named cheetah Gupta. And he is the head of Global Industrial AI at Hitachi, right, just delvis its offices just down the streets of Cairo here. He says, now that I come here, I realized I don't want to go to any other AI conference. I feel like I'm among my people. The other ones are important too, right. But but they serve completely different purposes, whether it is advancing the state of the art machine learning techniques, or whether it is a trade conference, the the knowledge first world and by the way, the URL is k one S T dot world is is for leadership, in business, and government and industry, and so on to get together and share war stories that mostly a failure up to this point. Right. And hopefully, tool, the software that we're building will enable more successes. Certainly, you know, I want people that share this point of view that you really need to have knowledge, domain knowledge to your data in order to real solve real world physical problems.
Justin Grammens 29:33
Yeah, this is fabulous. I'm looking at the website right now I see I see your face there at the at the first level but you got people I mean, from MIT, Columbia, Stanford,
Christopher Nguyen 29:41
the NSA as well right? You know,
Justin Grammens 29:44
you got you got awesome and and I see there's a guy know Pete Worden from useful sensors. He basically wrote the whole book on tiny mellow yep, yep. He's been on this podcast before actually. And, you know, I teach a class on IoT and machine learning at the at the University of St. Thomas here. In St. Paul, Minnesota, and I actually contacted Pete and see if he would be a guest speaker. And he said yes. Which was awesome. Like the guy that wrote the book on tiny ml actually came in and spoke to my my students via zoom. But I mean, I basically my whole class is all around 20 ml. So man, this this conference looks looks great. So you guys are planning on doing it again in fall? 2023. That's right, October. Perfect. Was this in the Bay Area? Seems like this will be
Christopher Nguyen 30:24
Yeah, the location is Stanford, your Stanford.
Justin Grammens 30:26
Okay. Interesting. Cool. I'm always looking for a good conference. So I will definitely put this definitely put this in the liner notes for other people to see. And yeah, I went to the 20 mile conference last spring, which, which was awesome. But I missed it this spring. So this would be great. Right AI, ended IoT conference, perfect.
Christopher Nguyen 30:45
Pitch spoke at the key first world as well. And he talked about useful sensors. I'm on a mission to make, you know, everything creepy. Meaning, I mean, you know, when your fans start, you know, responding to you. Right? He was wave our hand and TV should turn on and talk to your lipo. Of course, there's beginnings of that already, you know, Google Home and so on. But, but useful sensors about putting all of it into every device everywhere, your environment will start responding to you.
Justin Grammens 31:17
Yeah, running these models at the edge and being able to let them run for years on battery power, right. So that's when things get interesting is when they're not connected to any power anymore. And they can run for a long period of time and respond to stuff, not even over the internet stuff on their own. So
Christopher Nguyen 31:33
you're attached to our fans, right? Because we're anthropomorphize everything.
Justin Grammens 31:38
Well, good. So one of the things I'd like to ask people maybe at the end of the podcast is like, so if I'm a person coming out of school today, where should I be looking for resources to sort of like, learn this stuff? I mean, obviously, there's, there's your company, but I mean, what what advice would you give to college graduates or even people that are studying school today? Obviously, you mentioned computer engineering as a good field. But even if I cut out with a computer engineering degree, what are some interesting pockets or interesting publications? I mean, you mentioned a conference, which is awesome. Where should I be looking?
Christopher Nguyen 32:06
Well, narrowly, you should be looking at automatic. We do take interns or right hand of the distribution. But I think I think generally, the the interesting thing is that it is not about a role or a job. It's about connecting yourself to the new economy versus, you know, versus the old economy. I think it's an exciting time for young people. But it's also a very scary time, right? I have a friend that have kids at CMU, studying computer science Chachi PDK. Well, and that son call his dad as it is, it's been a record we needed any more. Right? I think that's, that's worth thinking about. But I think, you know, related to this knowledge, first, AI or knowledge, first sense that we have, I think humans will always be needed, right? Certainly, with large language models, a lot of things that that are sort of lower skills will become more and more commoditized. But there's always going to be this top executive layer that people say, you know, when these machines can do everything, what is there for humans to do? Well, I said, Yeah, one of our job is that the second function, not just the answers, but prioritizing the answers and choosing among the answers, right, and asking the questions, I think that's going to be even more needed than just doing economy. Right. So so the premium for that is going to be very high.
Justin Grammens 33:36
And that's one of things I've been here I like Chet GPT it's amazing, but you got to prompt it. Right? You have to ask the right prompts, if you don't understand the right questions to ask. It's useless. Right? So yeah, you're gonna need people are gonna have to actually understand, think about where how this technology can be best used, it's not just gonna automatically implement itself. So that's, that's definitely why it's where it's, well, are there other things you want to talk about? Christopher? I think we touched on a lot, a lot of stuff here.
Christopher Nguyen 34:03
I would appreciate if people go to automatic, just like automatic, but starting with AI. So I do aitomatic.com. And check us out, you know, particularly, you know, potential industry leaders that hear this and find that we may offer something of interest or even that the problems that I'm talking about resonates with what you have seen. And by the way, that's that's where the conversations slow the best people who have tried and fail, and so they know exactly what I'm talking about that okay, then tell me about your your solution, your approach?
Justin Grammens 34:35
Yeah, for sure. I mean, you have to expect implementing any new technology, there's going to be a series of there's sort of the Gartner Hype Cycle, right things kind of fall into this trap of just solution. mminton ai has gone through a number of different winters, but I feel like or I don't know everyone that I talked to everyone everyone's been on the podcast is yes, there's been a lot of trials and tribulations and there will continue to be things that don't work well, but it's really starting to pay off here. We're seeing a lot of great applications of it.
Christopher Nguyen 35:02
Well, you mentioned GPT itself was going through his own hype cycle.
Justin Grammens 35:05
Yes. I think it's actually overhyped right now. Right. It's everyone says it's going to do everything that really possibly can't do. And it's not even. We haven't even talked about artificial general intelligence. But it's not there. But people are starting to believe maybe it is. That's a whole different subject. AGI
Christopher Nguyen 35:23
will mark my word is a very simple mental model, which were largely which model is set up is just the first half. You did the other half.
Justin Grammens 35:31
Great. Yeah. That's a great point that you've made. And that's been sort of the foundation of our conversation today is you have to have that industry knowledge. So well. Cool, Christopher, I appreciate the time. Thank you so much for sharing with us your experience and good luck on your company. It sounds like this is probably one of many, many companies. You found it so excited to see what happens with it with your career in the future. I'm sure this is not your last, buddy. But good luck. And yeah, we have liner notes. So we'll have a link to your website and the link to the careers page. Link to the conference. The knowledge first World Cup of Princeton. Yeah, thank you so much for being on the program. Appreciate it.
Christopher Nguyen 36:04
Also. Thanks again.
AI Announcer 36:07
You've listened to another episode of the conversations on applied AI podcast. We hope you are eager to learn more about applying artificial intelligence and deep learning within your organization. You can visit us at applied ai.mn To keep up to date on our events and connect with our amazing community. Please don't hesitate to reach out to Justin at applied ai.mn If you are interested in participating in a future episode. Thank you for listening